[Jessica Curtis and Adam Hillman, both Forecasting Advisors at SAS, were co-authors of this post]
The world has been dramatically impacted by the recent COVID-19 pandemic. Many of us are juggling a completely new lifestyle that was forced upon us overnight. As consumers find their way to a new normal, retailers are experiencing upheaval like never before. In a matter of days, retailers went from thinking they may need to close some locations to closing all stores, and even halting e-commerce due to the challenge of maintaining a safe and healthy work environment.
The retail apparel and fashion industry are very different from the consumer-packaged goods (CPG) industry. CPG companies are trying to quickly react to dramatic increases in demand as consumers are over-purchasing in panic, while managing with inventory shortages. The retail apparel and fashion world are experiencing the complete opposite. Their retail locations have closed and demand has dropped to zero, or close to it. Inventory is piling up. Retailers are trying to cancel orders. Merchandise planners are trying to plan for the next season, and they don't even know yet when certain locations will be open.
SAS has advanced forecasting capabilities that leverage advanced artificial intelligence and machine learning techniques to capture and model the most likely outcome of demand for retailers. Whether the COVID-19 pandemic, or any other pandemic, demand patterns are changing across four distinct phases: preliminary, outbreak, stabilization, and recovery. These phases encompass two key calls to action for retailers: respond and recover.
Within each phase, the impacts to retail demand and the actions retailers can take tend to be very different. We're going to describe each phase, the impact to retail, and how retailers can leverage the power of SAS forecasting to react and quickly pivot in times of uncertainty.
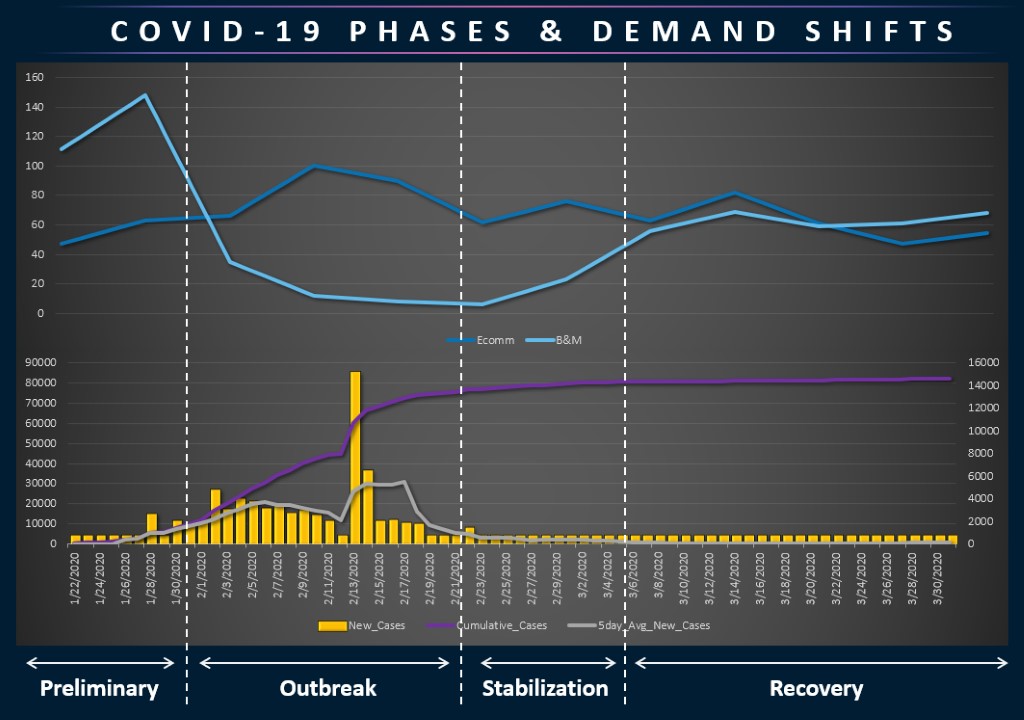
Respond: Preliminary & outbreak phases
A pandemic begins with the preliminary phase. In this phase, it's business as usual, with no government mandates and fairly typical consumer behaviors. Retailers may see a slight increase in core essential products as a small percentage of society will begin to ‘stock up’ on core essential items for hypermarket retailers. Most retailers will not see an impact to demand.
With business as usual, SAS forecasting utilizes trends, seasonality and cyclical patterns as well as causal factors such as promotions and in-store events to drive a statistically based merchandising process. SAS also utilizes advanced machine learning techniques, along with location and merchandise attributes, to intelligently develop sales forecasts for new items or items with little to no historical demand.
Merchandise planners can utilize the statistical forecast to plan overall sales within the merchandise financial planning process. Buyers can utilize this demand signal to plan core assortments as well as fashion assortments. The SAS forecast can also automatically integrate into current replenishment and allocation solutions to intelligently drive inventory management decisions. Each week, as sales actualize and new data is available, the SAS forecasting engine will re-forecast demand, taking into account recent trends. As the preliminary phase moves into the next phase, this becomes an important capability. Advanced forecasting models can begin to pick up trends and learn from them, driving better insights and quick responses for retailers.
Then the outbreak phase quickly follows. As the virus rapidly spreads geographically, travel restrictions are enforced and government mandates are rolling out regionally. This is where the challenges for retailers begin. Consumer demand begins to depress, retail locations begin to close due to regional stay-at-home orders, and eventually, this leads to company-wide store closures.
