All Posts
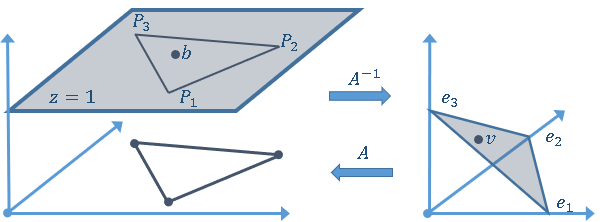
A colleague asked how to compute the barycentric coordinates of a point inside a triangle. Given a triangle in the plane with vertices p1, p2, and p3, every point in the triangle can be represented as a convex combination of the vertices: c1*p1 + c2*p2 + c3*p3, where c1,c2,c3 ≥
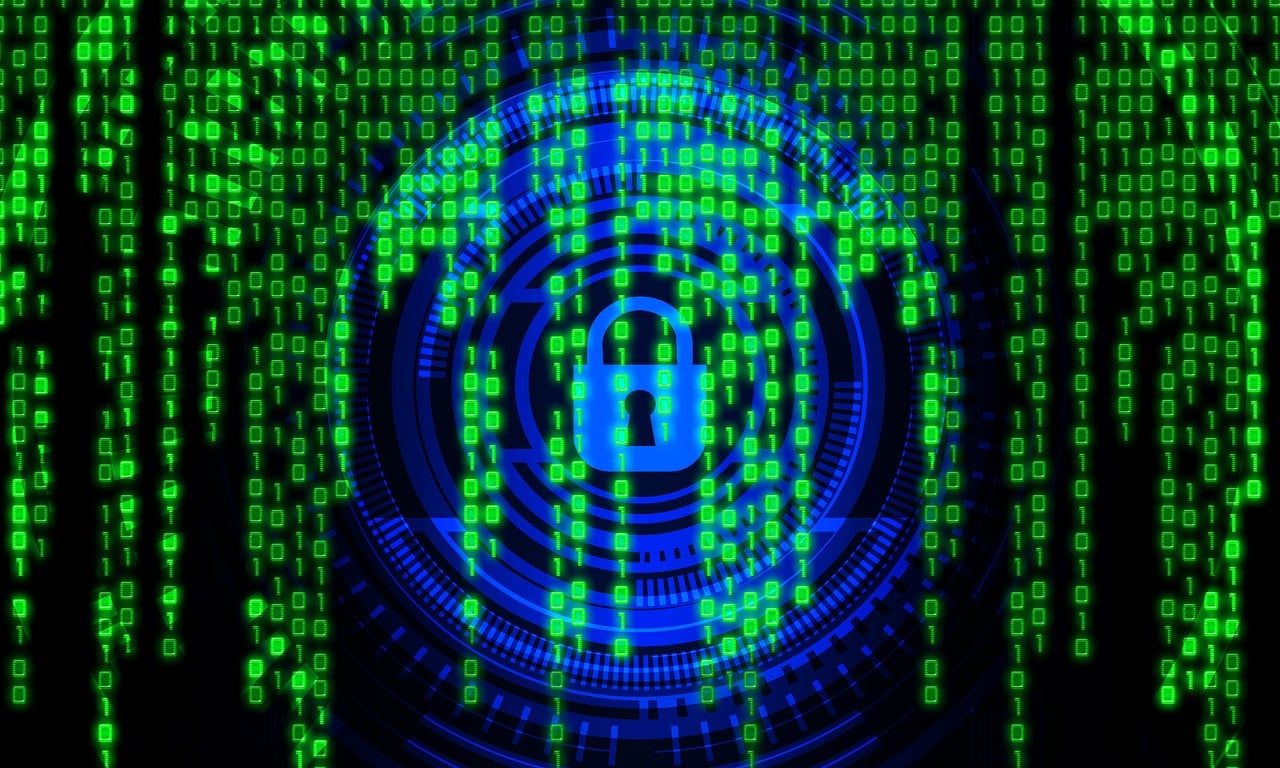
SAS' Phuong Ngo demonstrates an automated shift-left CI/CD security workflow.
