Customer Intelligence Blog
Evolving relationships for business growth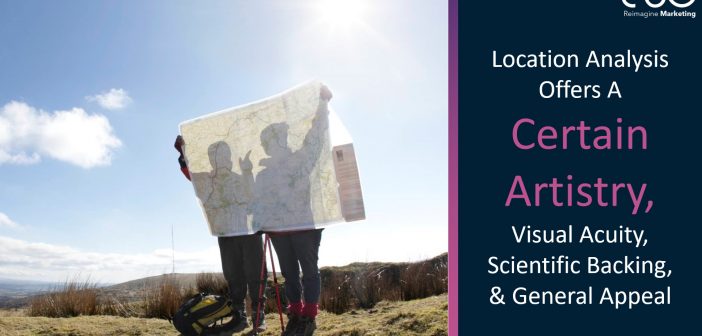
Everything happens somewhere, and much of our customer data includes location information. Websites include x, y coordinates in semi-structured click streams, and the mobile apps your prospects depend on frequently support device location to provide a personalized, targeted experience. As my SAS peer Robby Powell said: "Human brains are hardwired
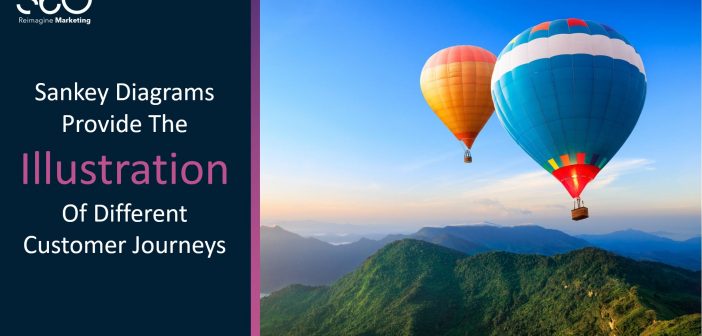
We live in the age of data. From global warming stats to customer behavior patterns, new technologies have made it easier to collect, store, access and analyze information. But our use of these technologies has also eroded our attention spans and fueled post-truth misunderstandings. To combat these trends, the question
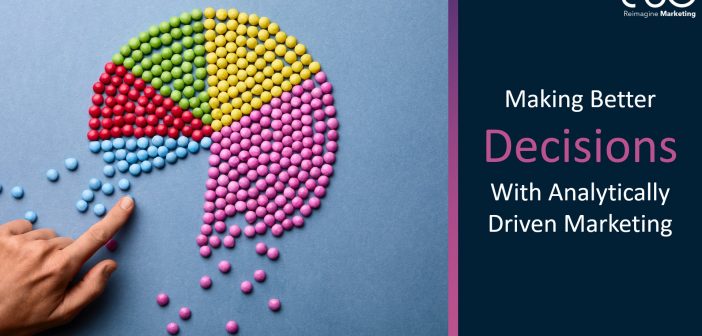
According to the SAS Experience 2030 global study, by the year 2030 67% of in-person customer engagements (think sales assistance and information queries) will be completed by smart machines rather than humans. And while it may seem a bit ironic, the most personalized customer experiences could involve no people at
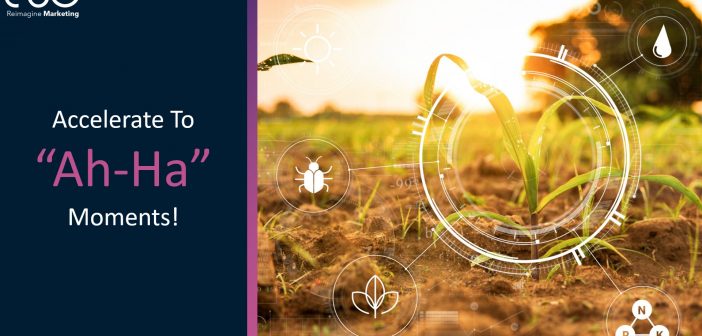
One of the wonderful aspects about my client-facing role at SAS is the breadth of audiences that I get to work with. No matter where you fall on this list: Data engineer. Business or marketing analyst. Citizen data scientist. Data scientist. Statistician. Executive. One topic is certain: We all love
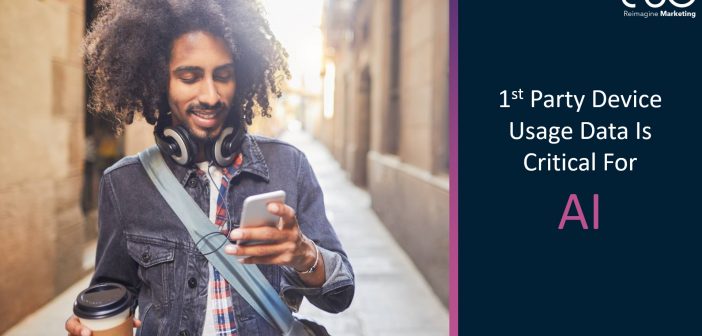
Over the last 20+ years, global society has adopted digital devices at scale, and consumer interaction behaviors continually evolve and mature. As analysts, we're uniquely positioned to notice worldwide trends, country-specific nuances and localized market behaviors that can have significant impact on our brand's business goals. This global scope is
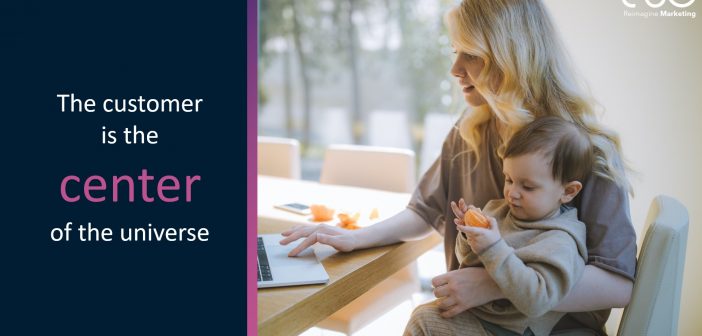
There's no question that we're all increasingly, and often exclusively, interacting with brands digitally. Consumers are now online through countless mechanisms – from laptops and mobile apps to AI-enabled voice assistants and sensor-based wearables. Engagement is diversifying in fascinating new ways. And when organizations can't see their customers interacting in