Get the latest machine learning algorithms and techniques
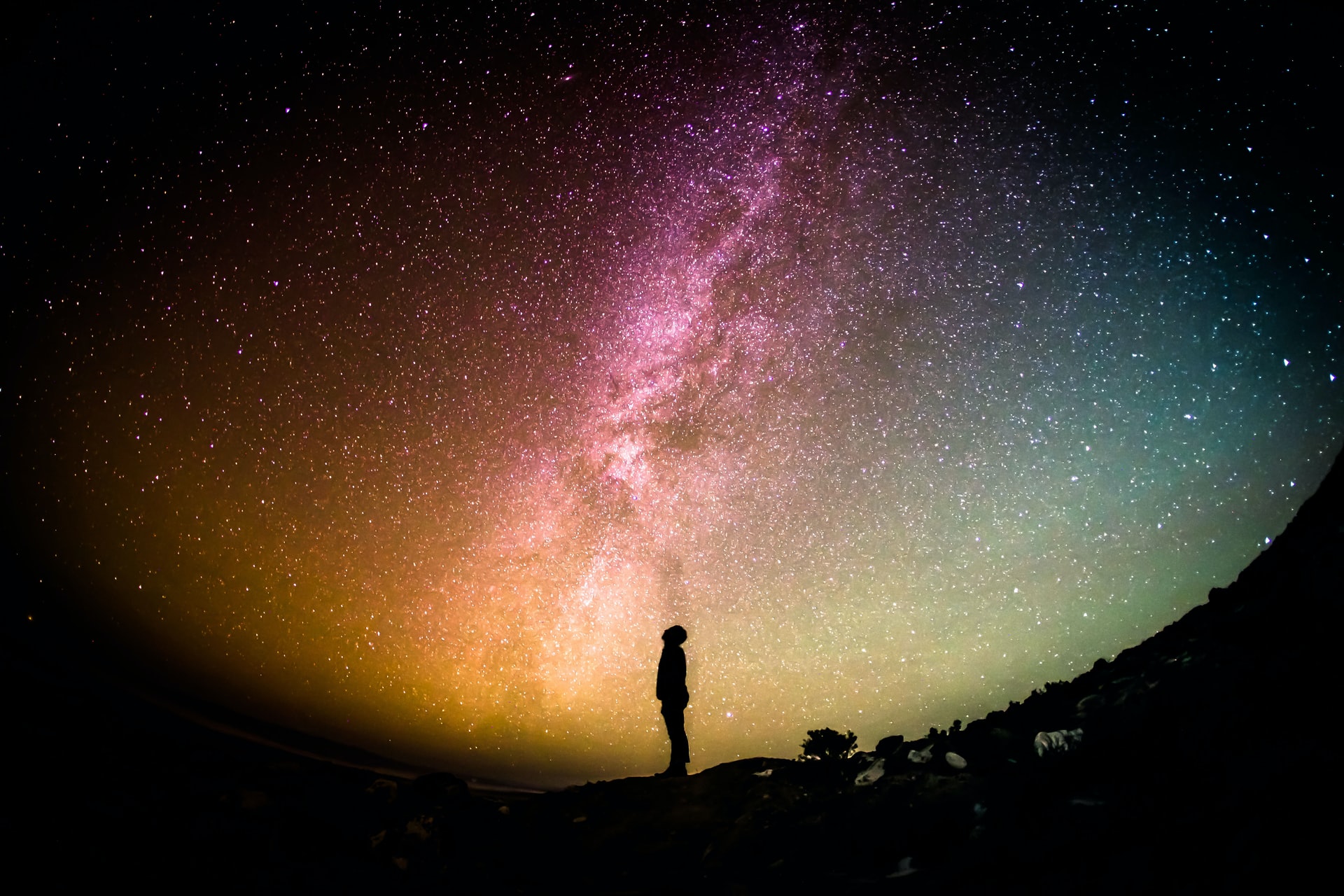
This year we had the pleasure to attend the 42nd International Symposium on Forecasting in Oxford, UK. SAS participants traveled from across the globe. They presented their research, discussed their ideas, and learnt from some of the most reputable names in the forecasting space. The presentations were captivating and the