Hidden Insights
Experience the possibilities with Business Analytics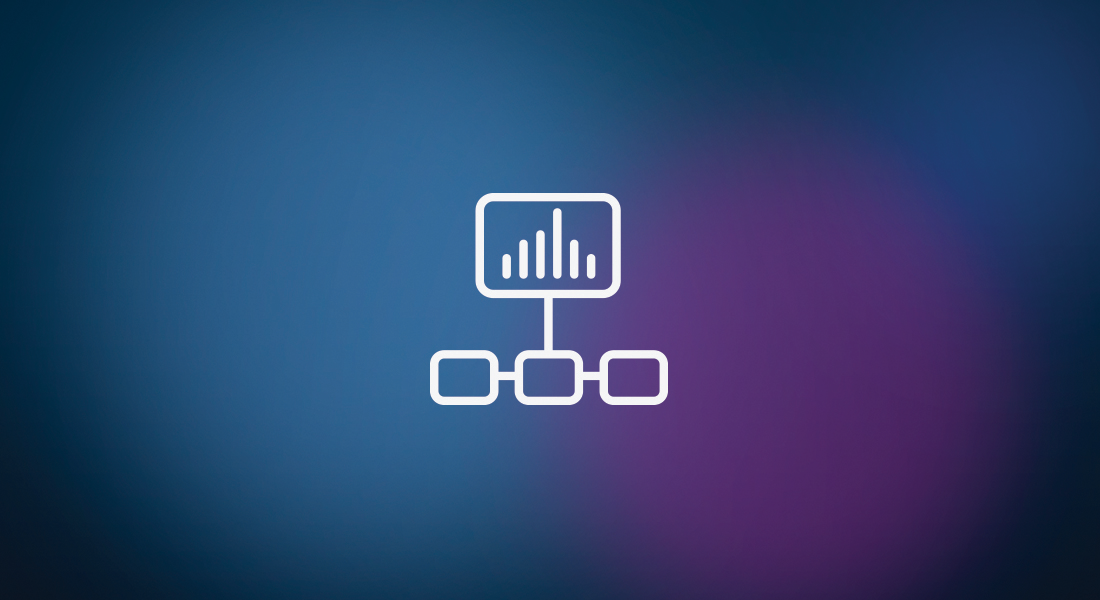
Adopting analytics and AI in the public sector is changing how governments make decisions. Technology improves the efficiency and transparency of work processes, positively impacting service delivery to increasingly demanding citizens. However, many government organizations still need help fully embracing a data-driven culture. The Covid-19 pandemic is one of the
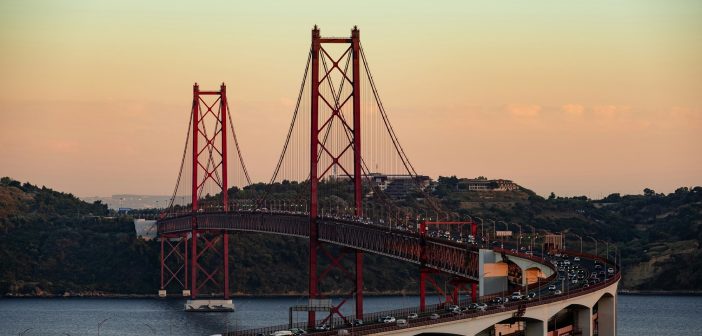
Estamos quase na Semana Santa o que, por ser uma das épocas em que mais viagens se realizam por todo o país, pode apresentar vários desafios à rede rodoviária e aos sistemas de transporte. A variabilidade nos padrões de movimento durante estas datas acrescenta uma camada adicional de complexidade, o
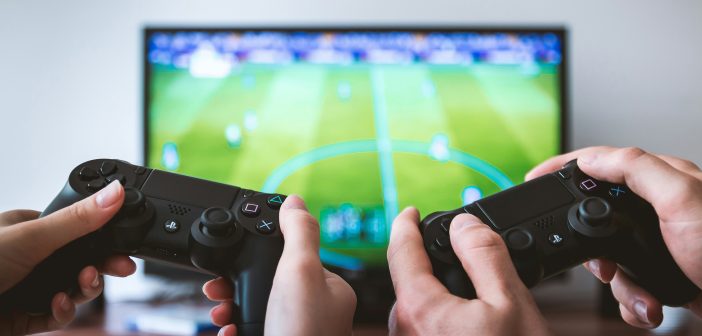
In the ever-evolving landscape of media, understanding how different generations consume content is crucial. Deloitte's report on Digital Media Trends 2023 sheds light on these generational disparities, highlighting a clear shift towards online experiences among younger audiences. This trend presents both challenges and opportunities for businesses, as they grapple with how
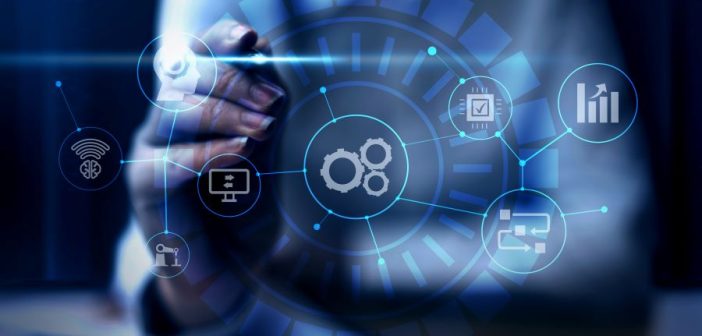
Es una realidad que la IA es una herramienta muy valiosa para combatir el fraude, ya que su capacidad para analizar datos y detectar patrones sospechosos supera en muchos casos la capacidad humana. Sin embargo, el uso de modelos de Machine Learning en nuestros sistemas de detección de fraude puede
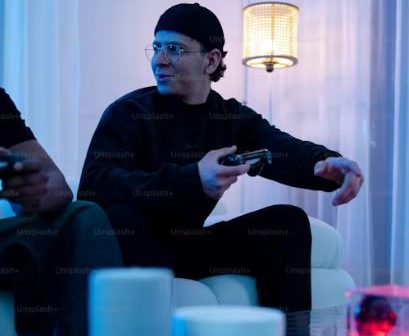
¿Cómo pueden las marcas mantenerse al tanto de lo que están haciendo sus clientes? Informes como el ‘Deloitte’s Digital Media Trends 2023’ son cruciales. En él se pone de manifiesto las diferencias que hay entre generaciones, además de ofrecer una serie de consejos para medios de comunicación y marcas. Estas
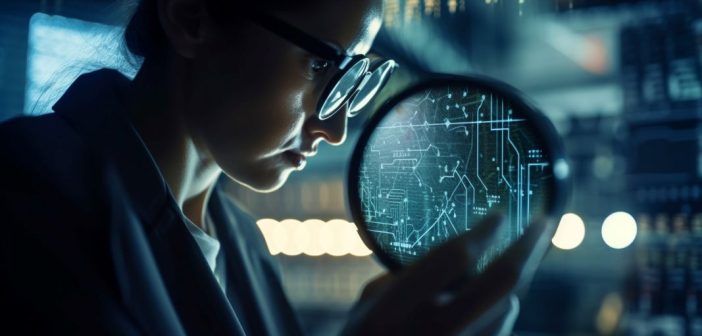
La inteligencia artificial se perfila como un aliado de gran valor en la lucha contra el crimen financiero. Su capacidad para detectar de forma temprana posibles actividades sospechosas, analizar grandes cantidades de datos en tiempo real o automatizar la detección de fraudes, la convierte en una herramienta indispensable para las