The SAS Data Science Blog
Advanced analytics from SAS data scientists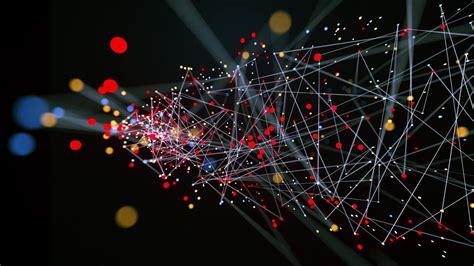
Editor's note: This article follows Curious about ChatGPT: Exploring the origins of generative AI and natural language processing. As ChatGPT has entered the scene, many fears and uncertainties have been expressed by those working in education at all levels. Educators worry about cheating and rightly so. ChatGPT can do everything
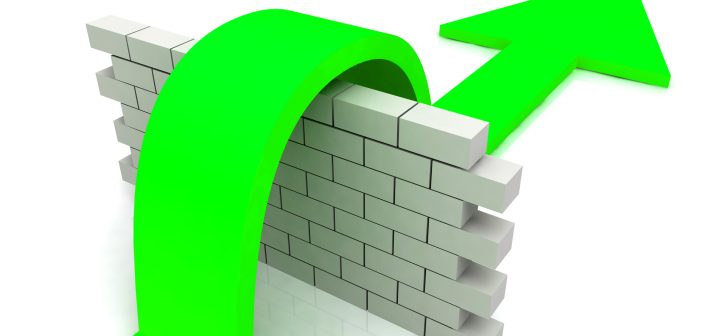
SAS' Mary Carter details challenges and benefits of accelerating the delivery of SAS software.
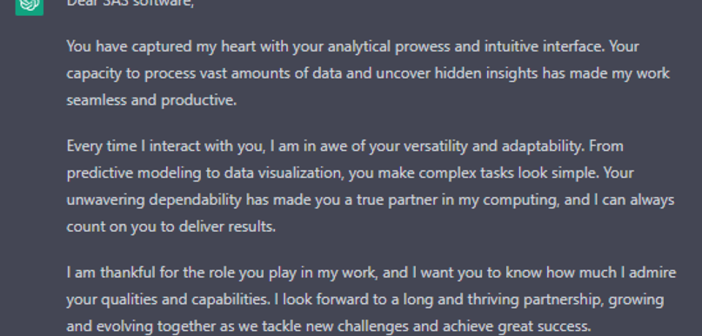
How did we get to a place where a conversational chatbot can quickly create a personalized letter? Join us as we explore some of the key innovations over the past 50 years that help inform us about how to respond and what the future might hold.
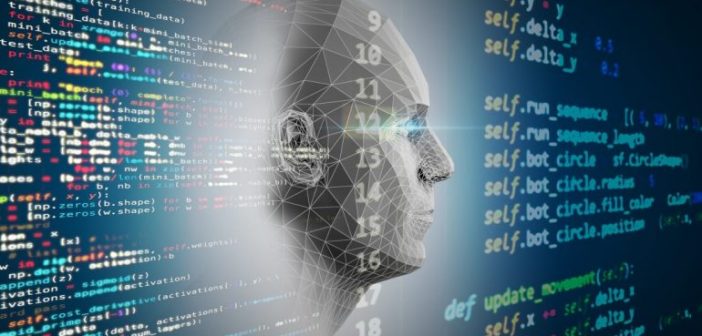
NeurIPS 2022 allowed researchers and practitioners to share progress and brainstorm new ideas for advancing machine learning and its related fields.
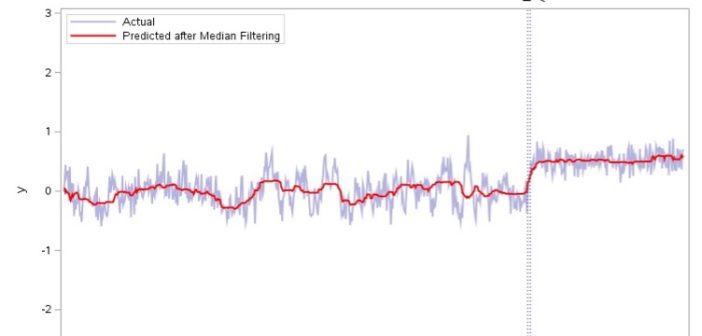
Time series data is widely used in various fields, such as finance, economics, and engineering. One of the key challenges when working with time series data is detecting level shifts. A level shift occurs when the time series’ mean and/or variance changes abruptly. These shifts can significantly impact the analysis and forecasting of the time series and must be detected and handled properly.
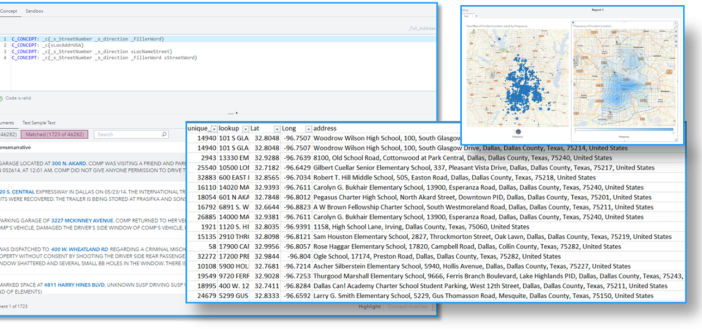
SAS' Kirk Swilley and Tom Sabo showcase how you can use perform text analysis on minimal structured narrative data to spot patterns of possible human trafficking.