Customer Intelligence Blog
Evolving relationships for business growth![SAS Customer Intelligence 360: A look inside the black box of machine learning [Part 3]](https://blogs.sas.com/content/customeranalytics/files/2018/11/interpretability-702x336.jpg)
In parts one and two of this blog posting series, we introduced machine learning models and the complexity that comes along with their extraordinary predictive abilities. Following this, we defined interpretability within machine learning, made the case for why we need it, and where it applies. In part three of
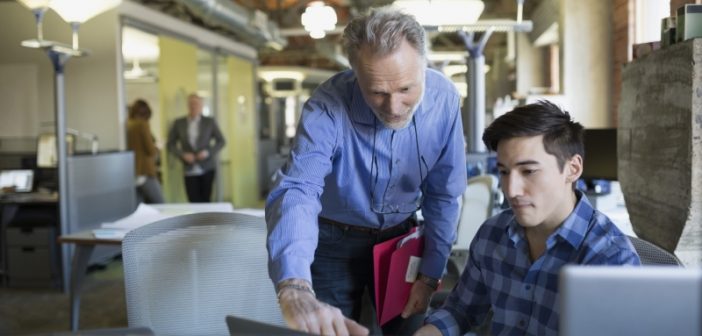
How different is different? Descriptive analytics is powerful. You get to see your data and seeing is believing as they say. But blindly believing what you see is not always the best strategy. Asking someone else if they see what you see helps but is still subjective. Objectivity starts with
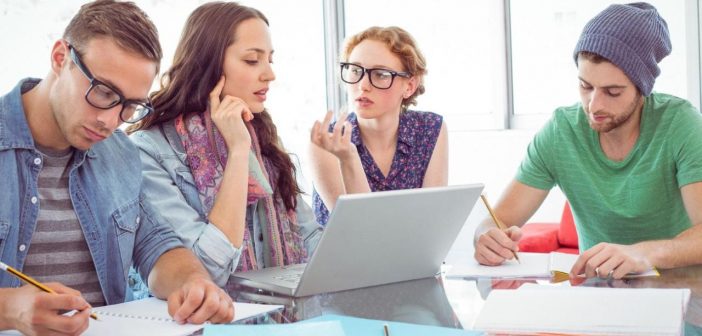
The only constant is change – Heraclitus As online user behavior continues to evolve, user expectations are growing as well. More and more, users expect to be known; thus, web personalization and targeted content are becoming mission critical and an expectation, not an exception. Using SAS Customer Intelligence 360, we
![SAS Customer Intelligence 360: A look inside the black box of machine learning [Part 2]](https://blogs.sas.com/content/customeranalytics/files/2018/11/machine-learning-2-702x336.jpg)
In part one of this blog posting series, we introduced machine learning models as a multifaceted and evolving topic. The complexity that gives extraordinary predictive abilities also makes these models challenging to understand. They generally don’t provide a clear explanation, and brands experimenting with machine learning are questioning whether they
![SAS Customer Intelligence 360: A look inside the black box of machine learning [Part 1]](https://blogs.sas.com/content/customeranalytics/files/2018/11/machine-learning-702x336.jpg)
As machine learning takes its place in numerous advances within the marketing ecosystem, the interpretability of these modernized algorithmic approaches grows in importance. According to my SAS peer Ilknur Kaynar Kabul: We are surrounded with applications powered by machine learning, and we’re personally affected by the decisions made by machines
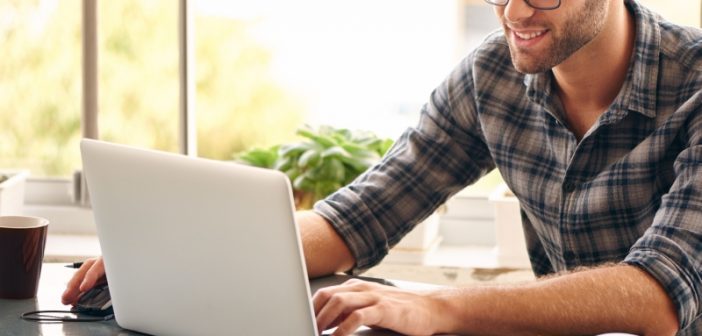
In an earlier blog post my colleague, Suneel Grover, wrote about how to take advantage of the product recommendations technology implemented in SAS Customer Intelligence 360. He noted that two methods are implemented behind the scenes, a visitor-centric and a product (or item)-centric approach. And most importantly, while some sophisticated