Get the latest machine learning algorithms and techniques
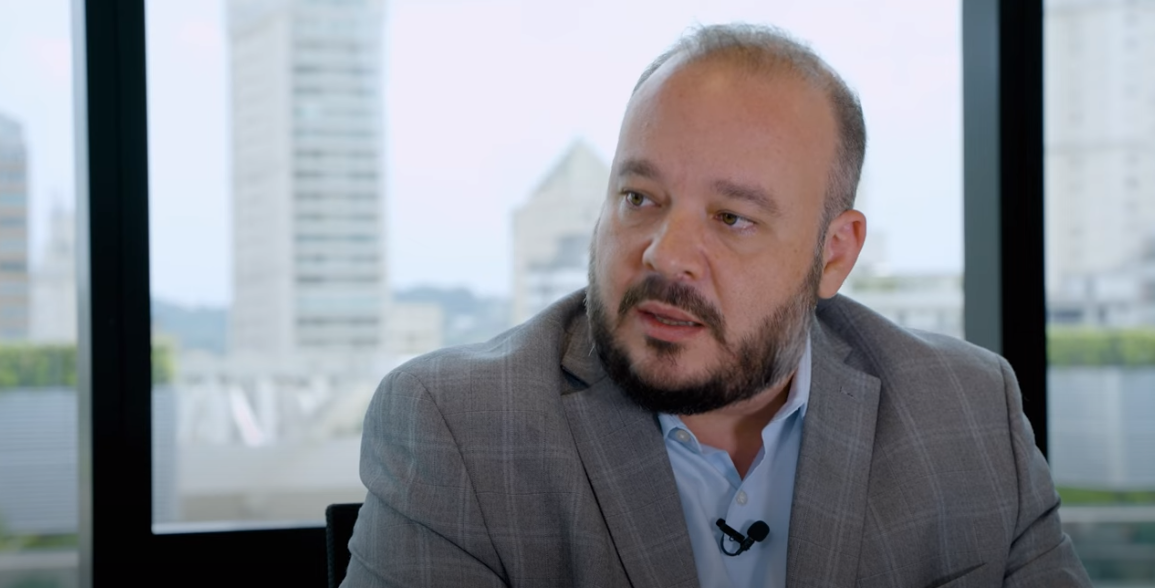
A relação entre a prevenção de fraudes e a experiência do cliente é indiscutivelmente estreita no mundo financeiro moderno. A análise de tendências atuais revela a importância da preservação dos dados como um fator crucial para organizações, impactando a confiança que clientes tem em empresas, a retenção deles - e,