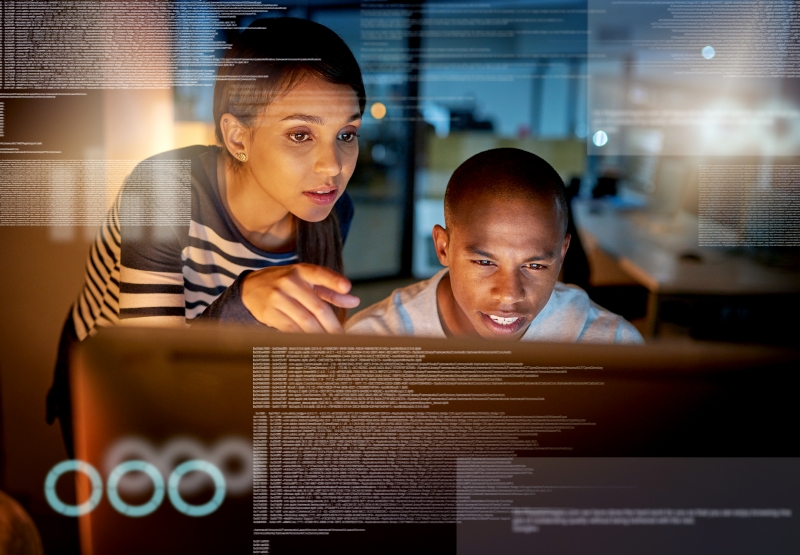
All analytics projects have data as their foundation and this data is usually spread across a variety of databases, storage systems and locations. This diverse and complex landscape causes data scientists to spend an inordinate amount of time searching for the right data and preparing this information for analytics. It’s