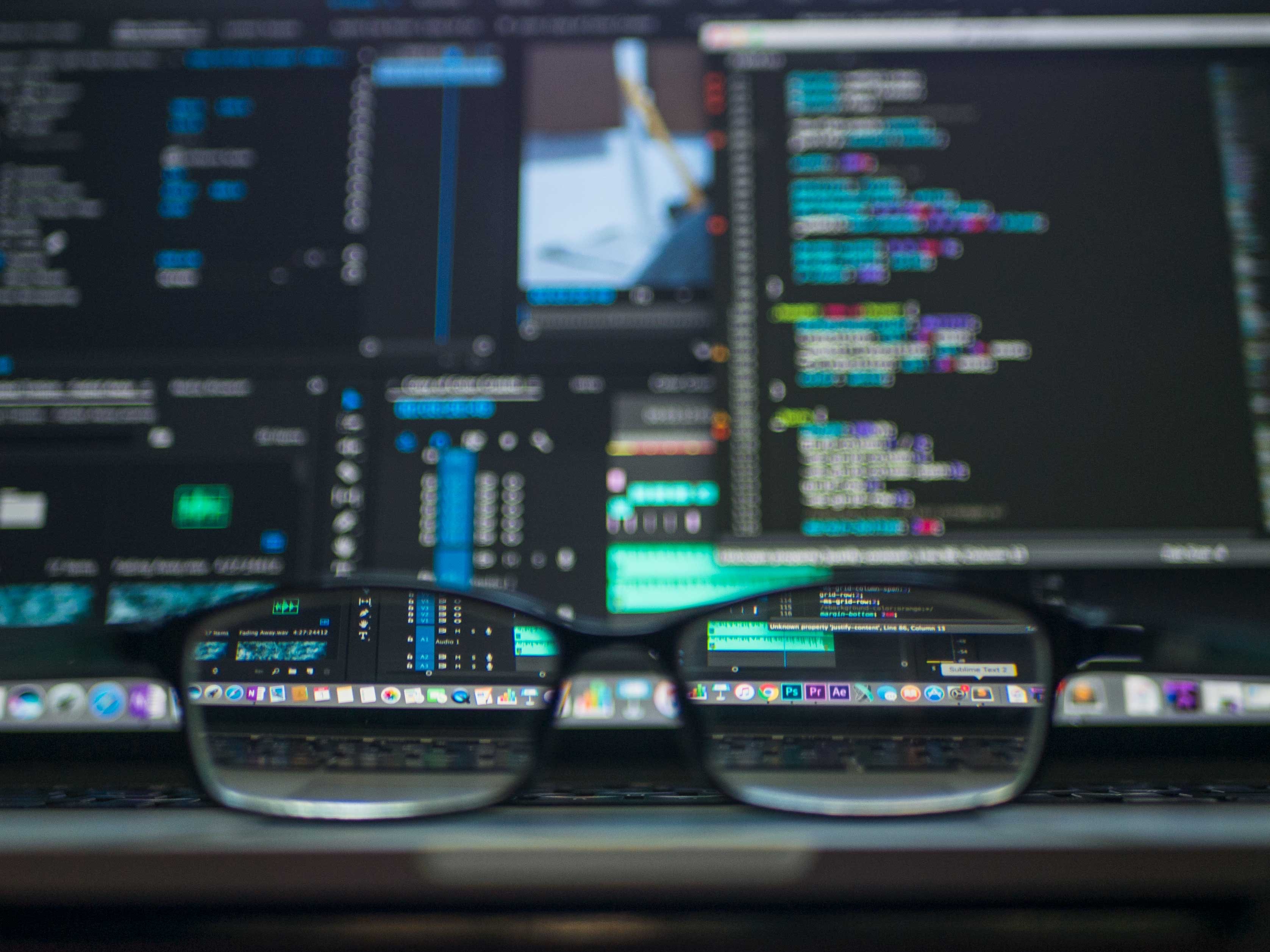
今回は「オペレーショナル・アナリティクス for Data Scientist」をメインテーマとしてご紹介します。企業で分析業務を行うデータサイエンティストの皆様はご存知の通り、モデルは開発しただけでは意味がありません。そのモデルを業務に実装(デプロイ)してはじめて、ビジネス課題を解決し、価値を創出することができるわけです。SASが長年蓄積してきたナレッジをご覧ください。 1.Using SAS® Viya® to Implement Custom SAS® Analytics in Python: A Cybersecurity Example この論文は、SASの分析機能により支えられているプロダクションレベルのアナリティクスソリューションを開発しようとしているデータサイエンティストを対象としています。本文では、SAS ViyaとCloud Analytics Service(CAS)に基づく、CASの構築基盤とサイバーセキュリティを説明します。そして、SASアナリティクスを本番環境でPythonで実装する方法を説明します。 2.What’s New in FCMP for SAS 9.4 and SAS Viya この論文では、下記いくつかポイントをメインとして議論していきます。まず、SASが提供しているFunctionコンパイラー(FCMP)の新しい特徴を紹介し、それから主にFCMPアクションセットを中心とし、リアルタイムアナリティクススコアリングコンテナ(ASTORE)とPythonのインテグレーションについても説明します。それらの説明により、SASの新しいテクノロジーに対し、更なる理解を頂けることを期待しています。 3.Influencer Marketing Analytics using SAS® Viya® この論文はSAS Viyaを使って、マーケティングアナリティクスを行う事例を紹介します。近来、マーケティングはますますインフルエンサーが大きな役割をしめるようになってきています。それらのインフルエンサーたちはソーシャルメディアのコンテンツ作成者であり、多くのフォロワーを持ち、人々の意見に影響を与え、購入を検討する人々にも影響を与えています。インフルエンサーマーケティングは、より伝統的なマーケティングチャンネルと同じようにコストがかかるため、企業にとって最も効果的なインフルエンサーを選択することは非常に重要です。 こういった背景において、この論文では、ソーシャルメディアで本当に影響力をもつ人、そしてその影響程度はなにかについて分析することを目指しています。ケーススタディは、感情面の影響を与えることに焦点を当てています。また、多くのフォロワーを持つインフルエンサーとその色んな投稿とアクティビティを分析します。実施するには、Pythonのライブラリとコードが使用されます。次に、彼らのアクティビティとネットワークを分析して、それらの影響範囲を分析します。これらの分析には、SAS Viyaのテキストおよびネットワーク分析機能が使用されます。データ収集ステップ(Python)はクライアントとしてJupyter Notebookを使用していますが、分析ステップは主にSAS Visual Text Analytics(Model Studio)とSAS Visual Analyticsを使用して行われています。 4.Take