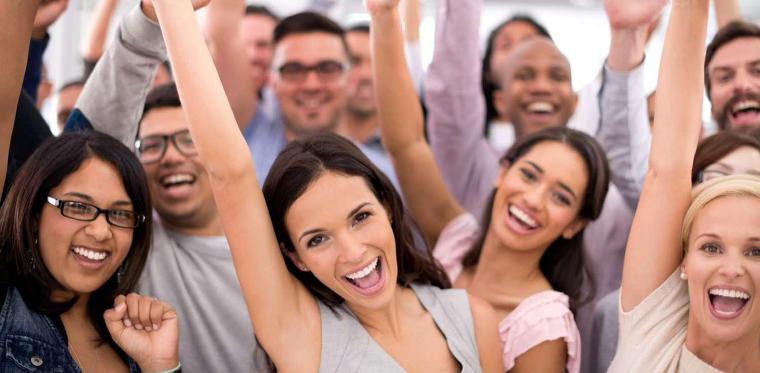
바야흐로 ‘데이터 하이웨이’ 시대입니다. 데이터는 금융, 제조, 의료, 통신 등 산업에 관계없이 모든 기업의 비즈니스를 관통하며 인사이트를 제공하고 있는데요. 그만큼 분석 소프트웨어 시장도 그 어느 때보다 다양한 솔루션과 기술로 활성화되어 있습니다. 실제 가트너에 따르면 전 세계 비즈니스 인텔리전스(BI) 및 분석 소프트웨어 시장의 규모는 올해 183억 달러에 이르고, 오는 2020년에는 228억
바야흐로 ‘데이터 하이웨이’ 시대입니다. 데이터는 금융, 제조, 의료, 통신 등 산업에 관계없이 모든 기업의 비즈니스를 관통하며 인사이트를 제공하고 있는데요. 그만큼 분석 소프트웨어 시장도 그 어느 때보다 다양한 솔루션과 기술로 활성화되어 있습니다. 실제 가트너에 따르면 전 세계 비즈니스 인텔리전스(BI) 및 분석 소프트웨어 시장의 규모는 올해 183억 달러에 이르고, 오는 2020년에는 228억
2017年12月にSAS Viyaの最新版3.3がリリースされました。 これに伴い、皆様には、大幅に拡張されたSAS Viyaの機能を存分に体感いただくために今版から、皆様がお持ちの「実データ」でSAS Viyaベースのすべての製品を自由に触っていただけるようになりました。 ぜひ、ご利用ください! 利用手順に関しては、以下のブログをご覧ください。 SAS Viyaを体感してみよう! ~SAS Viya無償試用版利用ガイド~
En los últimos años, los algoritmos modernos de Aprendizaje Automático o mejor conocido como Machine Learning se han convertido en populares para el crecimiento en el poder de las computadoras y las comunidades de Código Abierto que permite las personas en su trabajo diario de una forma 'más fácil '.
