Customer Intelligence Blog
Evolving relationships for business growth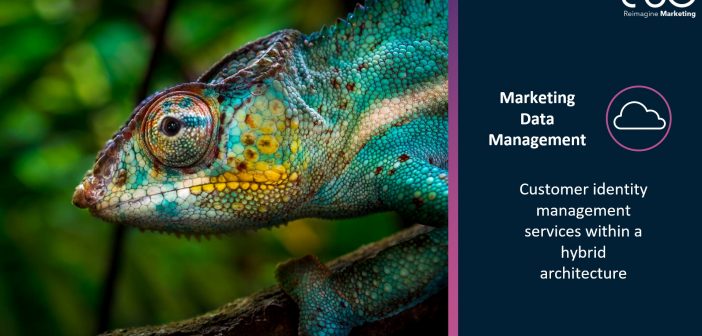
Customer data platforms (CDPs), data management platforms (DMPs), people-based marketing, identity graphs, and more overlapping topics represent an important ingredient of any martech brainstorming session in 2020. As your brand spreads out across touchpoints — from web to mobile applications, as well as call centers, email and direct mail —
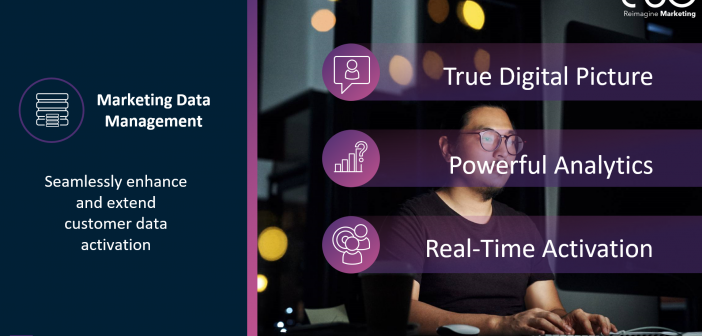
No matter what your brand's level of marketing maturity is, SAS can help you move from data to insight to action with rich functionality for adaptive planning, journey activation and an embedded real-time decision engine – all fueled by powerful analytics and artificial intelligence (AI) capabilities. Let's begin with a
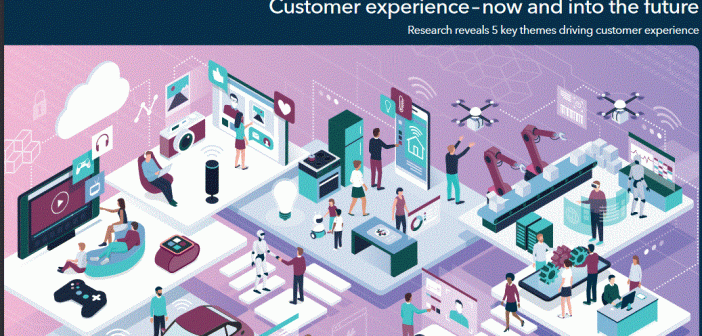
In our fast changing, increasingly digital world, building a strong customer relationship is the lynchpin to building a great business. The stakes are even higher now as global disruptions occur with alarming regularity—be it political unrest, economic meltdowns or a pandemic. A new, global study called Experience 2030: The Future
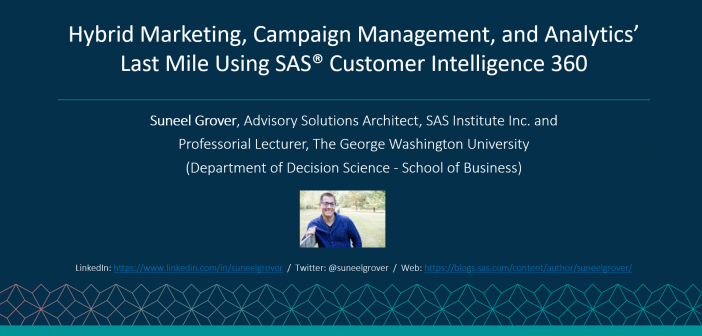
After careful consideration of the evolving COVID-19 situation, SAS made the decision in March to cancel the in-person SAS Global Forum 2020 conference in Washington, DC. The health and well-being of SAS customers and employees was the company's top priority in making that decision, and while it's unfortunate that we
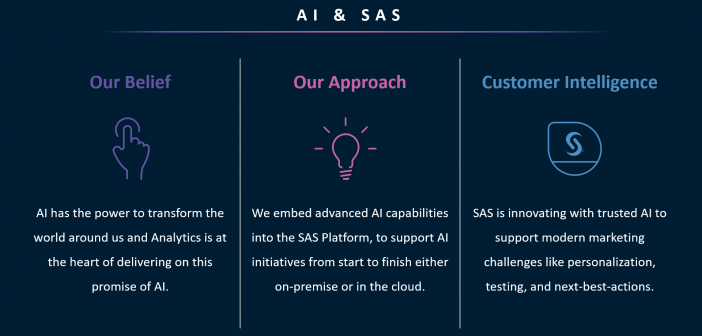
In February, SAS was recognized as a Leader in the 2020 Gartner Magic Quadrant for Data Science & Machine Learning Platforms report. SAS is the only vendor to be a leader in this report for all seven years of its existence. According to us, the topic of the research is
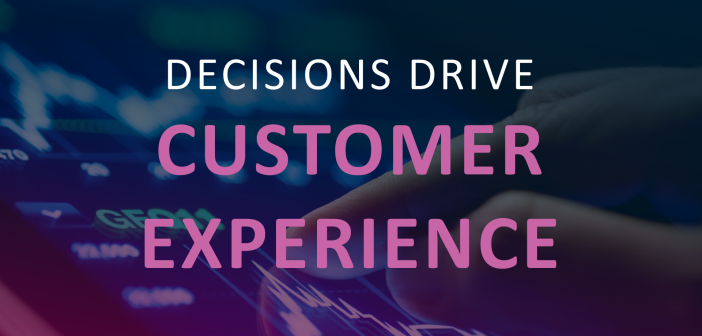
Excitement levels are high for the March 2020 release of SAS Customer Intelligence 360, which includes multiple years of research and development culminating in enhancements to the platform's underlying data model. The changes will introduce the unification of a comprehensive data model recording both: Customer behavior -- what users are