The SAS Data Science Blog
Advanced analytics from SAS data scientists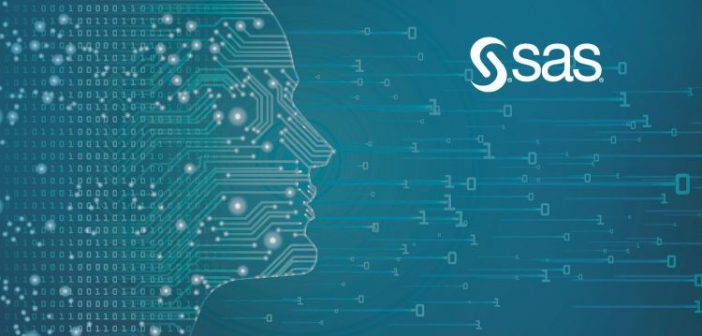
If you are an Enterprise Miner user, do not miss the opportunity to try out Model Studio in SAS Viya. I am sure you will love it!
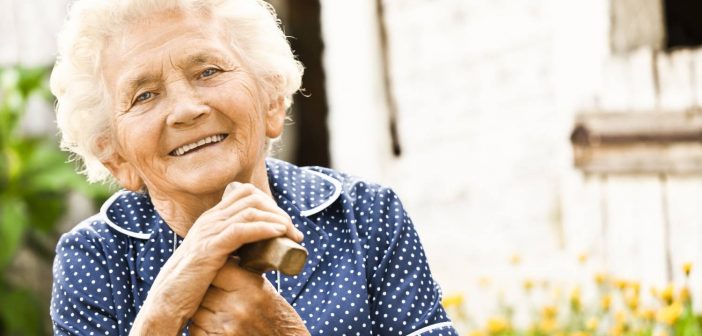
The application of IoT devices and artificial intelligence in healthcare presents numerous opportunities in use cases like training, research, end-of-life care, treatment, keeping well, early detection, diagnosis, and decision-making.
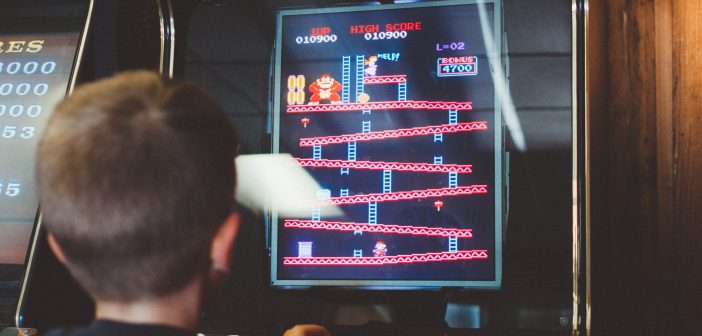
With modern advancements in artificial intelligence, we can teach computers to achieve super-human performance in retro videogames.
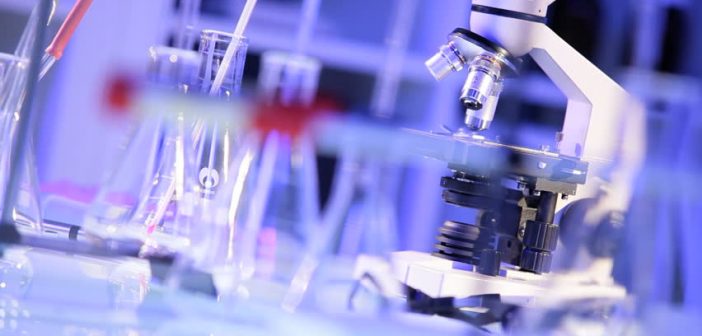
SAS' Courtney Ambrozic highlights how to use SAS VDMML to assess lesion response to chemotherapy for patients with colorectal liver cancer.
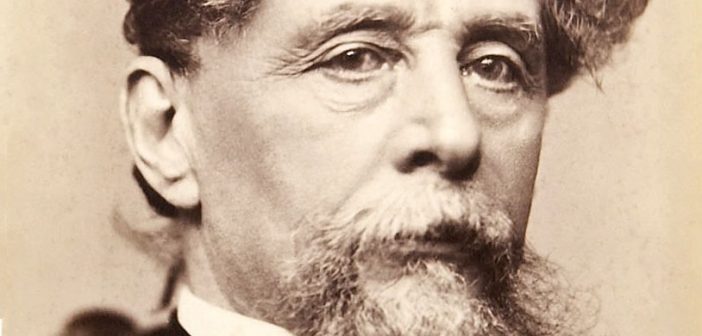
Corpus analysis is a technique widely used by data scientists because it provides an understanding of a document collection and provides insights into the text.
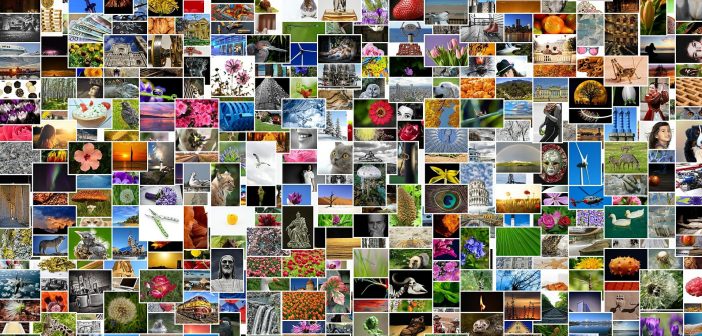
SAS' Pankaj Telang shows you new image-specific processing capabilities in SAS Visual Data Mining and Machine Learning.