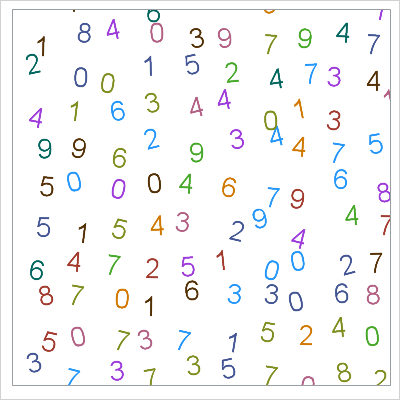
Are you still using the old RANUNI, RANNOR, RANBIN, and other "RANXXX" functions to generate random numbers in SAS? If so, here are six reasons why you should switch from these older (1970s) algorithms to the newer (late 1990s) Mersenne-Twister algorithm, which is implemented in the RAND function. The newer