As these events occur, retailers are challenged with understanding how to plan for this rapid decline in demand. One of the ways that SAS can help is by leveraging an analytical forecast modifier. This approach intelligently modifies a current forecast. This current forecast could be a SAS statistical forecast, an open source forecast, a naïve forecast or even a working plan value from excel. An analytically derived adjustment for the forecasts is used as a temporary intervention during the outbreak period to update and adjust merchandise plans, assortment plans as well as allocation and replenishment decisions.
This analytically derived adjustment utilizes external data such as COVID-19 statistics. There have been a plethora of free data sets available from many different public government agencies, such as the World Health Organization (WHO) and Centers for Disease Control (CDC). There are also programs available on GitHub that provide data and scripts that automate the refresh of this data.
Recover: Stabilization & recovery phases
As the number of new disease cases begins to level off, the pandemic moves into a stabilization and recovery phase. The stabilization phase is characterized by governments gradually removing restrictions across regions, stores beginning to re-open, and consumers no longer panic buying. Retailers begin to see an increase in in-store demand, and a semblance of a new normal on the horizon. In this phase, the challenge for retailers becomes how to analytically capture the impacts of the pandemic on demand historically, while building realistic merchandise and inventory plans going forward. As demand starts stabilizing, that’s when event modeling across the different phases of the pandemic, and incorporating causal variables into forecasts, are critical to ensuring valuable demand forecasts in the future.
SAS forecasting offers flexible and robust event modeling capabilities, with the ability to model each phase of the pandemic and apply specific event types to each. SAS automatically determines the impact of each phase of the event across the entire product portfolio, quantifying the lift or decline at a granular level. Retailers can leverage past event data from natural disasters such as hurricanes, along with demand data from markets that were hit early in the pandemic, such as China, as proxies for the expected pattern of demand modeled into an event. From there, SAS enables retailers to run what-if scenarios on the next phases of the pandemic – including the event duration and recurrence in the future – to understand best- and worst-case outcomes proactively. This framework empowers retailers to be prepared if there are subsequent waves of the pandemic.
Improved forecasting during the stabilization phase also includes incorporating both internal and external causal variables. Internal data includes sales and inventory history to capture demand shifts across time, pantry loading effects, and long-term product mix changes. Product and location attributes can be leveraged to understand changing consumer preferences both during and after the event. Understanding website traffic along with store closure and re-open dates regionally is important for capturing the shifts in demand across channels. SAS can help retailers incorporate external variables as well, including historical COVID-19 statistics, future COVID-19 projections, and government restriction dates. Social media data can be harnessed through SAS text analytics to understand sentiments and plan for the recovery period. Economic indicators, such an unemployment rates, and market trends also provide a perspective on the long-term impacts of this disruptive event.
During the stabilization phase, SAS improves forecasting decisions through not only traditional time series techniques, but also a breadth of machine learning algorithms. These machine learning algorithms assess demand shifts at the most granular levels, and automatically learn, adapt, and improve over time as new demand data is available. The combination of time series and machine learning algorithms provides superior forecasting results to time series methodologies alone4, leading to improved inventory productivity and consumer centric decisions across both merchandising and supply chain.
The stabilization phase is still categorized by uncertainty of what the future holds. Therefore, it's imperative for retailers to have an agile, iterative forecasting process for capturing new data as the situation changes from day to day. SAS automates the forecasts and enables a process to quickly react as new information comes in, with new models, multiple scenarios, collaborative decisions, and immediate impact to downstream decision-making. This positions retailers on a path to recovery, and ultimately, to long term growth.
As the number of cases continues to stabilize and most businesses are now open, the pandemic moves into the recovery phase. In the recovery phase, governments have generally removed restrictions across most regions and retailers are returning to near 100% operation. While social distancing rules and regulations may still be in place, companies now have the confidence to plan their business without major constraints.
In the recovery phase, the need for accurate machine learning statistical forecasts to drive business decisions is essential. As models were improved in the stabilization phase with additional causal variables and events, reviewing and re-tuning models to improve forecast accuracy is now the core objective.
Incorporating new information into machine learning models allows users to understand what variables will be useful in explaining recent demand shifts due to the COVID-19 virus. SAS’ suite of visual analytics serves as a foundation for evaluating large data sets and quickly gain insights. Evaluating forecast models may involve understanding the significance of casual variables such as COVID-19 cases, unemployment data, store traffic, and other useful information that was introduced in the stabilization phase. In addition to understanding the significance of these casual variables, testing of models through the use of holdout samples is prudent. The re-tuning of forecast models will maximize success for retailers, as demand patterns will continue to be volatile.
Following multiple iterations of evaluation and re-tuning of models, confidence in forecast models has now increased dramatically. Long lead time forecasts for 2021 that anniversary the COVID-19 epidemic should be reviewed to reality check statistical forecasts and determine if additional information should be considered.
Reimagine: Prepare for the future
Throughout each phase of a pandemic, SAS statistical forecasting is a key driver to understanding and evaluating the impact to overall demand and consumer behavior. Leveraging both internal and external data, advanced modeling techniques can be utilized to identify and quantify the significance and impact to demand across products, locations, and channels. This demand signal can then be consumed throughout the organization to drive merchandise financial planning, assortment planning, allocation and replenishment strategies. SAS statistical forecasting helps to ensure retailers are maximizing profitability while effectively optimizing inventory across all phases of the pandemic. Ultimately, this builds resilience in the go-forward forecasting processes and prepares retailers for future success.
Find more information on the SAS Retail & CPG COVID-19 resources site.