Discover how AI is used today and how it will augment human experience in the future
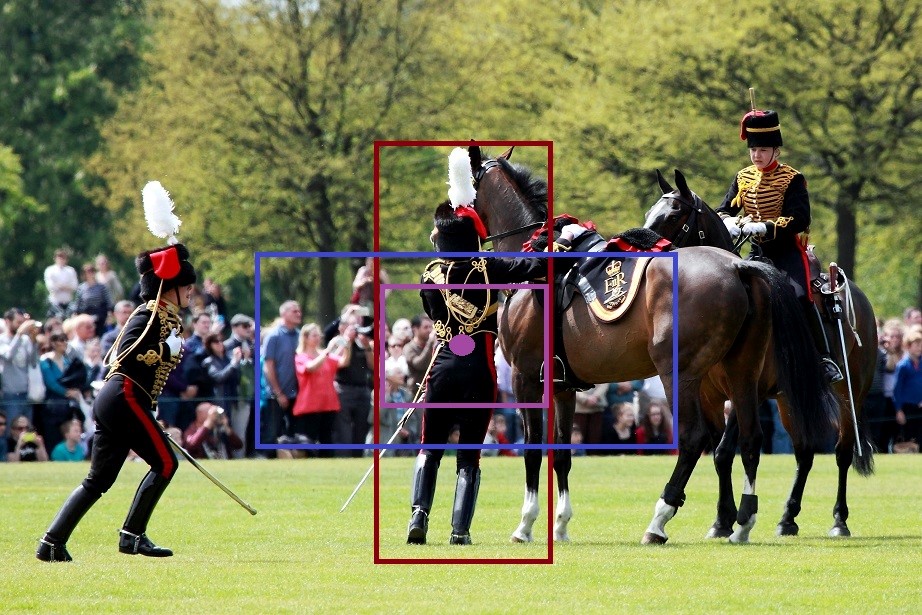
この記事はSAS Institute Japanが翻訳および編集したもので、もともとはXindian Longによって執筆されました。元記事はこちらです(英語)。 物体検出とは? 物体検出とはコンピューター・ビジョンの一分野であり、画像内に含まれる関心対象の物体を自動的に背景から区別して位置特定する手法です。例えば、図1に示す2つの画像では、いずれも前景に物体があります。左の画像では鳥が、右の画像では犬と人間が前景にあります。 「物体検出問題の解決」とは、「これらの物体をぴったりと囲むバウンディング・ボックスを配置し、それぞれのバウンディング・ボックスに対して正しい物体カテゴリーを関連付けること」を意味します。画像処理の領域における他のタスクと同様、物体検出を実行するときにもディープ・ラーニングは最先端の手法として力を発揮します。 物体検出の仕組み 物体検出に関する重要な問題の1つは、前景にある物体の数が画像によって様々に異なる、ということです。しかし、ここでは物体検出の仕組みを理解するために、まずは1つの画像に1個の物体しか存在しないと仮定し、この制約条件の下で物体検出問題を考えてみましょう。1つの画像に1個の物体しか存在しない場合、バウンディング・ボックスの発見と物体のカテゴリー判断という問題は、単純明快な方法で解決することができます。バウンディング・ボックスは4組の数値で表現されますから、バウンディング・ボックスの位置を学習するタスクは、回帰問題として無理なくモデル化することが可能です。そのタスクが済めば、物体のカテゴリー判断は分類問題として解くことができます。 ここでの「制約条件付きの物体検出」という課題に関する回帰および分類問題に対する解法を提供するのは、図2に示す畳み込みニューラル・ネットワーク(CNN)です。コンピューター・ビジョンの領域における他の従来型タスク(例:画像認識、キーポイント検出、セマンティック・セグメンテーションなど)の場合と同様、ここでの「制約条件付きの物体検出」という課題では、固定数のターゲットを扱います。これらのターゲットの当てはめは、固定数の分類または回帰問題としてターゲットをモデル化することによって実行可能です。 前述のとおり、真の物体検出はN個の物体を処理できなければなりません(Nの値は画像によって異なります)。残念ながら、図2に示したCNNは、このような、より一般的な問題を解決することができません。しかし、多くの矩形ボックスの位置とサイズについて仮説を立てることによってCNNの変種を使用し、CNNを物体の分類にのみ利用する、というやり方は可能かもしれません。私たちはそのような場合の矩形ボックスを、しばしば「ウィンドウ」と呼びます。ウィンドウ仮説を汎用的なものにするためには、画像内で考えられる全ての位置とサイズをカバーしなければなりません。それができれば、それぞれのサイズと位置のウィンドウについて、「その中に物体が存在するかどうか?」と、「存在する場合、物体のカテゴリーは何か?」を判断することが可能になります。 図3は、このアプローチで物体検出を実現する場合に候補となりうるウィンドウをいくつか示しています。画像は有限個のピクセルで構成されていますから、ウィンドウの総数は膨大です。検討すべきウィンドウの数の膨大さを考えると、このアプローチはコンピューティングの観点からは非実用的です。 ウィンドウを用いて物体を探すための効率的な手法 では、「一部のウィンドウだけを調べる」というスマートな方法で物体検出を実行することは可能でしょうか? 答えは「イエス」です。このような「ウィンドウの部分集合」を発見する方法には2つのアプローチがあり、それらは2つの異なる物体検出アルゴリズムへとつながります。 第1のカテゴリーのアルゴリズムは、最初にリージョン・プロポーザル(領域候補の抽出)を実行します。これは具体的には、コンピューター・ビジョンの従来の手法(選択的検索など)を用いて、あるいは、ディープ・ラーニングに基づくリージョン・プロポーザル・ネットワーク(region proposal network: RPN)を用いて、物体を含んでいる可能性の高い領域を選択する、ということです。