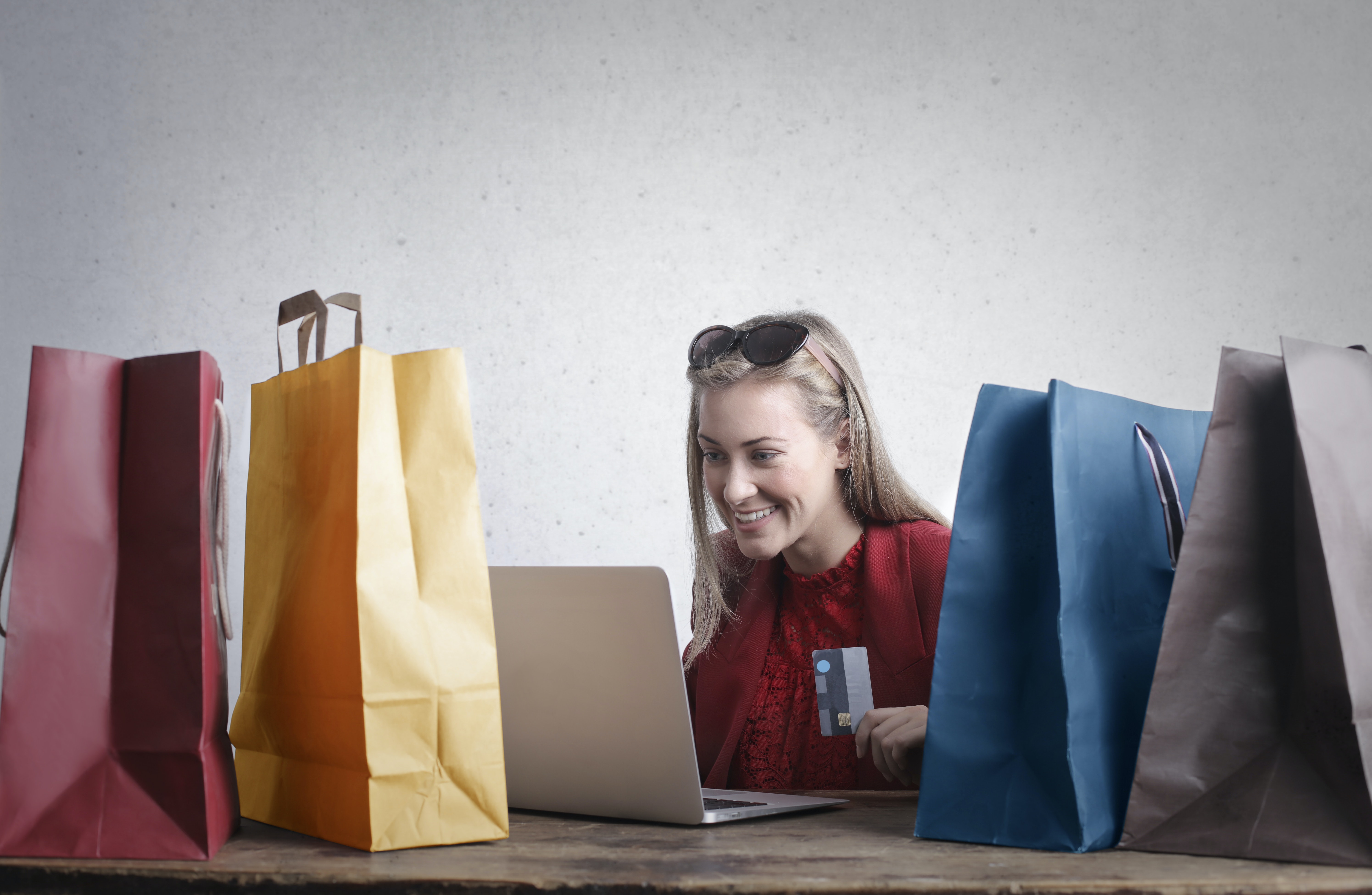
Las temporadas altas de ofertas como el hot sale representan una gran oportunidad tanto para las compañías como para los clientes, pero también para los defraudadores. De acuerdo con SAS, firma pionera y especializada en analítica avanzada, IA y gestión de datos, a medida que aumenta la cantidad de transacciones
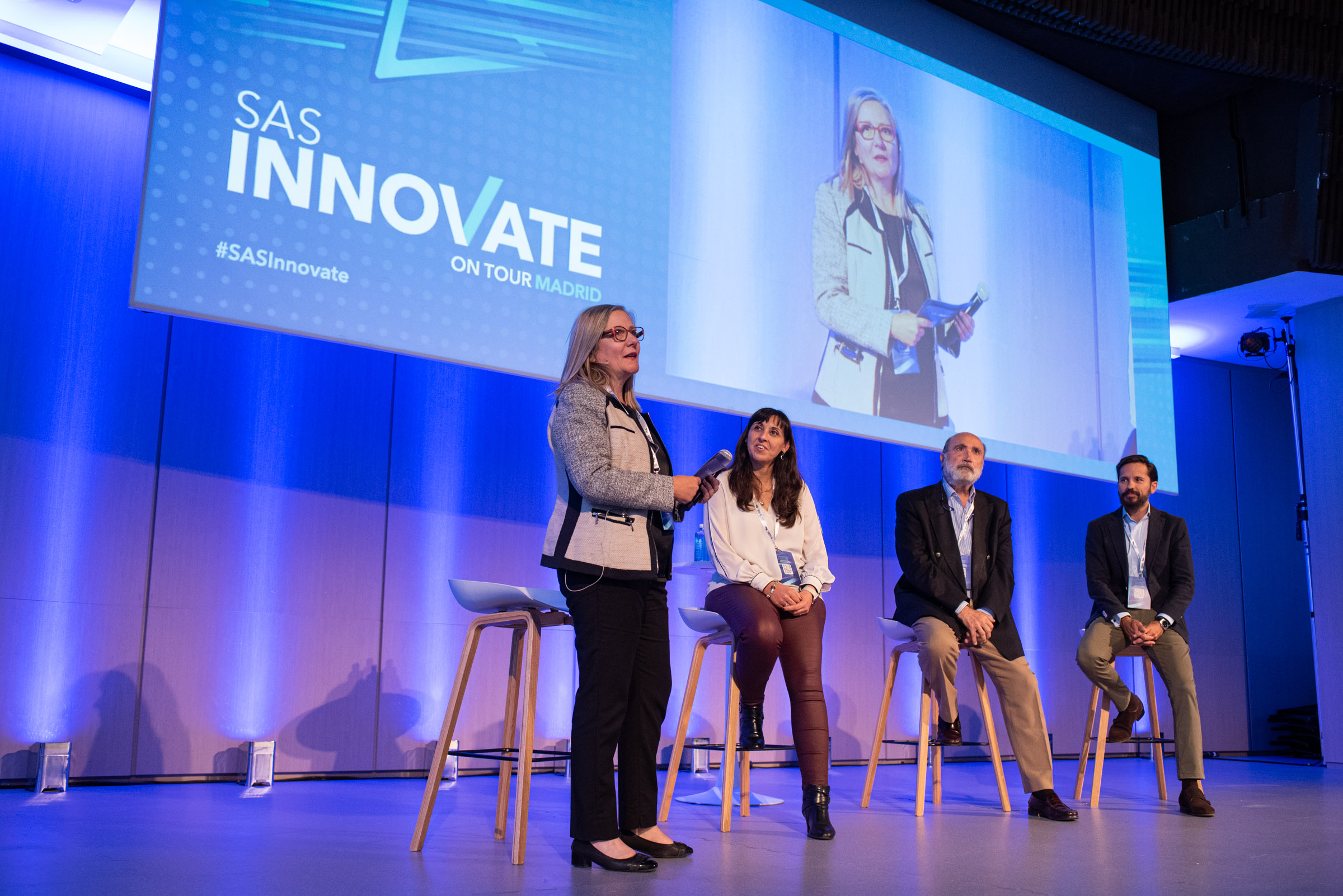
Había mucha expectación en torno a la celebración del SAS Innovate Madrid, que tuvo lugar el pasado 25 de mayo. Escuchamos muchas conversaciones sobre lo que la gente esperaba del evento. Estas incluían el deseo de volver a encontrarse cara a cara con clientes, partners y colegas del sector para
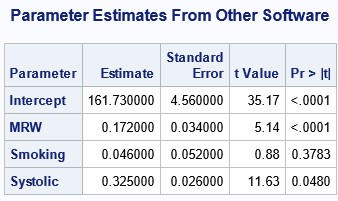
