This year we had the pleasure to attend the 42nd International Symposium on Forecasting in Oxford, UK. SAS participants traveled from across the globe. They presented their research, discussed their ideas, and learnt from some of the most reputable names in the forecasting space.
The presentations were captivating and the ongoing research that is taking place in forecasting got us very excited. Machine learning and deep learning techniques are becoming mainstream in the field of forecasting. However, there is still much research around the more traditional statistical ways. These are still well-utilized due to their robustness, their ability to achieve fast and accurate results; and their easiness of interpretation compared to the more complex ML-based methods.
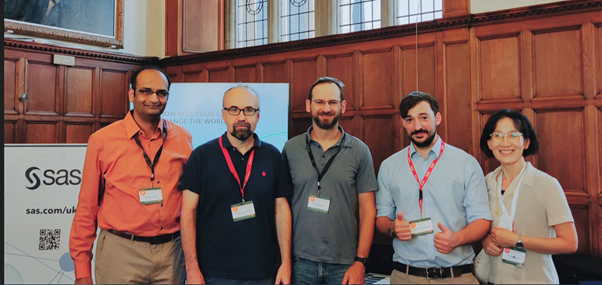
So, what did we learn at ISF this year? Undoubtedly, it’s way too much to discuss in a single article. Still, the presentations will be available on IIF’s YouTube channel. So please keep an eye on that if you want to learn more about a particular topic. The learnings that stood out for us were the following:
1. Both ML and traditional statistical methods are now a part of the required toolkit of a forecaster
Machine learning methods have proven their worth in the latest forecasting competitions. While more and more companies have started quickly adopting them in their regular forecasting activities. There is strong evidence that there are many cases when they fail to outperform robust statistical methods. A debate between the two ways is equivalent to debating whether a fork or a knife is better to eat your dinner. ML techniques require special attention in data preparation and work best when dealing with complex patterns and a large volume of interrelated series. In the M5 forecasting competition, 92.5% of the participating teams failed to outperform the benchmark, which was based on exponential smoothing models.
2. Clustering before forecasting can enhance forecasting results
The problem arises when we try to decide which clustering method to use. Many presentations focused on which clustering method could lead to the most accurate forecasting results when models are applied (post-clustering) separately to each group. Youngjin Park, a principal researcher from the SAS R&D division, showcased some essential clustering methods. Including similarity-based, component and k-means, which can all be implemented using SAS procedure as well as an attribute clustering method that comes out of the box with SAS Visual Forecasting. It classifies time-series data based on historical demand patterns. Her analysis showed that attributes-based clustering could enhance forecasting accuracy, while similarity-based clustering is the most suitable among the first three methods and can be combined with the attributes-based segments to improve the quality of forecasting models further.
3. Are we using the right measure to assess models?
That’s a theme that has been going on since the inception of forecasting. This year a new criterion for forecast selection was presented by prof. Fotios Petropoulos and prof. Enno Siemsen, that tries to quantify the representativeness of the forecasts. Until now, we didn’t have a criterion to tell us if the forecasts made sense, and we had to rely solely on human judgment to make this decision. The new standard, called REP, aims to fill that gap by putting more emphasis on recent time windows and rejecting forecasts that look unrealistic. Using all data from the M1-M4 competitions, the paper’s authors proved that selecting forecasts by REP generally leads to better forecasts for most of the data frequencies tested. SAS is looking to incorporate this new criterion in SAS Visual Forecasting to allow practitioners to use it in their forecasting processes.
Overall, we learned loads and can’t wait for next year’s conference in Charlottesville, Virginia, USA, June 25-28 2023!
Please click HERE to access the SAS Viya Free trial and HERE for SAS Academics Programs.