候補ウィンドウの少数のセットを収集したら、セットの数だけ回帰モデルおよび分類モデルを定式化することによって、物体検出問題を解決することができます。このカテゴリーに属するアルゴリズムとしては、Faster R-CNN[1]、R_FCN[2]、FPN-FRCN[3] などがあります。このカテゴリーのアルゴリズムは、通常、「2段階法」と呼ばれます。これらは一般に、この後に紹介する「1段階法」に比べ、正確性は優れていますが、処理は低速です。 第2のカテゴリーのアルゴリズムは、固定位置にある固定サイズの物体だけを探します。これらの位置とサイズは、ほとんどのシナリオがカバーされるように戦略的に選択されます。通常、これらのアルゴリズムは、元の画像を固定サイズのグリッド(格子)領域に分割した上で、それぞれのグリッド領域に関して、あらかじめ決めておいた所定の形状およびサイズの、固定数の物体を予測することを試みます。このカテゴリーに属するアルゴリズムは「1段階法」と呼ばれます。この手法の例としては、YOLO[4]、SSD[5]、RetinaNet[6]などが挙げられます。このカテゴリーのアルゴリズムは、通常、より高速に実行できますが、正確性は劣ります。このタイプのアルゴリズムは、リアルタイム検出を必要とするアプリケーションで活用されることが多くなっています。 以下では、これらのうち2つの一般的な物体検出手法を取り上げ、もう少し詳しく検討します。 YOLOによる物体検出 YOLO (You Only Look Once) は、1段階の物体検出手法を用いる代表的なアルゴリズムです。このアルゴリズムが物体を検出するためにたどるステップを、図4とその下の箇条書きに示します。 元の画像を等サイズのグリッドに分割します。 それぞれのグリッドに関して、事前に定義した形状の、グリッドの中心を中心位置とするバウンディング・ボックスを、事前に設定した数だけ予測します。それぞれの予測には、クラス確率と物体信頼度(その領域が物体を含んでいるか、あるいは背景のみか)が関連付けられます。 最後に、高い物体信頼度およびクラス確率が関連付けられたバウンディング・ボックスを選択します。最も高いクラス確率を持つ物体クラスが、その物体のカテゴリーとなります。 事前に定義した形状の事前に設定した数のバウンディング・ボックスは「アンカーボックス」と呼ばれ、k平均法アルゴリズムによってデータから取得されます。アンカーボックスは、物体のサイズと形状についてデータセットから予備知識を捕捉します。異なるサイズおよび形状の物体を検出するためには、異なるアンカーが設計されます。例えば図5では、1つの場所に3種類のアンカーが表示されていますが、最終的には赤のアンカーボックスが中央の人物を検出します。言い換えると、アルゴリズムは、物体とこのアンカーボックスの適切なサイズを一緒に検出します。通常、最終的な予測は、アンカーの位置またはサイズ自体とは異なります。なぜなら、画像の特徴量マップから取得される最適化されたオフセット値が、アンカーの位置またはサイズに加算されるからです。 YOLOアルゴリズムのアーキテクチャを図6に示します。検出層は、多数の回帰および分類オプティマイザーを含んでおり、その数はアンカーの数によって決まります。 Faster RCNNによる物体検出 Faster RCNN[1] は、2段階の物体検出アルゴリズムです。図7は、Faster RCNNの2つの段階を示しています。アルゴリズム名に “Faster” と付いていますが、「1段階法よりも高速」という意味ではありません。この名称は歴史的な経緯を反映しており、以前のバージョン(オリジナルのRCNNアルゴリズム[7] やその後継のFast RCNN[8])よりも高速であることを示しています。Faster RCNNにおける高速化は、個々の関心領域(Region of Interest: RoI)に関する特徴抽出計算を共有する手法と、ディープ・ラーニングに基づくリージョン・プロポーザル・ネットワーク(RPN)の導入によって実現されています。 多数のCNN層を用いて特徴量マップを抽出した後、リージョン・プロポーザル・ネットワーク(RPN)が、物体を含んでいる可能性の高い多数のウィンドウを出力します。その後、アルゴリズムは、各ウィンドウ内部の特徴量マップを取得し、それらを固定サイズにリサイズ(またはポール)することで(=RoIプーリング)、物体のクラス確率とより正確なバウンディング・ボックスを予測します。