Part of the power of the SAS ODS system is the ability to visualize data by using ODS templates. An ODS template describes how to render data as a table or as a graph. A lot of papers and documentation have been written about how to define a custom template
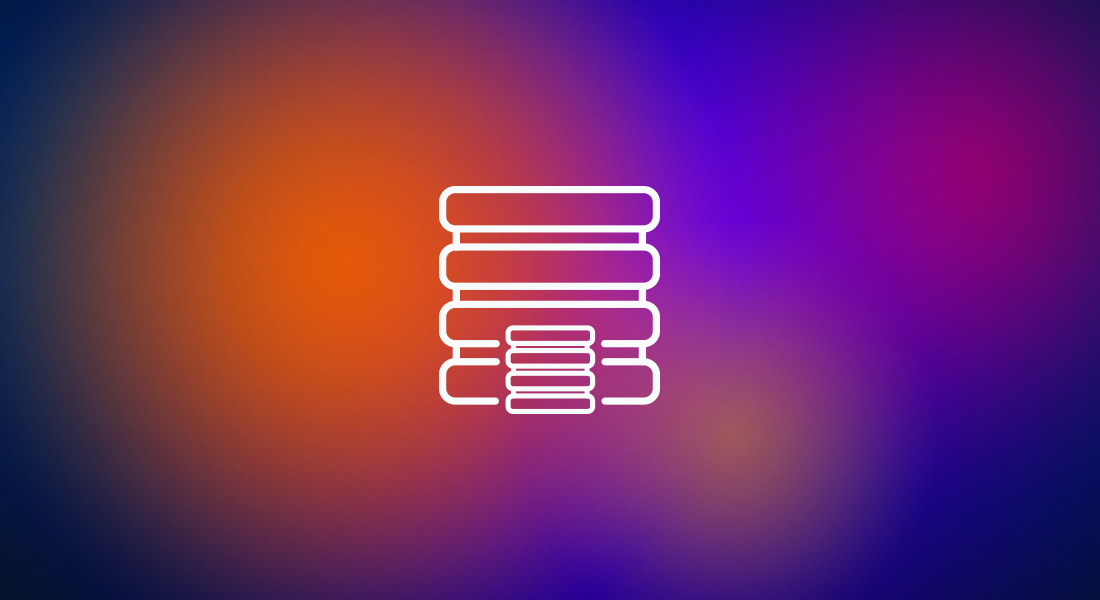
データサイエンスの使いどころ・・・攻めと守りの圧倒的な違い 以前のブログで、データ活用における攻めと守りについてお話しました。今回は小売業を例に多くのデータ活用プロジェクトが陥りやすい罠と、真の目的達成のための方法についてご紹介します。 小売業の目的はもちろん他の業種企業と変わらず、収益の最大化です。昨今データ分析を武器として売り上げの最大化、コストの削減、業務プロセスの生産性向上を目指す企業が増えてきています。時には、データサイエンティストが、データサイエンスを駆使してプロジェクトを実行しているケースもあるでしょう。 ここで、今一度現在取り組んでいる、またはこれから取り組もうとしているデータサイエンスやAI活用のプロジェクトがどんな利益を自社にもたらすのかを改めて考えてみましょう。昨今、需要予測についての相談が非常に多いので、ここでは需要予測について考えてみます。 弊社にご相談いただくケースの中で、少なくない企業が、需要予測をこのブログで言うところの「守りの意思決定」としてとらえています。