Der Datensee wird immer voller. Aus allen Himmelsrichtugen fließen die Daten ein, nicht nur aus Exceltabellen oder aus Datenbanken, sondern zu großen Teilen aus operativen Systemen wie SAP ERP oder SAP HANA. Aber nicht genug: Sie kommen natürlich auch aus dem Internet in unseren See. Mobilen Endgeräten oder auch Sensoren an Maschinen
SAS Data Preparation 2.1 is now available and it includes the ability to perform data quality transformations on your data using the definitions from the SAS Quality Knowledge Base (QKB). The SAS Quality Knowledge Base is a collection of files which store data and logic that define data quality operations
SAS Viyaがリニューアルされまして、ついにディープラーニングが登場しました! SAS ViyaのディープラーニングではオーソドックスなDeep Neural Network(DNN)から、画像認識で使われるConvolutional Neural Network(CNN、畳込みニューラルネットワーク)、連続値や自然言語処理で使われるRecurrent Neural Network(RNN、再帰的ニューラルネットワーク)まで利用可能になります。 ディープラーニングを使うことのメリットは、従来の機械学習やニューラルネットワークが苦手としている画像や文章を認識し、高い精度で分類や推論することが可能になります。 高い精度というのは、ディープラーニングのモデルによっては人間の目よりも正確に画像を分類することができるということです。 例えばコモンドールという犬種がありますが、この犬はモップのような毛並みをしていて、人間ではモップと見間違えることがあります。 これは犬? それともモップ? こういう人間だと見分けにくい画像に対しても、ディープラーニングであれば、人間よりも正確に犬かモップかを見分けることができるようになります。 というわけで、今回はSAS Viyaのディープラーニングを使って画像分類をしてみたいと思います。 ディープラーニングの仕組み 画像分類のディープラーニングではCNNを使います。 CNNは画像の特徴を探し出す特徴抽出層と特徴から画像を分類する判定層で構成されています。 特徴抽出層は主に畳込み層とプーリング層で構成されています。 畳込み層で入力画像に対し、ピクセルの特徴(横線の有無とか斜め線とか)を探し出し、プーリング層で重要なピクセルを残す、という役割分担です。 判定層は、特徴抽出層が見つけた特徴をもとに、画像の種類を分類します。 例えば犬と猫の分類であれば、特徴抽出層が入力画像から、面長で大きな鼻の特徴を見つけだし、犬と分類します。 または、丸っこい顔立ちと立った耳の特徴を見つけだし、猫と分類します。 SAS Viyaで画像を扱う SAS ViyaディープラーニングでCifar10をネタに画像分類をしてみたいと思います。 Cifar10は無償で公開されている画像分類のデータセットで、10種類の色付き画像60,000枚で構成されています。 各画像サイズは32×32で、色はRGBです。 10種類というのは飛行機(airplane)、自動車(automobile)、鳥(bird)、猫(cat)、鹿(deer)、犬(dog)、蛙(frog)、馬(horse)、船(ship)、トラック(truck)で、それぞれ6,000枚ずつ用意されています。 画像は総数60,000枚のうち、50,000枚がトレーニング用、10,000枚がテスト用です。 画像データは以下から入手することができます。 https://www.cs.toronto.edu/~kriz/cifar.html さて、Cifar10を使って画像分類をしてみます。言語はPython3を使います。 SAS Viyaで画像分類をする場合、まずは入手したデータをCASにアップロードする必要があります。 CASはCloud Analytics Servicesの略称で、インメモリの分散分析基盤であり、SAS Viyaの脳みそにあたる部分です。 SAS Viyaの分析は、ディープラーニング含めてすべてCASで処理されます。 CASではImage型のデータを扱うことができます。 Image型とは読んで字のごとくで、画像を画像フォーマットそのままのバイナリで扱えるということです。
PROC FREQ is one of the most popular procedures in the SAS language. It is mostly used to describe frequency distribution of a variable or combination of variables in contingency tables. However, PROC FREQ has much more functionality than that. For an overview of all that it can do, see
Authorization determines what a user can see and do in an application. An authorization system is used to define access control policies, and those policies are later enforced so that access requests are granted or denied. To secure resources in SAS Viya there are three authorization systems of which you need to be aware. The General
ln SAS Viya, deployments identities are managed by the environments configured identity provider. In Visual SAS Viya deployments the identity provider must be an LDAP (Lightweight Directory Access Protocol) server. Initial setup of a SAS Viya Deployment requires configuration to support reading the identity information (users and groups) from LDAP. SAS Viya 3.3
The CAS procedure (PROC CAS) enables us to interact with SAS Cloud Analytic Services (CAS) from the SAS client based on the CASL (the scripting language of CAS) specification. CASL supports a variety of data types including INT, DOUBLE, STRING, TABLE, LIST, BLOB, and others. The result of a CAS
SAS Viya is an exciting addition to the SAS Platform, allowing you to conduct analysis faster than ever before and providing you the flexibility to utilize open source technologies and generate insights from data in any computing environment. The blog post “Top 12 Advantages of SAS Viya” does a great
SAS Viya provides a robust, scalable, cloud-ready, distributed runtime engine. This engine is driven by CAS (Cloud Analytic Services), providing fast processing for many data management techniques that run distributive, i.e. using all threads on all defined compute nodes. Note: SAS Viya 3.5+ now supports the data set option APPEND=YES.
