As promised in this latest blog about the Gartner Data & Analytics Summit in London, here’s an update from the second day at the SAS booth.
To make a long story short, each day the SAS booth team posed a question to attendees visiting the booth. They could submit three votes to be selected from five possible answers, registered by a column of coloured balls (one for each voting option).
On day #2 the question raised was: “What is the best strategy (in order) to scale analytics?” with the following response options:
- Democratize with low or no-code.
- ModelOps (automation and governance).
- Centre of excellence.
- Cloud adoption.
- Training and data literacy.
As you can see below, visitors voted most for “Training and data literacy” – or perhaps there was a simple preference for the colour purple. Of course, training is important but I think voters underestimate the impact of a centre of excellence (COE), which would have received my first vote, and let me explain why.
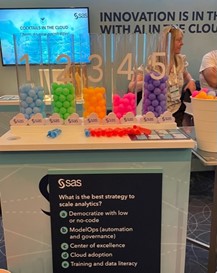
Centre of excellence
In most of the customer organizations I’ve worked with over the years that have been successful with analytics, a common factor has been the presence of a COE function to drive and evangelise the role of analytics. Now this catalyst may not necessarily exist in the guise of a formal analytics COE (with headcount and reporting lines) and it could be as modest as an individual leader who has been able to galvanize and promote the take-up of analytics. A common factor is the ability to lead and influence.
Visitors to our booth were equally divided between those organizations with an analytics COE, and those who had yet to implement one.
Of course, it’s tough to implement a data-driven culture unless your consumers are data literate and have access to up-to-date training. And you could argue this topic should be owned & driven by the analytics COE.
University training and education
We witnessed a very animated discussion on this topic between booth visitors where one argued that training and data literacy should be educated at schools and universities and would no longer be required as a strategy to scale analytics. Certainly, there is a clear evolution (in skills) going on between recent graduates versus older employees.
We’re now finding graduates are emerging from universities with knowledge of Python (rather than the SAS language). It is tempting for customer organizations with an existing SAS environment to on-board new team members and simply let them “learn on the job.” However, the risk is that individuals without training will simply build and extend by replicating existing SAS code. While this is effective, it may not be efficient or optimal as the SAS platform has evolved massively over the past 40+ years.
Here are some examples of what SAS Viya can offer today:
- A GUI-based interface for data preparation, visualization, advanced analytics, ML & AI, which allows citizen data scientists to become rapidly effective without needing to code. This delivers a ”low or no-code” capability to improve analyst consumer productivity.
- At the same time, for data analysts who prefer to code, there’s a programming interface providing access to identical capabilities.
- SAS Viya embraces open-source tooling for high-end analytics but also everyday tasks. For example, you can create data acquisition flows using mix of SAS and Python code.
- SAS Studio is a web-based code interface that replaces old favourites such as SAS Enterprise Guide and PC SAS Display Manager, but alternatively you could use a Jupyter notebook or Microsoft VS Code.
But I’m drifting away, so let’s return to the topic of voting.
ModelOps
It’s curious to see that ModelOps came a close second in the voting as many of the visitors of our booth confessed that their organizations were facing more basic challenges related to data governance and data quality.
ModelOps addresses the ”last mile” problem that exists in many industries including supply chain and telecommunications. Simply put, organizations are challenged to convert analytics into effective decision making. This reflects the fact that it’s becoming more difficult to deploy analytical models (created by data science teams) into production contexts.
ModelOps is a relatively new discipline which treats models as assets and introduces governance and a lifecycle-based methodology for model development, deployment and management. You can read more about how SAS operationalizes analytics here.
Cloud adoption
The topic of the cloud was reduced by most to merely the infrastructure of the public cloud, while there is so much more to it. The introduction of a cloud-native architecture, continuous integration and continuous deployment (CI/CD), a more flexible and agile way of working that these architectures enable, provides a broader view. This topic might have been more popular if presented as such.
So what did we learn from day two? It’s the power of purple, and despite my reservations, I reconcile myself to the wisdom of the crowd. Stay tuned for the final episode of day three, where we will discuss the hurdles and challenges on the bumpy road of the data-driven organisation.