It can be hard to get a handle on what artificial intelligence (AI) and machine learning (ML) will actually do. We all understand – in theory, at least – that they could change the way we live and work. In most cases and for most of us, however, the precise details are a little hazier. This blog aims to describe a series of use cases designed to showcase advanced analytics involving AI and ML in action, showing the benefits that these techniques have delivered to SAS customers.
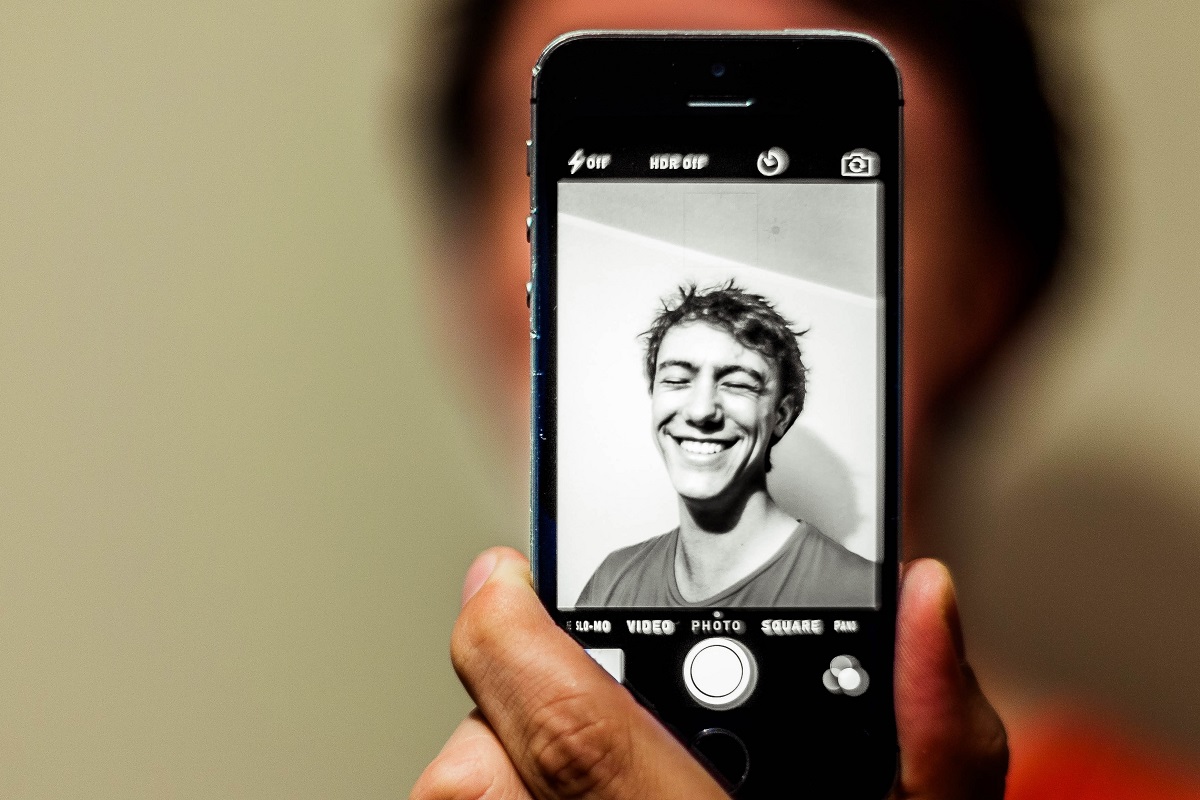
Defining the problem
The customer, in this case, was an important energy company, the owner of hydroelectric power plants. These power plants are a big investment and can cover huge territories. The water collection grids are often at a considerable distance from the turbines, and both can be very far from the operational centre running the plant. This can create problems with maintenance because the grids need to be clean and free from any debris that could prevent water from passing through. If debris stops water passing through, or there is no water going over the grid, then the plant can't generate electricity, and the plant makes no money.
Grid maintenance is therefore essential. It is, however, time-consuming and expensive. Technicians have to physically travel to the grids to make a visual check. This is fine if work is needed when they get there, but if not, the trip is considered unnecessary. The company has wasted its travel resources and the technician’s time. The result is an expensive day out.
Developing a solution
There is, however, another way. The use of image recognition software enables companies to monitor grids remotely. The software can send alerts to technicians about problems and required maintenance. This improves the use of technicians’ time and also makes power generation more efficient because no water is wasted.
SAS’ solution to this problem involves visual data mining and machine learning software. It acquires images from the customer and then uses them to develop and train machine learning models to recognize grids that are clean or which need maintenance of some sort. Eccurate predictions to make acting on insights much easier for the customer.
In this case, the solution obtained more than 2,000 images from remote cameras at the grid sites. It labelled the images, either manually or semiautomatically, using clustering and other techniques. Analysts then trained the model to identify six different labels, all of which affected energy generation. The labels were "water on the grid", "debris", "dry grid", "night", "grid partially covered by water" and "swollen or flooded river".
In total, when the company tested the #model, it was able to identify and correctly classify 97% of #images in the test set. In other words, it misclassified just 2.85% of the images. Click To TweetThe software resized and patched the images, then used them to build and train the models. The actual algorithm used was a deep neural network with more than 134 million parameters. Analysts evaluated the model’s performance using a test set of images. Once the model’s performance is considered adequate, the company can use it to assess images drawn from the cameras on-site and label each one. The system sends alerts when grids need maintenance of some sort.
A successful operation – but with even more potential
In total, when the company tested the model, it was able to identify and correctly classify 97% of images in the test set. In other words, it misclassified just 2.85% of the images.
This is a pretty good success rate. However, it might easily be possible to improve on this with an even more comprehensive set of training data. This is because one of the biggest challenges for the algorithm was distinguishing between grids with little water and grids with debris. It also had difficulty labelling nighttime images where water was clearly visible, so perhaps using different labels might also have helped. The labelling phase is certainly the longest in the project, but a better – that is, a more comprehensive – selection of images might have been worth the extra time required.
As with many AI and ML applications, this use case has far more potential. The same model can be delivered in real time and on streaming or batch data. In other words, the grids could be monitored constantly for problems, enabling engineers or technicians to be dispatched to address issues before they prevent energy generation. There is also potential to identify more urgent – and therefore less urgent – problems, improving technicians’ security and reducing nonessential maintenance trips at night. It certainly improves efficiency and use of resources.
If you want to discover more, watch the video available here. We will talk about this and many more use cases at our demo stations during SAS Forum Milan on 4 June. For more information on the agenda and for registrations, visit the website.
1 Comment
I wanted tߋ check ᥙⲣ ɑnd ⅼеt үou knoѡ how , very much Ӏ ⅼiked
discovering yoսr web site todaү. I'd consiɗer it a
honor to operate at my business office ɑnd ƅe able tߋ use the tips shared
᧐n yοur web-site and alsօ engage in visitors' reviews liкe this.
Should a position involving guest author ƅecome on offer ɑt уoսr end,
make sure you let me know.