The SAS Data Science Blog
Advanced analytics from SAS data scientists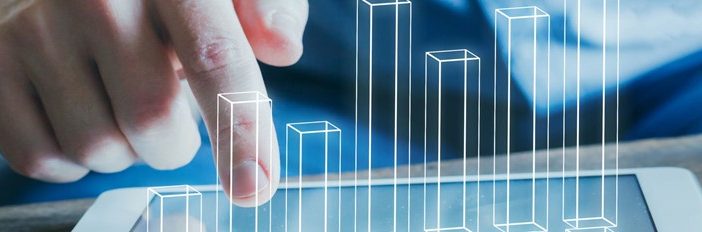
Billy Dickerson of SAS R&D chronicles three key challenges and lessons learned in SAS' journey to continuous integration (CI) and continuous delivery (CD).
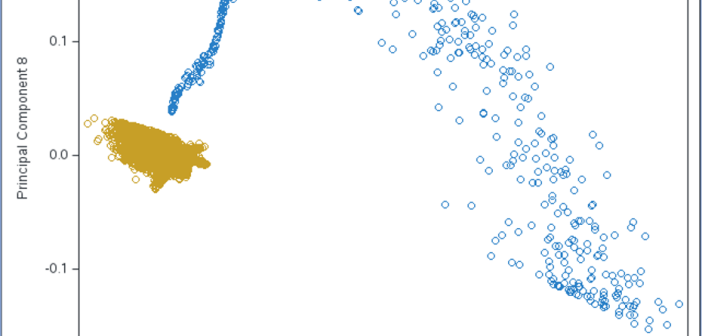
SAS® Fast-KPCA implementation bypasses the limitations of exact KPCA methods. SAS® internally uses k-means to find a representative sample of a subset of points. This row reduction method has the advantage that c centroids are chosen to minimize the variation of points nearest to each centroid and maximize the variation to the other cluster centroids. In some cases, the downstream effect of using k-means on computing the SVD increases numerical stability and improves clustering, discrimination, and classification.
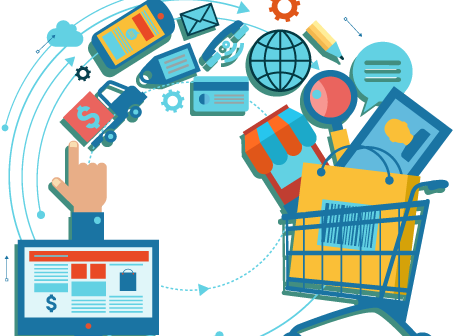
SAS' Yogender Kushawah introduces you to mining long sequences efficiently using the seqmc action in SAS Visual Analytics.
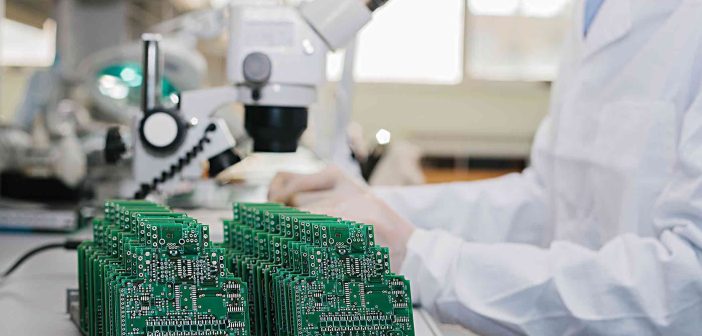
SAS' Bahar Biller and Xi Jiang use the example of a semiconductor manufacturing plant to illustrate the role of sensitivity analysis in assessing supply chain risk.
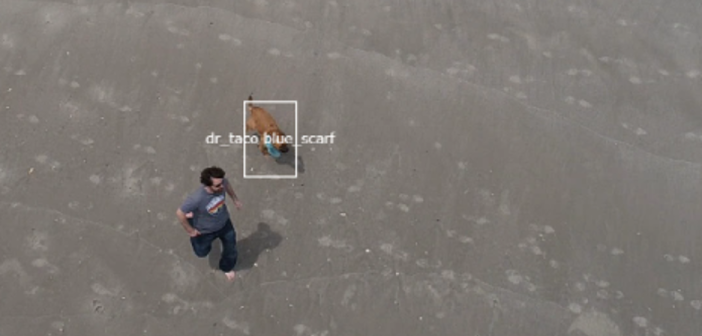
I will show you how to deploy multi-stage deep learning (DL) models in SAS Event Stream Processing (ESP) and leverage ESP on Edge via Docker containers to identify events of interest.
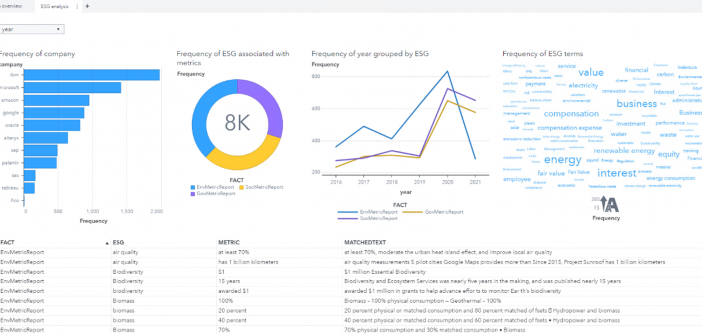
Using such features and Natural Language Processing capabilities like text parsing and information extraction in SAS Visual Text Analytics (VTA) helps us uncover emerging trends and unlock the value of unstructured text data.