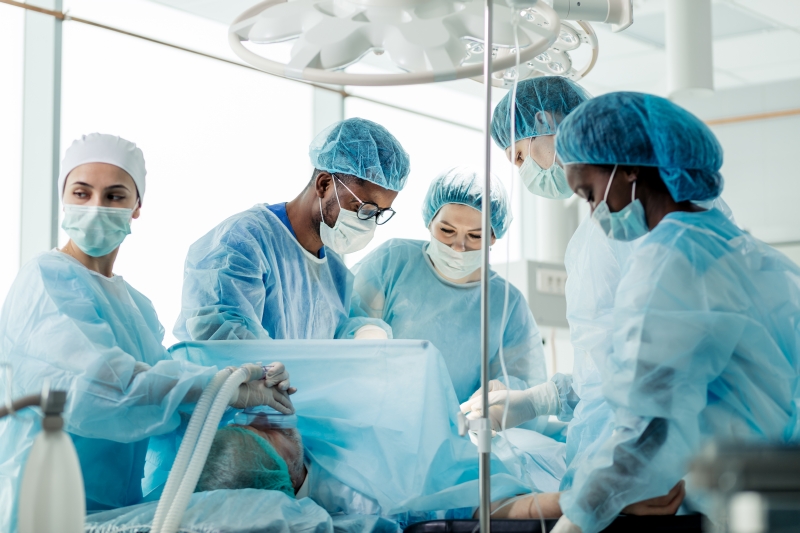
Cada vez mais as ferramentas de IA apoiam a tomada de decisão e ajudam na criação modelos que identificam tendências e padrões de comportamentos que, juntamente com regras de negócios, permitem que as empresas tomem decisões mais assertivas, seja qual for sua área de atuação. As análises mais avançadas incluem