多くのケースで、過去の実績をベースに将来の需要を予測することで、在庫過多や欠品を減らそうというプロジェクトに投資をしていたり、しようとしています。言い換えると、過去の実績を学習データとして、将来を予測するモデルを構築し、ひとつの将来の需要予測を作成し、それを在庫を加味したうえで、発注につなげています。 手段が目的化することで見失う可能性のある本来の目的とは 非常に典型的なAI活用、データサイエンス活用かと思いますが、実は、「AIで予測」、「機械学習で予測」といった言葉で最新のデータ活用をしているかのような錯覚に陥っているケースが見受けられます。数十年前から行われており、昨今でも同様に行われている、機械学習を用いた典型的な需要予測は、「守り」です。すなわち、どんなに多くの種類のデータを使うかどうかにかかわらず、過去の傾向が未来も続くという前提のもとに予測モデルを作成している場合には、あらかじめ定義した前提・業務プロセスの制約の下で、機会損失を最小化するために予測精度をあげているにすぎません。 つまり、そのような前提での需要予測は、小売業の収益向上という観点では、期待効果が限定的であるということです。では、最終的な収益の最大化を実現するには、何をすべきでしょうか? 収益を向上させるためにはもちろんより多くの商品を売ることにほかなりません。より多くの商品を売るためには当然、顧客の購買心理における購買機会に対して販売を最大化する必要があります。あるいは、顧客の購買心理そのものを潜在的なものから顕在化したものにすることも必要でしょう。つまり、販売機会を最大限に活用するということは、店舗中心ではなく、顧客中心に考えるということです。 小売業における攻めのデータ活用の1つは、品ぞろえの最適化 このように、顧客中心に考えることで初めて最適な品揃えの仮説検証のサイクルが可能となります。