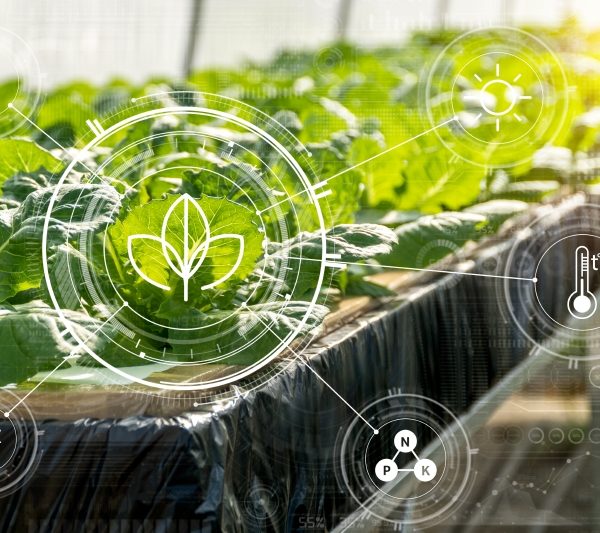
Many of us are lucky to have food on the table and grocery stores stocked with the food and beverages we want or need, and this is in a large part, due to our farmers. If you didn’t grow up on a family farm or a rural area where corn
Many of us are lucky to have food on the table and grocery stores stocked with the food and beverages we want or need, and this is in a large part, due to our farmers. If you didn’t grow up on a family farm or a rural area where corn
You've probably seen many graphs that are similar to the one at the right. This plot shows a regression line overlaid on a scatter plot of some data. Given a value for the independent variable (x), the regression line gives the best prediction for the mean of the response variable
Most of us know about electric vehicles (EVs), and the fact that they charge when connected to the grid. However, the technology is moving on. Instead of simply drawing from the grid, some EVs now have the capacity to feed energy to the grid from their batteries. This is known
La pandémie COVID-19 a mis en évidence des défis majeurs dans le secteur des sciences de la vie, mais elle a également identifié de réelles opportunités de changement et de modernisation. A l’avenir, il ne fait aucun doute que les données et l’analytique auront un rôle beaucoup plus important à
Images and artificial intelligence You don’t have to be a computer geek to know that artificial intelligence (AI) is having a moment in the sun just now. In recent years, we have made huge progress in the field of AI, and particularly through deep learning algorithms. These algorithms have been
Si vous travaillez dans le retail et que vous cherchez à mettre en œuvre, ou à remplacer vos solutions de planification ou d'optimisation, vous avez trois options : Développer une solution en interne Acquérir une solution tierce « best-of-breed » Utiliser une plateforme analytique qui peut s’adapter à vos processus
How coronavirus has led banks to reassess the value and purpose of local banking services
A previous article shows how to use a recursive formula to compute exact probabilities for the Poisson-binomial distribution. The recursive formula is an O(N2) computation, where N is the number of parameters for the Poisson-binomial (PB) distribution. If you have a distribution that has hundreds (or even thousands) of parameters,
When an election is on the horizon, I always feel compelled to plot some data! And this time I'm plotting North Carolina's voter registration data! State Data For this graph, I downloaded several of the data snapshots from the NC State Board of Elections' website, and plotted a line showing
By Sarah Gates, Analytics Platform Strategist at SAS, and Olivier Penel, Data & Analytics Strategic Adviser at SAS Today, organizations of all types are having to change their perspective – of how to do business, of how to collaborate with remote employees, of how to best engage with customers and
At SAS, we believe analytics is the force that drives change across organizations. Today, as change has been further accelerated, digital transformation is happening faster than anyone planned. Amid these advances, the use of analytics has become even more crucial, especially as a role in mission-critical applications. In 2020, even
The model management process, which is part of ModelOps, consists of registration, deployment, monitoring and retraining. This post is part of a series examining the model management process, orchestrated through the Model Manager (MM) APIs. The focus of part one is on model registration, specifically on using the APIs from
Collaboration and cooperation are a fact of life in every organization. However, how it's done in practice and how effective it is can vary significantly. What drives these differences and variations? Research shows that organizational culture is an important influence, especially the value placed on two main factors: relationships and
Interview mit Lehrstuhlinhaber für Data Science der Hochschule für angewandte Wissenschaften in Darmstadt, Professor Markus Döhring.
Technologies have driven business progress by providing innovative and effective ways to solve business problems. The financial sector is one of the most accepting of innovation, and the growing pressure from fintechs has encouraged other businesses to act. This has driven rapid incorporation of artificial intelligence (AI) processes and machine
Finite-precision computations can be tricky. You might know, mathematically, that a certain result must be non-negative or must be within a certain interval. However, when you actually compute that result on a computer that uses finite-precision, you might observe that the value is slightly negative or slightly outside of the
Text analytics: Theoretically, a telecoms company, say, could use topic modelling to look at product reviews divided into themes.
This is the decade of action. The United Nations urges this current generation to end extreme poverty, win the race against climate change and conquer injustice and gender inequality. But how do people take action when many are working and learning from home? How can individuals put the Sustainable Development
Seconds are passing us by at this very moment, and there's not much you can do in one second. Well, there is something. Let's say smile, take a deep breath, close your cellphone and say thank you. Within a second you can also make decisions: click the buy button in
A note from Udo Sglavo: The need for randomization in experimental design was introduced by the statistician R. A. Fisher in 1925, in his book Statistical Methods for Research Workers. You would assume that developing a successful treatment for COVID-19, the illness caused by the SARS-CoV-2 virus, will eventually conclude in
When the automatic time-series techniques can't produce adequately forecasts, a tool should be equipped with multiple machine learning techniques.
When working with a probability distribution, it is useful to know how to compute four essential quantities: a random sample, the density function, the cumulative distribution function (CDF), and quantiles. I recently discussed the Poisson-binomial distribution and showed how to generate a random sample. This article shows how to compute
Now that we are many months into the COVID-19 pandemic, I've started going back and reexamining the data for lessons or trends (you might say hindsight is 20/20). This time, I want to explore how COVID-19 has been spreading around the US. I do this by using a graphical idea
September is Suicide Prevention Awareness Month and Recovery Month, which have the important goals of preventing suicide and promoting the idea that recovery from behavioral health conditions is achievable. Amid an unprecedented year of stressors, 2020’s awareness months around behavioral health conditions have become more relevant to far more people. In recognition of the challenges and changes in people’s work lives,
The more information they have to learn from, the better. Naturally, this will not allow you to predict global pandemics or financial collapses.
The Poisson-binomial distribution is a generalization of the binomial distribution. For the binomial distribution, you carry out N independent and identical Bernoulli trials. Each trial has a probability, p, of success. The total number of successes, which can be between 0 and N, is a binomial random variable. The distribution
When you get something new, the hope is that it will be better than the old thing it's replacing. As I often do, I asked my Facebook friends to provide a random picture for my blog - in this case, a picture of one of their new/recent purchases. My friend
The Text Investigation Framework is a flexible solution for addressing text challenges across several domains. It was designed to create a process for turning unstructured text data into a decisioning system.
In the future this kind of data analysis can help to make an even better exoskeleton!
Les institutions gouvernementales que ce soit pour la défense, les transports, les services publics, la sécurité, ou les soins de santé ont un défi et une opportunité à traiter : donner un sens à d'énormes volumes de textes non structurés qui ne font que croître. Plus de 80 % de