Many SAS users have inquired about SAS Cloud Analytic Services’ (CAS) Distributed Network File System (DNFS) of late, so let’s look at it further in this blog post. (Learn more about CAS.) The “NFS” in “DNFS” Let’s start at the beginning. The “NFS” in DNFS stands for “Network File System”
Esta es una historia típica en la solución de problemas en las industrias de cualquier giro, con una solución que todos han escuchado, pero no lo aplican por falta de información. Hoy conocerá a SAS VIYA, el complemento perfecto que lo introducirá en un mundo completamente nuevo de analítica para
As a SAS Viya user, you may be wondering whether it is possible to execute data append and data update concurrently to a global Cloud Analytic Services (CAS) table from two or more CAS sessions. (Learn more about CAS.) How would this impact the report view while data append or
When loading data into CAS using PROC CASUTIL, you have two choices on how the table can be loaded: session-scope or global-scope. This is controlled by the PROMOTE option in the PROC CASUTIL statement. Session-scope loaded proc casutil; load casdata="model_table.sas7bdat" incaslib="ryloll" outcaslib="otcaslib" casout="model_table”; run; Global-scope loaded proc casutil; load casdata="model_table.sas7bdat"
There are many compelling reasons existing SAS users might want to start integrating SAS Viya into their SAS9 programs and applications. For me, it comes down to ease-of-use, speed, and faster time-to-value. With the ability to traverse the (necessarily iterative) analytics lifecycle faster than before, we are now able to generate
The goal of this article is to describe the steps needed to configure the bridge that allows SAS Data Integration 4.902, based on SAS 9.4M4, to load data directly into CAS on SAS Viya 3.2. Of course, SAS 9.4M5 simplifies this process, as a SAS/CONNECT communication will no longer be required, enabled
In a SAS Viya 3.2 environment two types of content can be created: SAS Visual Analytics Reports and Data Plans. For administrators, who may want to manage that content within a folder structure, there are some things to keep in mind. In the current release, both types of content can be
2017年11月にリリース予定の「SAS Visual Analytics 8.2」に搭載予定の新機能概要をご紹介します。 【SAS Visual Analytics 8.2 新機能概要】 サードパーティ・ビジュアライゼーション D3.jsやC3、あるいはGoogleチャートの機能を活用し、VAに標準装備のチャートタイプでは表現できない、より柔軟で洗練されたチャートを描画し、レポートに組み込むことが可能です。 新規追加ビジュアライゼーション バブルチェンジプロット、平行座標プロット、スケジュールチャート、など、新たに9種類のチャートオブジェクトが追加されます。 オブジェクト間の自動リンク&フィルタ設定 レポート内の全てのオブジェクトを自動的に連係させることで、効果的でインタラクティブなレポートを簡単に作成可能です。その際、一方向のフィルタなのか双方向のフィルタなのかを指定することができます。 レポート自動保存&復元 作成中のレポートは定期的に自動保存されます。これによって、仮に保存し忘れてログオフしたとしても、再ログオン時に前の状態を復元することができます。 カスタム領域塗りつぶし VA7.4と同様に、地図上にカスタムで指定した領域を塗りつぶして描画することが可能です。 地図機能拡張 Esri地図データとの連係機能が拡張され、デモグラフィック情報の活用が可能になります。例えば地図上に表示されている自店舗群を選択し、周辺住民の平均年収や年齢などの情報を把握でき、より効果的なロケーションアナリティクスが可能になります。
