SAS Voices
News and views from the people who make SAS a great place to work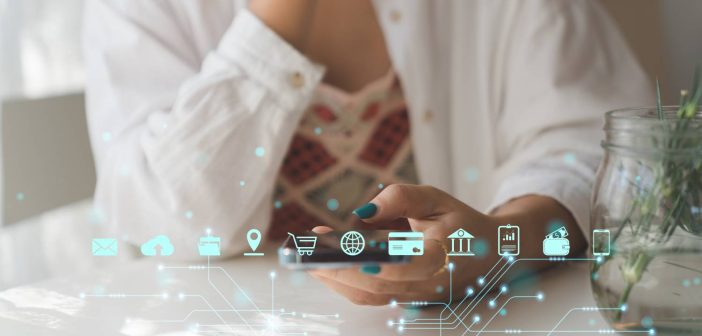
Digital banking, big tech and data privacy are all contributing to a shift in the Scandinavian banking scene, according to Tom Palving, Center of Excellence Lead for Digital Communication and Sales Loyalty at Jyske Bank. As a result, says Palving, traditional banks are facing a number of challenges, including: Customers
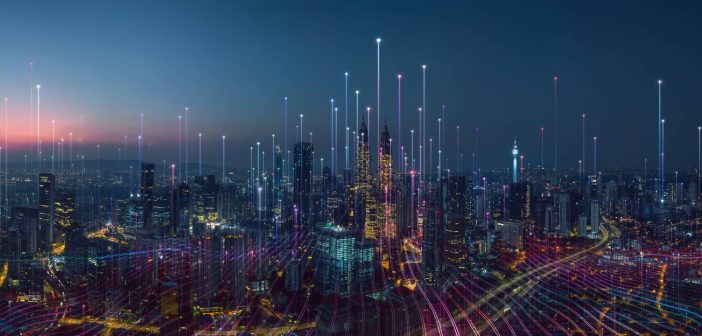
Urbanization is rapidly accelerating, allowing communities to grow with more access to services and technological advancements. However, this quick change in growth can present challenges as well, such as pollution and lack of infrastructure. Smart cities can support urbanization by offering innovative solutions with artificial intelligence (AI), the Internet of
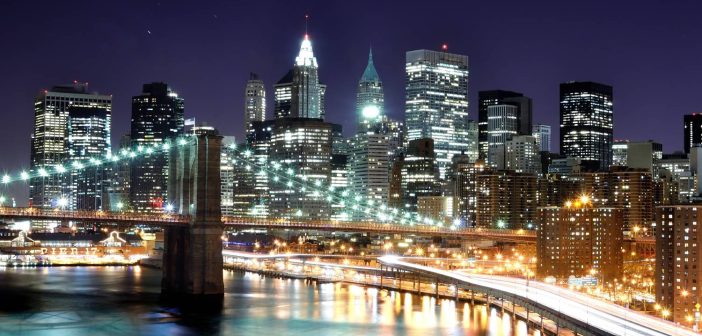
Thanks to the ubiquity of smartphones and laptops, people are used to getting what they need at the press of a button – whether they’re looking for information, seeking action or trying to solve a problem. Citizens want that same ease of access from their city’s government. As more citizens
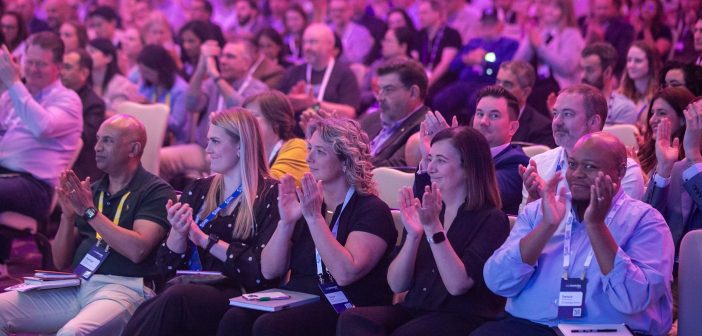
Bright ideas came to light as we closed out SAS Innovate 2024. Day three opened on a high note with a fun performance from iLuminate. After that, we jumped into learning about our partners’ work and SAS innovations. How partners are helping others SAS Vice President of Global Channels John Carey
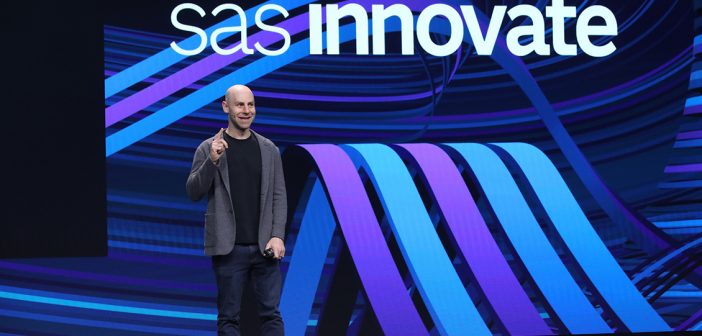
Original thinker, effective teacher, advocate for underdogs: These are just a few of the ways SAS Innovate featured speaker Adam Grant has been described. Add to that corporate culture expert and #1 New York Times bestselling author – most recently for Hidden Potential: The Science of Achieving Greater Things. SAS
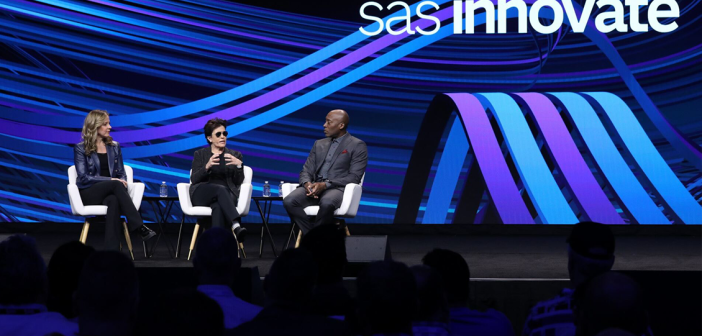
Have you ever heard the saying, “Products that don’t perform won’t be trusted, and products that can’t be trusted aren’t worth the machines they’re coded on”? Maybe not, because I just made it up. But at SAS, as we continue to create products that perform and change the world, we