The SAS Data Science Blog
Advanced analytics from SAS data scientists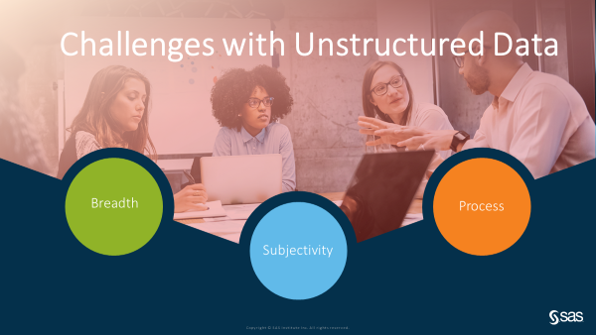
The Text Investigation Framework utilizes several technologies built on SAS Viya, including SAS Visual Text Analytics, SAS Visual Data Mining and Machine Learning, and SAS Visual Investigator. SAS Visual Investigator acts as the orchestrator to surface the results. With its broad set of capabilities, SAS Visual Investigator can perform scenario authoring, alert generation and disposition, and comprehensive workflow to gather vital outcomes and feedback.
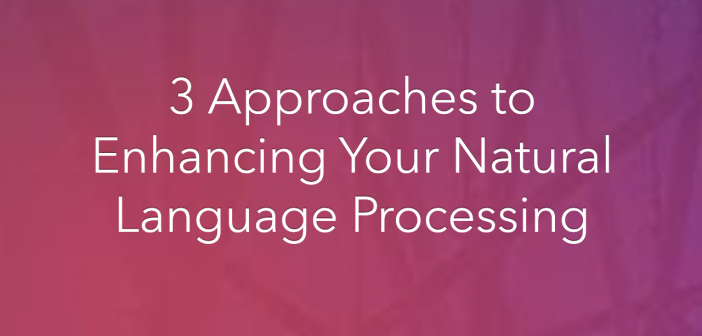
Unlocking the potential of your unstructured text data can lead to great business outcomes but the prospect of starting a new or enhancing your existing Natural Language Processing (NLP) program can feel overwhelming because of the inherently unique (and sometimes messy) nature of human language. Text data doesn’t fit neatly into rows or columns the way that structured data does, which can make it seem more complex to work with. Conversations and written language range from objective statements to subjective perspectives and opinions. The same sentence, depending on its intent and the nuances in how it's said, can have a positive, negative, or neutral sentiment. To get us started, we'll share different types of NLP models used to analyze unstructured data with a focus on the hybrid approach.
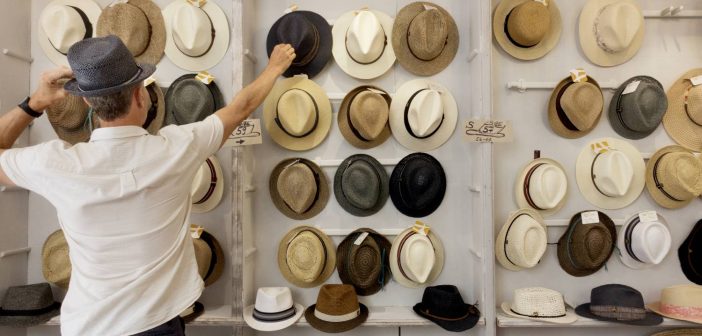
Remember Subconscious Musings? It was the name of the blog Radhika Kulkarni (now retired Vice President of SAS R&D) started in 2012. She wrote about trends that drove innovation and challenges that expanded the boundaries of what we thought was possible. It eventually evolved into what we now know as
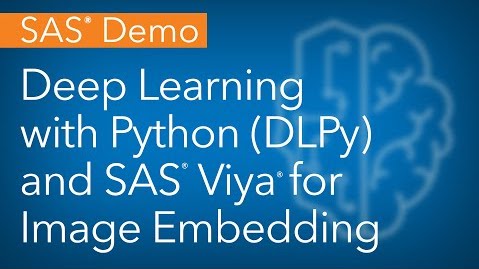
An embedding model is a way to reduce the dimensionality of input data, such as images. Consider this to be a type of data preparation applied to image analysis. When an embedding model is used, input images are converted into low-dimensional vectors that can be more easily used by other computer vision tasks. The key to good embedding is to train the model so that similar images are converted to similar vectors.
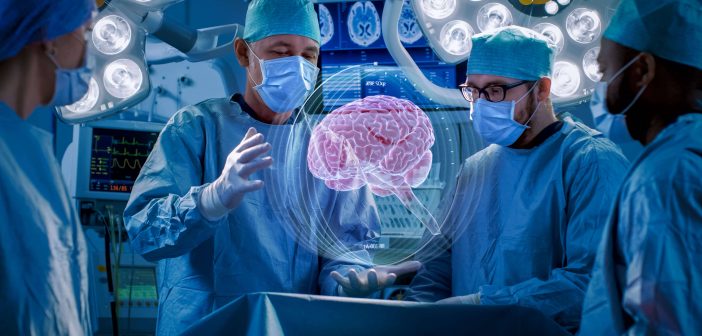
I spoke with Antonie Berkel and Dr. Joost Huiskens about a systems analysis project for the design of a clinical decision support system (CDSS) which integrates computer vision to help radiologists monitor the progression of tumors.
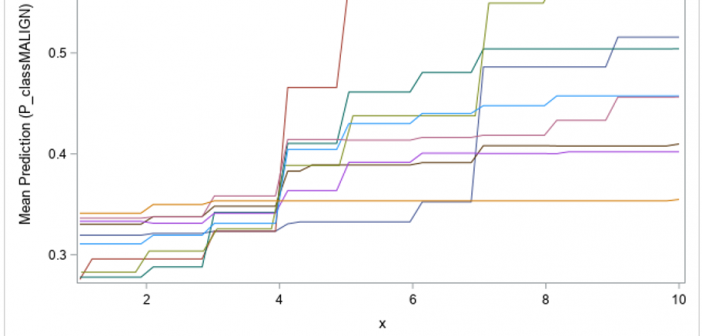
In the preceding two posts, we looked at issues around interpretability of modern black-box machine-learning models and introduced SAS® Model Studio within SAS® Visual Data Mining and Machine Learning. Now we turn our attention to programmatic interpretability.