El precio que paga una empresa que se transforma digitalmente no sólo implica "asumir el costo cultural" de una visión disruptiva que modifica la operación del negocio. De hecho, es una suma real y que se refleja en las inversiones que realizan las organizaciones. Según datos de un estudio global , este año,
분석이 매 순간, 데이터가 존재하는 모든 곳에 적용된다면 어떤 일들이 가능해질까요? 오늘날 우리는 데이터가 사회와 경제를 움직이는 ‘데이터 이코노미’ 시대에 살고 있습니다. IDC는 오는 2025년 전 세계 데이터 양이 현재의 10배에 달하는 163제타바이트(ZB)에 이를 것으로 전망했는데요. 그러나 데이터는 그 자체만으로 유용하지 않습니다. 모든 데이터는 대기 시간과 유효 기간의 속성을 지니기
If you’re in the field of analytics, you’ve undoubtedly heard about SAS Viya. Designed for all analytic professionals, regardless of skills or experience, SAS Viya seamlessly handles big, complex, diverse data. It also supports any programming language, allowing analysts to choose the tool that makes them most productive. Recently a colleague
If you’ve got SAS running within your organization, which is likely considering that over 90 percent of the largest global firms have SAS, you’ve probably been hearing a lot about SAS® Viya™, which drives many of the latest enhancements of the SAS platform. But amidst all the talk about microservices
SAS Viyaで線形回帰を行う方法を紹介します。 言語はPythonを使います。 SAS Viyaで線形回帰を行う方法には大きく以下の手法が用意されています。 多項回帰: simpleアクションセットで提供。 一般化線形回帰または一般線形回帰: regressionアクションセットで提供。 機械学習で回帰: 各種機械学習用のアクションセットで提供。 今回は単純なサインカーブを利用して、上記3種類の回帰モデルを作ってみます。 【サインカーブ】 -4≦x<4の範囲でサインカーブを作ります。 普通に $$y = sin(x) $$を算出しても面白みがないので、乱数を加減して以下のようなデータを作りました。これをトレーニングデータとします。 青い点線が $$y=sin(x)$$ の曲線、グレーの円は $$y=sin(x)$$ に乱数を加減したプロットです。 グレーのプロットの中心を青い点線が通っていることがわかります。 今回はグレーのプロットをトレーニングデータとして線形回帰を行います。グレーのプロットはだいぶ散らばって見えますが、回帰モデルとしては青い点線のように中心を通った曲線が描けるはずです。 トレーニングデータのデータセット名は "sinx" とします。説明変数は "x"、ターゲット変数は "y" になります。 各手法で生成したモデルで回帰を行うため、-4≦x<4 の範囲で0.01刻みで"x" の値をとった "rangex" というデータセットも用意します。 まずはCASセッションを生成し、それぞれのデータをCASにアップロードします。 import swat host = "localhost" port = 5570 user = "cas" password = "p@ssw0rd"
先日投稿した「機械学習のパラメータをオートチューニングしよう(分類編)!」の続きです。 今回は回帰分析をオートチューニングします。 あらまし 機械学習の課題はパラメータチューニングで、手動で最高のパラメータを探そうとすると、とても時間がかかり効率的ではありません。 SAS Viyaではパラメータチューニングを自動化するオートチューニング機能を提供しています。 オートチューニング機能を使うことで、限られた時間内、条件下で最高のパラメータを探索し、予測モデルを生成することができます。 今回やること 今回はオートチューニングを使って数値予測モデルを生成します。 使うデータは架空の銀行の金融商品販売データです。顧客の取引履歴と営業履歴から構成されており、新たな金融商品の販売数を予測するデータとなっています。 内容は以下のようになっており、約5万行、22列の構成です。 1行1お客様データとなっていて、顧客の口座情報や取引履歴、営業履歴が1行に収納されています。 ターゲット変数はcount_tgtで、これは各顧客が購入した金融商品数を表しています。 ほとんどが0(=未購入)ですが、購入されている顧客の購入数を予測するモデルを生成します。 今回はランダムフォレストを使って予測したいと思います。 ランダムフォレストは別々の決定木を複数作り、各決定木の予測値をアンサンブルして最終的な予測値とする機械学習の一種です。 まずは手動で予測 SAS Viyaでランダムフォレストを使って予測モデルを生成するにあたり、まずはCASセッションを作ってトレーニングデータとテストデータをインメモリにロードします。 # PythonからCASを操作するためのSWATライブラリをインポート import swat # 接続先ホスト名、ポート番号、ユーザー名、パスワードを指定 host = "localhost" port = 5570 user = "cas" password = "p@ssw0rd" # mysessionという名称のCASセッションを作成 mysession = swat.CAS(host, port, user, password)
