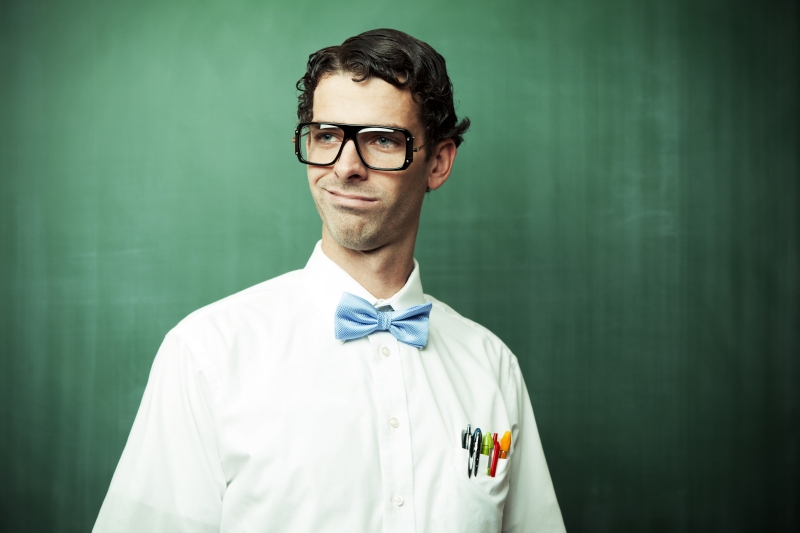
Model Governance ist nicht die neueste Daumenschraube der EZB, sondern eine Notwendigkeit mit Effizienzpotenzial - Warum Banken bei regulatorischen Anforderungen zum Abwarten neigen – und warum das kein nachhaltiges Vorgehen ist - , habe ich meinem letzten Blogpost beschrieben. Wie aber sieht denn ein sinnvolles Vorgehen aus? Die gute Nachricht