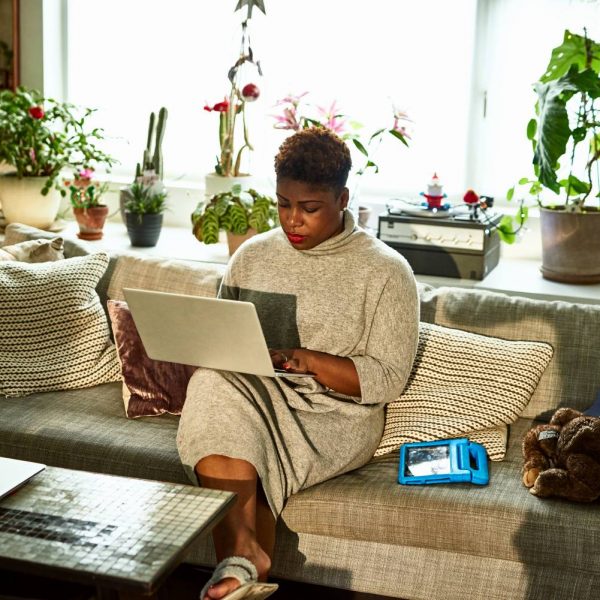
Every year hundreds of dedicated SAS® users and event volunteers work tirelessly to create an amazing conference experience for SAS Global Forum attendees. This year, as you know, COVID-19 changed our plans. But our devoted team of volunteers, including our conference chairs and session presenters, was still determined to provide