The Data Roundtable
A community of data management experts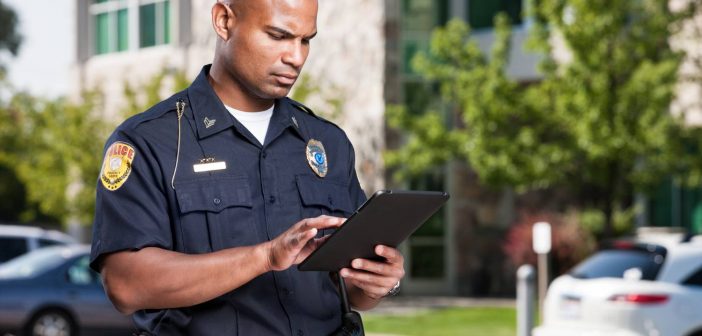
5 technology considerations for law enforcement
Having the right data analytics platform can help law enforcement solve crimes faster.
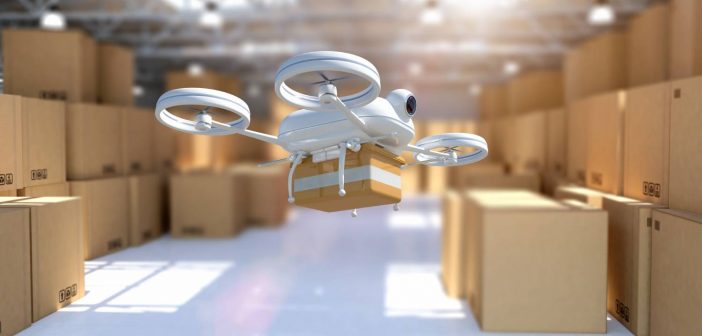
How containers have moved us to next-gen virtualization
With containers, you can build once, run anywhere – no worries about underlying dependencies.
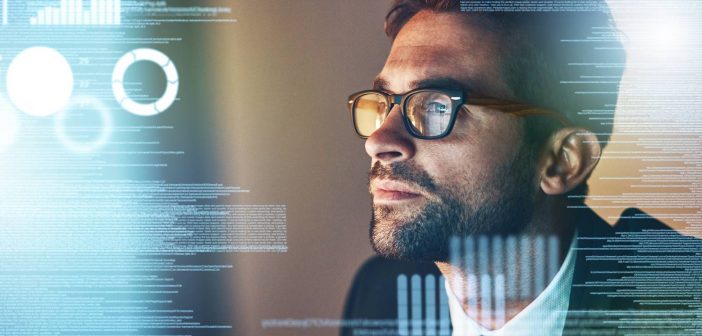
Alternative data may feel risky: Here's why it's not
Some businesses overlook alternative data. That's probably a mistake.
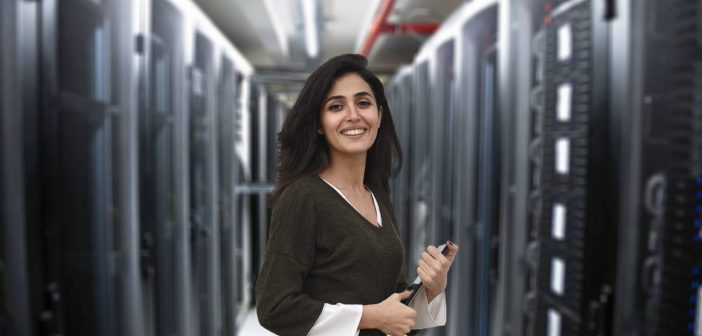
Get the scoop on data lake vs cloud
Jim Harris takes a deep dive into data lakes and how they relate to the cloud.
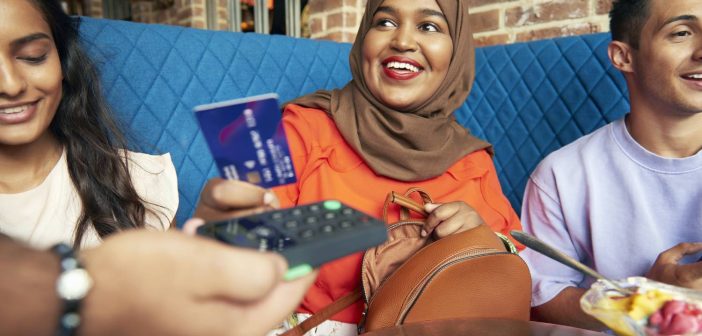
Building for the digital future of service
The evolution of service is simplicity and clarity. Build for the digital future of service today.
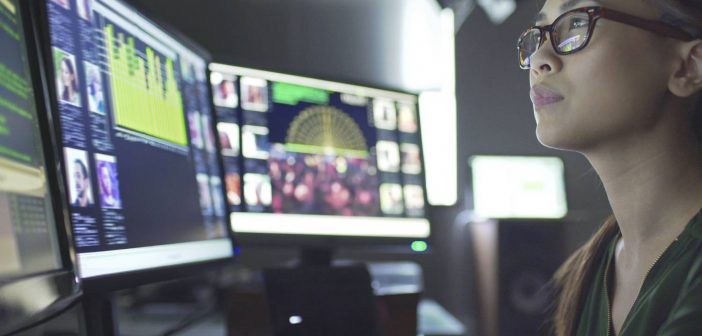
Why it's hard to say "big data" without saying "cloud"
Ready to turn big data into big business insights? Look to the cloud.