Find out how analytics, from data mining to cognitive computing, is changing the way we do business
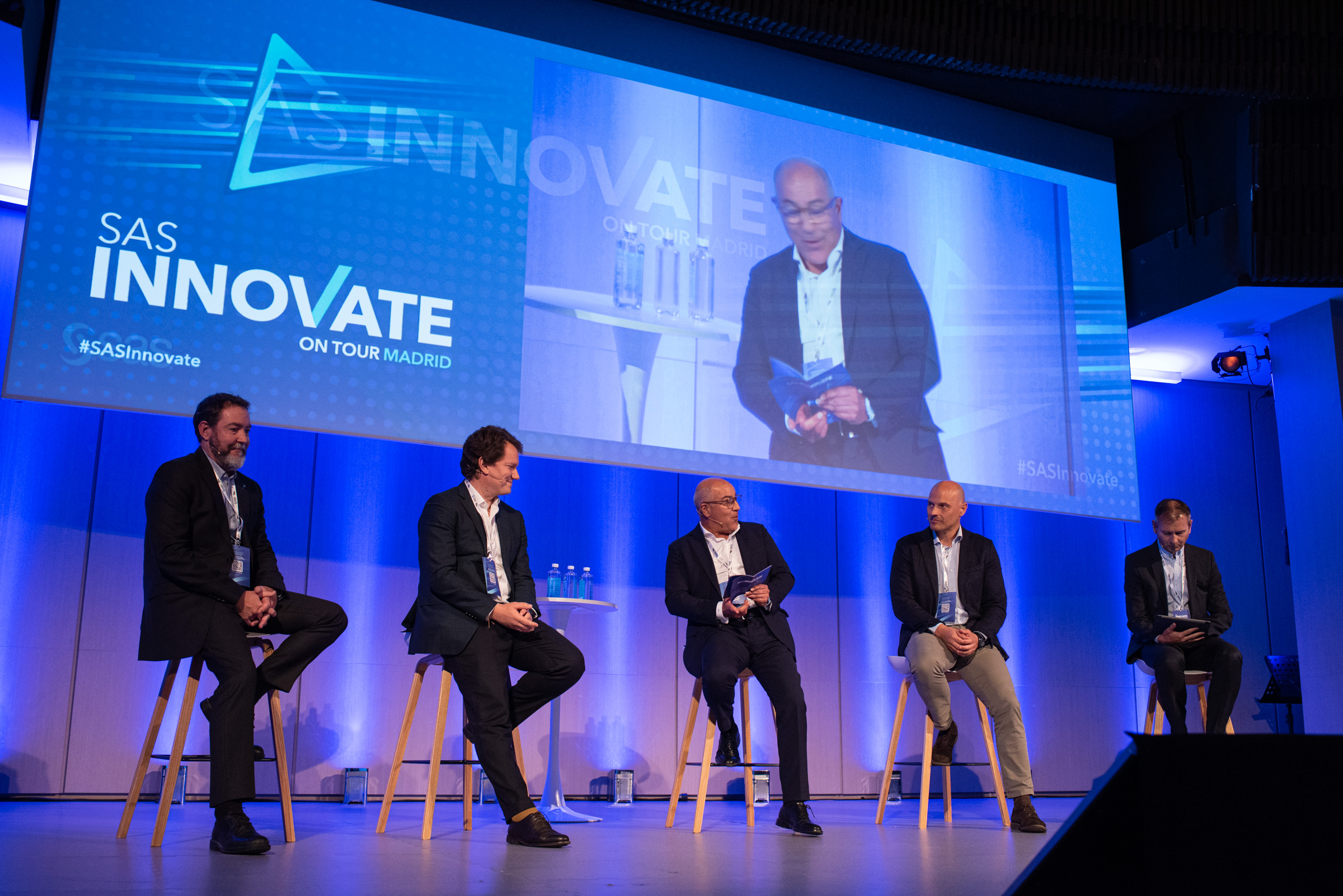
Ao longo da última década, a inteligência artificial evoluiu para responder à necessidade de gerir grandes volumes de dados, os quais aumentam constantemente, tendo-se tornado uma ferramenta essencial para as empresas. De facto, de acordo com o estudo do SAS Resiliency Rules, 92% dos diretores portugueses acreditam que a IA