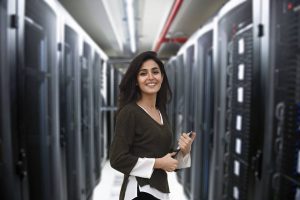
What's different with business technology today compared to 10 years ago? It might be better to ask what has not changed. Think about huge leaps in the capabilities of data and analytics technologies and platforms – like cloud computing and big data cloud – along with easier data access, remarkable differences in service delivery, and a growing need for enterprise-wide collaboration. To make the most of new business opportunities accompanying these trends, many organizations have turned to the data lake. But before we delve into why and how businesses are using data lakes, let's review some definitions and consider data lake vs cloud.
What is a data lake?
A data lake is a storage repository that can rapidly ingest large amounts of raw data in its native format. In turn, business users can quickly access it whenever they need it. And data scientists can easily analyze data in a lake to generate new insights. A lake can store data of all varieties, too – any source, any size, any speed, any structure. This includes:
- Structured data, such as rows and columns from relational database tables.
- Semistructured data, such as delimited flat text files and schema-embedded files.
- Unstructured data, such as social media content and data emanating from the Internet of Things (IoT).
Even before you define data structures and business requirements, a data lake can quickly consume incredibly large data volumes. And since it’s not restricted to a single structure, data lakes accommodate multi-structured data for the same subject area. For example, it's possible to blend structured sales transactions with unstructured customer sentiment in a data lake. And because it’s focused on storage, a data lake requires less processing power than other methods (like the data warehouse) – making it easier, faster and more cost-effective to scale up as data volumes grow.
Empowering business users
By providing fast (even real time), self-service data access, exploration and visualization to volumes of new data, data lakes empower business users to see and respond to new information sooner. A data lake also opens access to data businesses couldn't get in the past. These new – and new types of – data can be made available for data discovery, proofs of concept, visualizations and advanced analytics. For example, a data lake is the most common data source for machine learning – often applied to log files, clickstream data from websites, social media content, streaming sensors, and data emanating from other internet-connected devices.
Many businesses have long searched for a better way to perform discovery-oriented exploration, advanced analytics and reporting. Data lakes quickly provide the scale and data diversity needed for these pursuits. A data lake can also be a consolidation point for both big data and traditional data, enabling analytical correlations across all types of data.
Data lake tips, common misperceptions
It's important not to mistake a data lake for nothing more than a massive data repository. A data lake demands a well-designed data architecture along with proper planning, management and governance. Without this oversight, it can quickly devolve into a poorly managed and ungoverned data dumping ground akin to a data swamp.
An undocumented and disorganized data lake is also nearly impossible to navigate. That's why metadata tagging is one of the most essential data lake management practices – it makes the data in the data lake easier to find. It can be difficult to trust the data in a lake – so, data lake governance is important for vetting its usability for enterprise applications.
Another important data lake tip is to include it in your data and analytics strategy. Keep in mind that a data lake is only one part of an entire ecosystem of source systems, ingestion pipelines, integration and data processing technologies, databases, metadata, analytics engines and data access layers. Another common misperception – a data lake is not a data platform. Rather, it's a container for multiple collections of varied data coexisting in one convenient location.
You need a comprehensive platform to generate the most business value from a data lake – and that requires integration, cleansing, metadata management and governance. Leading organizations are taking this holistic approach to data lake management so that analytics can correlate diverse data from diverse sources in diverse structures. In turn, the data lake helps generate more comprehensive insights for the business. This can drive better decisions for things like product development, customer service and overall business strategy.
Data lake vs cloud
When businesses use a data lake as a centralized repository through which all enterprise data flows, it becomes an easily accessible staging area from which all enterprise data can be sourced. This includes data that's consumed by both on-siteand cloud-based applications. Which leads to the question: Where should the data lake be located? Data lake vs cloud implies choosing between an on-premise data lake and a cloud data lake.
Data lake in the cloud
You can certainly put your data lake in the cloud, and there are cloud data lake offerings worth considering. Using data lake storage in the cloud provides complementary benefits, to be sure. Those include elastic scalability, faster service delivery and greater IT efficiency – along with a subscription-based accounting model.
On-site data lake versus cloud
Many enterprises still opt for grounding their data lake within their own walls. The reasons are similar to the arguments made for managing a private cloud on-site.
Keeping a data lake on-site provides the utmost security and control while protecting intellectual property and business-critical applications. It also safeguards sensitive data in compliance with government regulations. But the disadvantages of managing a private cloud on-site also apply to a data lake. For example, managing the data lake on-site requires more in-house maintenance of the data lake architecture, hardware infrastructure, and related software and services.
Hybrid data lakes
Some enterprises decide to implement a hybrid data lake, which splits their data lake between on-site and cloud. In hybrid architectures, the cloud data lake typically stores less business-critical data, personally identifiable information (PII), or other sensitive data that has been obscured or anonymized. This helps the business comply with data security and privacy policies – and many analytical applications don't need those details anyway. In hybrid data lakes, the data stored in the cloud can be purged periodically or after pilot projects are completed to minimize cloud storage costs.
Read a TDWI report: Faster Insights from Faster Data