Move beyond spreadsheets to data mining, forecasting, optimization – and more
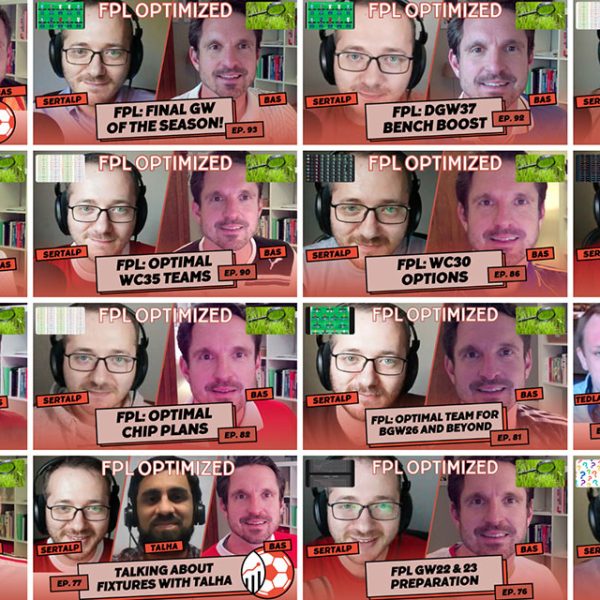
Fantasy sports are all about making the right calls—do you trust your gut, or do you trust the data? In fact, sports and data are a powerful combination and nowhere is that more evident than in the world of fantasy sports. Whether it's the excitement of the Premier League or