The SAS Data Science Blog
Advanced analytics from SAS data scientists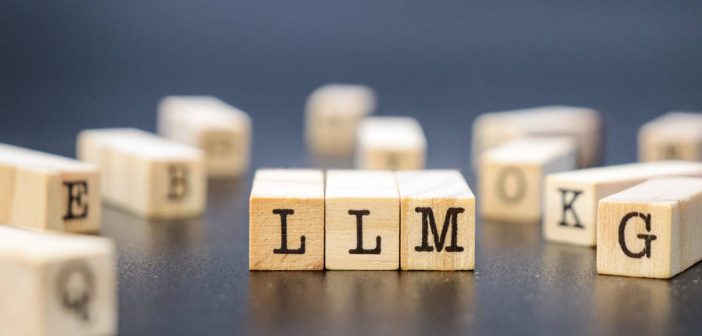
Adding linguistic techniques in SAS NLP with LLMs not only help address quality issues in text data, but since they can incorporate subject matter expertise, they give organizations a tremendous amount of control over their corpora.
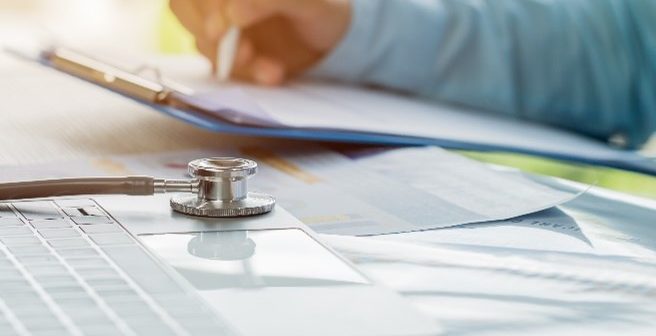
SAS' Greg Massey describes a real-world example of digital transformation for a large customer grappling with manually reviewing patient medical records.
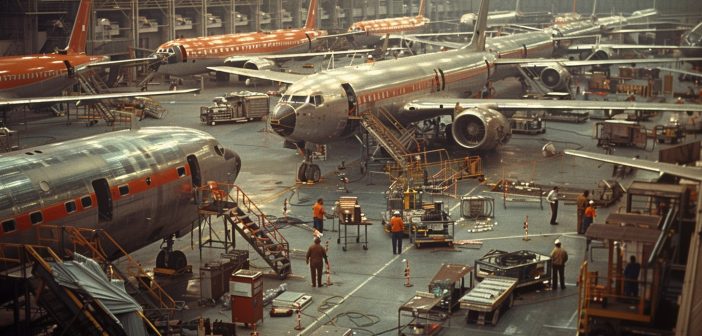
SAS' Bahar Biller guides you through an asset lifetime prediction scenario using a synthetically generated historical data set and a solution built on SAS reliability modeling.
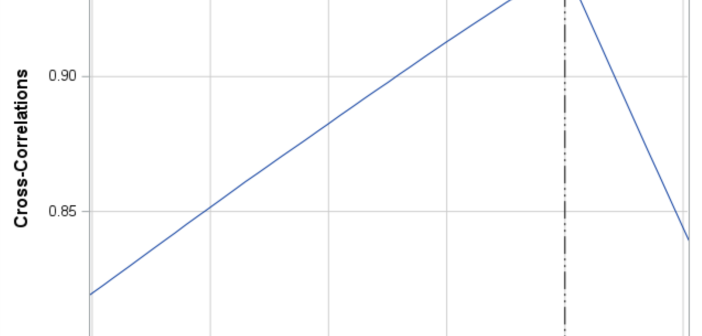
Batch manufacturing involves producing goods in batches rather than in a continuous stream. This approach is common in industries such as pharmaceuticals, chemicals, and materials processing, where precise control over the production process is essential to ensure product quality and consistency. One critical aspect of batch manufacturing is the need to manage and understand inherent time delays that occur at various stages of the process.
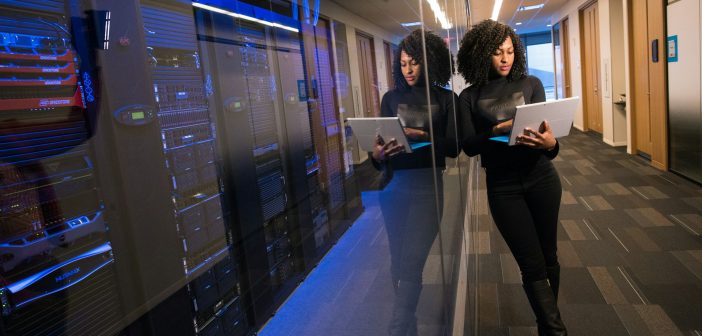
SAS' Federica Citterio answers the perennial data science question: "How can I trust (generative) LLM to provide a reliable, non-hallucinated result?"
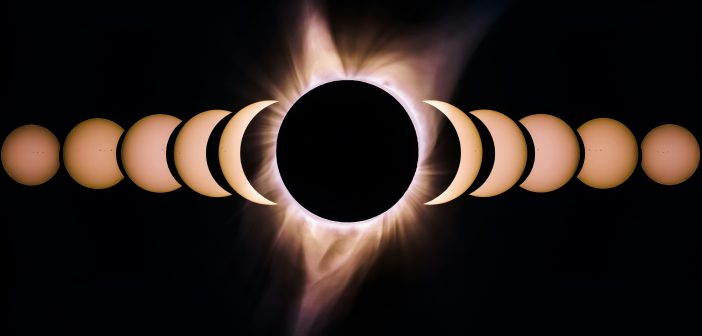
Authors: Steven Harenberg and Amy Becker The total solar eclipse taking place across a thin band of the United States on April 8, 2024, is going to be a stellar event. In this post, we will help plan a journey to see the total solar eclipse. We will use algorithms