The Data Roundtable
A community of data management expertsOver my last two posts, I suggested that our expectations for data quality morph over the duration of business processes, and it is only at a point that the process has completed that we can demand that all statically-applied data quality rules be observed. However, over the duration of the
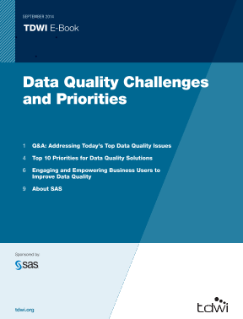
One of the significant problems data quality leaders face is changing people's perception of data quality. For example, one common misconception is that data quality represents just another data processing activity. If you have a data warehouse, you will almost certainly have some form of data processing in the form
In my last post, I pointed out that we data quality practitioners want to apply data quality assertions to data instances to validate data in process, but the dynamic nature of data must be contrasted with our assumptions about how quality measures are applied to static records. In practice, the

Utilizing big data analytics is currently one of the most promising strategies for businesses to gain competitive advantage and ensure future growth. But as we saw with “small data analytics,” the success of “big data analytics” relies heavily on the quality of its source data. In fact, when combining “small” and “big” data
@philsimon on the need to recognize DQ differences.
It’s common at the start of a new year to create a long list of resolutions that we hope to achieve. The reality, of course, is by February those resolutions will likely be a distant memory. The key to making any resolution stick is to start small. Create one small