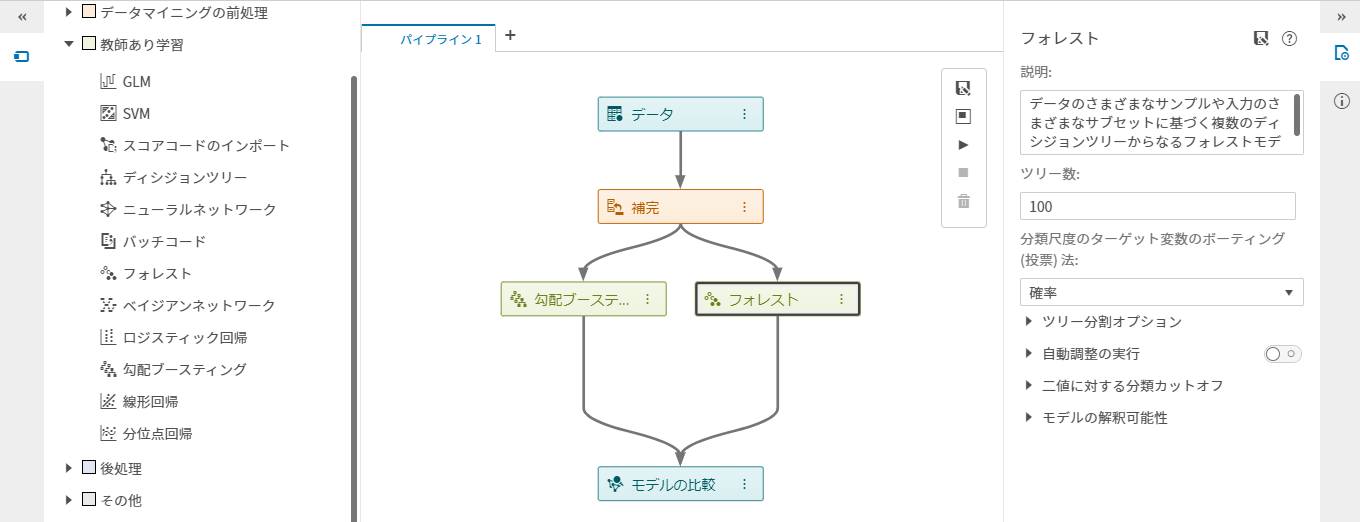
AIプラットフォームであるSAS Viyaでは、SAS言語のみならず、PythonやR、Java、Luaなどの汎用プログラミング言語からViyaのAI&アナリティクスの機能を使用し、予測モデルを生成することができるようになっています。しかし、昨今、「AI民主化」の流れに沿って、予測モデル生成を必要としているのはデータサイエンティスト(以降:DS)だけではなく、業務部門のビジネスアナリストや一般のビジネスユーザーも必要としています。こうしたコーディングスキルを持たないビジネスユーザー向けに、SAS Viyaでは、GUI上でマウスの簡単操作だけで予測モデル生成を可能としています。 もちろん、DSの中にも、コーディングせずに、もっと簡単に精度の高い予測モデルを生成できる手段があれば活用したいと感じている人達もいます。 SAS Viyaでは、Model Studioを使用し、機械学習のモデル、時系列予測のモデル、テキストマイニングのモデルをGUIベースの簡単マウス操作で作成することができます。モデル生成プロセスをグラフィカルなフロー図として描き、実行するだけです。このフロー図のことを「パイプライン」と呼んでいます。 Model Studioで予測モデルを生成するには、大きく2通りの方法があります。 1つは、マウスの簡単ドラッグ操作でパイプラインを一から作成する方法と、もう一つは、予め用意されているパイプラインのテンプレートを使用する方法です。 まずは、パイプラインを一から作成する際の基本的な手順を紹介します。 プロジェクトの新規作成と学習用のデータソース選択 パイプラインの作成と実行 実行結果(モデル精度)の確認 1.プロジェクトの新規作成と学習用のデータソース選択 SAS Viyaの統合GUIのホームページのメニューから「モデルの作成」を選択すると、 Model Studioの画面が表示されます。 「プロジェクトの新規作成」をクリックします。 「プロジェクトの新規作成」画面内で、プロジェクトの名前を入力し、モデルの種類(データマイニングと機械学習 / テキスト分析 / 予測)を選択し、学習用のデータソースを選択します。今回は、「データマイニングと機械学習」を選び、ローンの審査モデルを作成します。HMEQJというデータソースは、顧客ごとに1行の横持ち形式のデータです。 「保存」をクリックすると、ローン審査モデルプロジェクトが作成され、選択したデータソースの変数リストが表示されます。 予測対象の項目(ターゲット変数)を指定します。変数名:BAD(ラベル名:延滞フラグ)を選択し、右画面内で、役割に「ターゲット」を選択します。 延滞フラグには、過去に延滞の実績があればフラグに“1”が、無ければ“0”が設定されています。 2.パイプラインの作成と実行 予測対象の項目を指定後、画面上部にある「パイプライン」をクリックします。 パイプラインには「データ」ノードのみが表示されています。左端の機能ノードアイコンをクリックすると、 パイプラインに追加可能な機能ノードのリストが表示されます。 今回は、欠損値補完を行った上で、勾配ブースティングとランダムフォレストでモデルを生成してみましょう。まず、データに対する前処理として欠損値補完を行います。 「データマイニングの前処理」内にある「補完」を「データ」ノード上にドラッグすると、 「データ」ノードの下に「補完」ノードが追加されます。 同様の手順で、「教師あり学習」内にある「勾配ブースティング」を「補完」ノード上へドラッグすると、「補完」ノードの下に「勾配ブースティング」ノードが追加されます。(同時に「モデルの比較」ノードが自動的に追加されます) このようにドラッグ操作でノードを追加する以外に、パイプライン上のメニューからノードを追加することもできます。 「補完」ノードの右端にある、3つのドットが縦に並んでいる(スノーマン)アイコンをクリックし、「下に追加」>「教師あり学習」>「フォレスト」の順に選択すると、 「補完」ノードの下に、「フォレスト」ノードが追加されます。 機能ノードごとの詳細なオプションの設定は、右側画面内で行います。 パイプラインが完成したら、パイプラインの実行アイコンをクリックし、実行します。実行中の機能ノードは時計アイコンがクルクル回転し、正常に完了すると緑のチェックマークが表示されます。 3.実行結果(モデル精度)の確認 パイプラインの実行が完了したら、「モデルの比較」ノードのスノーマンアイコンをクリックし、メニュー から「結果」を選択します。 モデルの比較結果が表示されます。今回は勾配ブースティングのモデルの精度の方が高い=チャンピオンであると判定されています。 「アセスメント」タブ内では、リフトやROCの情報などを確認することができます。 以上が、ビジュアルパイプラインで予測モデルを一から生成する際の基本的な手順です。 ※ビジュアルパイプラインによるモデル生成(基本)は、SAS Viya特設サイトの「機械学習」トピック内にある動画でもご覧いただけます。