Get the latest machine learning algorithms and techniques
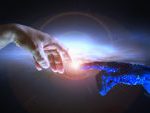
About four weeks ago i attended the ISF forecasting conference in Thessaloniki for the first time. It was a great conference with a lot of focus on forecasting methods. This year one of the hot topics was how to use machine learning in forecasting. One of the key questions was