With the proliferation of aggregator websites, such as comparethemarket.com and confused.com, customers now have more choice than ever when selecting an insurer. Gone are the days of long-term customer loyalty and traditional company choice. Instead, when customers face the annual continuation reminder and frequent premium increase, they have easy access to a range of providers. And they head online for their options. The aggregators make the selection process simple. There are no more interminable and intrusive forms. After a few quick and easy prompts, the quote is before the customer with cheaper offers and enticements.
The consequence of this for insurers is that the loss ratio (claims paid out vs. premiums earned) is very tight. At 100%, this means an insurer is neither making nor losing money. Currently, this figure is lurking at around 99%. What does this mean? It means that it is vital to get the price right, coupled with the need to create a far higher volume of business. It is obvious, but it must be acknowledged; in the current climate insurers need to sell more policies.
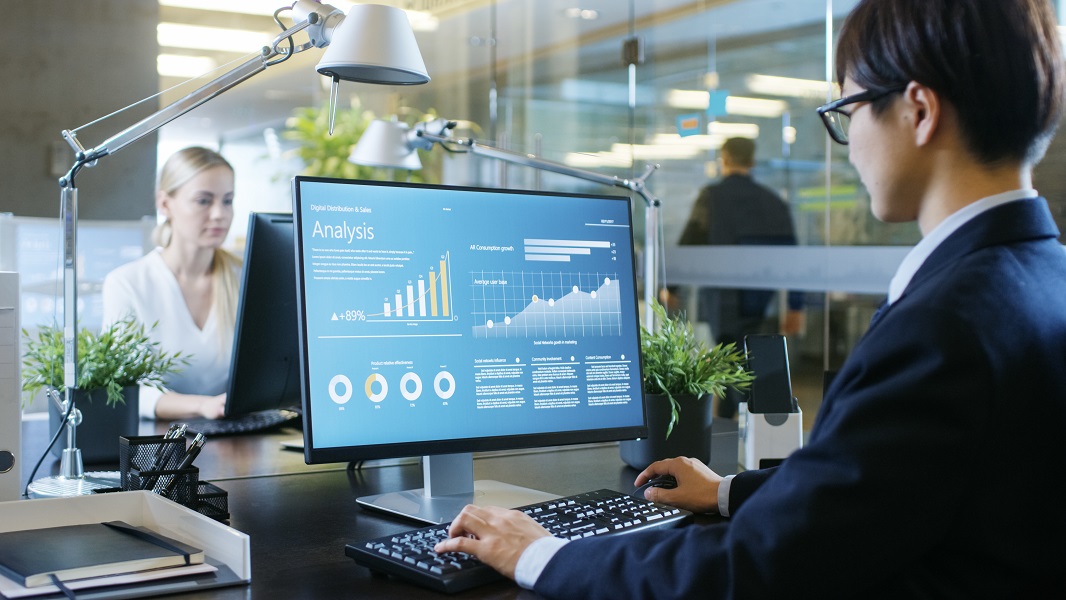
Pricing insurance policies
What happens if insurers do not get the price right? Overpricing a policy, giving the customer a higher premium whilst their risk profile (their chances of having an accident, making a claim or having their vehicle stolen, etc.) is low, means there is a high chance of losing that safe customer. Conversely, pricing the policy lower than it ought to be means the insurer is bearing the brunt of the risk, rather than having the customer pay for it.
Additionally, insurers face any number of inflationary issues. These include greater data volumes, new data streams, GDPR and a changing regulatory landscape.
So what is wrong with the current way of pricing? It is not so much wrong as outdated. Pricing insurance policies is usually done based on one’s risk profile. When customers apply for a quote, they submit their details, name, address, job, etc. Then the insurer calculates the risk of that customer segment with analytical models. There are two sets of pricing that need to be calculated for a customer.
- Technical pricing, the minimum insurance price for a policy (baseline). This is what the insurer can charge at a minimum but make no profit.
- Commercial pricing, what the insurer ought to charge. This uses the technical/ baseline price but includes other important information. The insurer may want to target all females over 40 (hence offer a reduction in premium) or have less under age 30 in their portfolio (and offer a higher premium to deter them). The calculation would need to include this logic.
What’s changed
- Pricing models - In this increasingly competitive age, the analytical models are not providing good enough pricing. Insurers tend to use generalised linear models (GLM) to produce pricing. GLMs assume a linear relationship – which may not be the best model to use. For example, look at the relationship between age and risk. If you model the relationship using a GLM, you are broadly saying as the age of a customer increases, the risk decreases. This is likely to be true with younger drivers being riskier until a certain age, of course, where there is an increased risk for elderly drivers. So GLMs are not necessarily the best model to use.
- Real-time pricing - Insurers do not usually provide tailored pricing to the customer. Instead, they calculate it for a segment, a group of people who share similar attributes. Insurers then give this pricing data to the aggregators and provide it to customers when they request a quote. Being able to provide real-time pricing tailored to the individual customer would inevitably improve accuracy.
- New data sources - The use of telematics is becoming more popular. Telematics gathers data from "black boxes" installed in customer cars, which assess how well you are driving. This allows customers to get reduced premiums – but also gives insurers the ability to assess the risk of the customer. Insurers need to incorporate this new data source into new pricing models.
Transforming how to price
SAS seeks to address the challenges discussed above by providing an end-to-end solution to streamline the pricing process. SAS provides a comprehensive suite of AI and machine learning techniques to address the limitations of GLM models, including gradient boosting, random forest, neural networks, decision trees and many more. Furthermore, it allows you to incorporate unstructured data, such as telematics or data from wearables, which currently cannot be modelled with GLMs.
SAS also includes data management and data preparation capabilities. You can use them to incorporate new data sources, such as telematics. But they also help you get your data in the right form – which many insurers still struggle with – irrespective of data volumes. Some insurers have turned to open source for AI and machine learning algorithms but have hit a wall when attempting to operationalise the models. SAS provides the ability to seamlessly incorporate, cleanse and shape the data, extensive modelling techniques and intelligent decisioning to derive the optimum policy price and provide tailored quotes to individual customers in real time.
SAS did just that for an insurance company that was struggling with over- and underpricing its policies. Not only was it able to uplift pricing accuracy through benchmarking an array of advanced machine learning algorithms – including gradient boosting and neural network techniques – but also provide those prices in real time via REST APIs. This led to a reduction in loss ratio by 1%.
AI and machine learning can help insurers streamline a customer centric pricing process. Click To TweetTo find out more about how SAS can help with pricing and actuarial transformation, I will be interviewing Georgios Kapetanvasileiou, SAS Analytics Consultant, during a 30-minute webinar on 31 October at 2 p.m. UK time about Calculating risk at the segment of one: the future of insurance.