機械学習で予測モデルを作るとき、課題のひとつにパラメータのチューニングがあります。 パラメータとはどういう設定値や制限値で機械学習の予測モデルを作るのかを示すものです。 料理に例えると、チャーハンを作る過程が機械学習のアルゴリズムだとすると、どういう具材をどのくらいの量入れるのかがパラメータです。 お米の品種や卵の有無、豚肉か鶏肉か、調味料の種類や量がパラメータになります。チャーハンの良し悪しはこれらパラメータの良し悪しに左右されます。おいしいチャーハンを食べるためには、具材をベストな組み合わせと量で投入する必要があります。 昼食においしいチャーハンを食べたので、チャーハンでたとえました。 話を戻すと、機械学習の決定木の深さであったり、ニューラルネットワークのニューロン数であったり、パラメータは自分で設定する必要があります。機械学習では複数のパラメータを組み合わせて、ベストなレシピを作らねば良い予測モデルは作れません。 SAS Viyaでは各種機械学習アルゴリズムを提供していますが、各機械学習にそれぞれのパラメータが用意されています。料理に例えると、メニューにチャーハンのみならず餃子、ラーメン、寿司、ステーキ、チーズケーキがあるようなものです。シェフ(≒データサイエンティスト)は全てのベストなレシピ(≒パラメータ)を探索せねばならず、労力がいります。 しかし! SAS Viyaには更に便利な機能として、オートチューニングというものが用意されています。 オートチューニングは最も良いパラメータを短い時間で探索してくれる機能です。料理に例えると、究極のチャーハンレシピをViyaが自動的に作ってくれる機能です。夢のようですね。 オートチューニングでは機械学習のパラメータを変えながら複数の予測モデルを作り、最も良い予測モデルのパラメータを探してくれるというものです。決定木だけでもパラメータは10種類以上あるのですが、それらの最良な値をみつけてくれます。 パラメータチューニングを行う際、最も安易な探索方法は各パラメータの全パターンを試すことです。全パターンを試せば、その中から最も良いものはたしかにみつかります。しかし欠点はパラメータチューニングに長い時間がかかってしまい、現実的な手法ではありません。 SAS Viyaのオートチューニングはより賢いパラメータ探索のアルゴリズムを4種類用意しています。 遺伝的アルゴリズム(Genetic Algorithm, GA):パラメータを遺伝子と見立てて、淘汰、交叉、突然変異を組み換えすことでパラメータを探索する。 ラテン超方格サンプリング(Latin HyperCube Sampling, LHS):層別サンプリングの一種で、各パラメータをn個の区間に分割し、区間からランダムに値を取り出してパラメータを探索する。 ベイズ最適化(Bayesian Optimization):説明変数と予測の間にブラックボックス関数があると仮定し、ブラックボックス関数のパラメータの分布を探索する。 ランダムサンプリング(Random Sampling):ランダムにパラメータの値を選択して探索する。 探索アルゴリズムを詳しく説明していると終わらないので説明を短くまとめました。SAS Viyaではいずれかのアルゴリズムを利用してオートチューニングを実行することができます。 今回はPythonからSAS Viyaを操作して、オートチューニングを試してみたいと思います。 まずはPython SWATをimportし、CAS Sessionを生成してデータをロードします。 # PythonからCASを操作するためのSWATライブラリをインポート import swat # mysessionという名称のCASセッションを作成 mysession = swat.CAS(host, port, user, password) #
SAS Viya deployments use credentials for accessing databases and other third-party products that require authentication. In this blog post, I will look at how this sharing of credentials is implemented in SAS Environment Manager. In SAS Viya, domains are used to store the: Credentials required to access external data sources. Identities
In this blog post I am going to cover the example of importing data into SAS Viya using Cloud Analytic Services (CAS) actions via REST API. For example, you may want to import data into a CASLib via REST API. This means you can perform an import of data outside
The Cloud Analytic Server (CAS for short) is SAS’ latest high-performance, scalable, in-memory analytic data server. In this post, I’d like to discuss the CAS physical data model, i.e.what features CAS offers for data storage, and how to use them to maximize performance in CAS (and consequently SAS Visual Analytics