過去のデータは、単に過去の企業活動の結果であり、世の中の「真理~ここでは顧客の本当の購買思考」を表しているわけではありません。真理への到達は、仮説検証ベースの実験によってのみ可能になります。わかりやすく言うと皆さんよくご存じのABテストです。このような実験により、品ぞろえを最適化することで、販売機会を最大化することが可能なります。そのプロセスと並行して、オペレーショナルな需要予測を実践していくことが重要となります。 需要予測と品ぞろえ最適化の進化 昨今、AIブーム、データサイエンティストブーム、人手不足や働き方改革といったトレンドの中で、従来データ活用に投資してこなかった小売業においても投資が進んでいます。しかし多くのケースでこれまで述べてきたような守りのデータ活用にとどまっていたり、古くから行われている方法や手法にとどまっているケースが見受けられます。歴史から学ぶことで、無用なPOCや効率の悪い投資を避けることができます。今、自社で行っていることがこの歴史の中でどこに位置しているかを考えてみることで、投資の効率性の向上に是非役立てていただければと思います。 小売業におけるデータ活用のROI最大化にむけたフレームワーク SASでは長年、小売業や消費財メーカのお客様とともにお客様のビジネスの課題解決に取り組んできました。その過程で、小売業・消費財メーカー企業内の個々の業務プロセスを個別最適するのではなく、それら個々の業務プロセスを統合した、エンタープライズな意思決定フレームワークが重要であるとの結論に至っています。AIやデータサイエンスという手段を活用し、データドリブンな意思決定のための投資対効果を最大化するための羅針盤としてご活用いただければと思います。
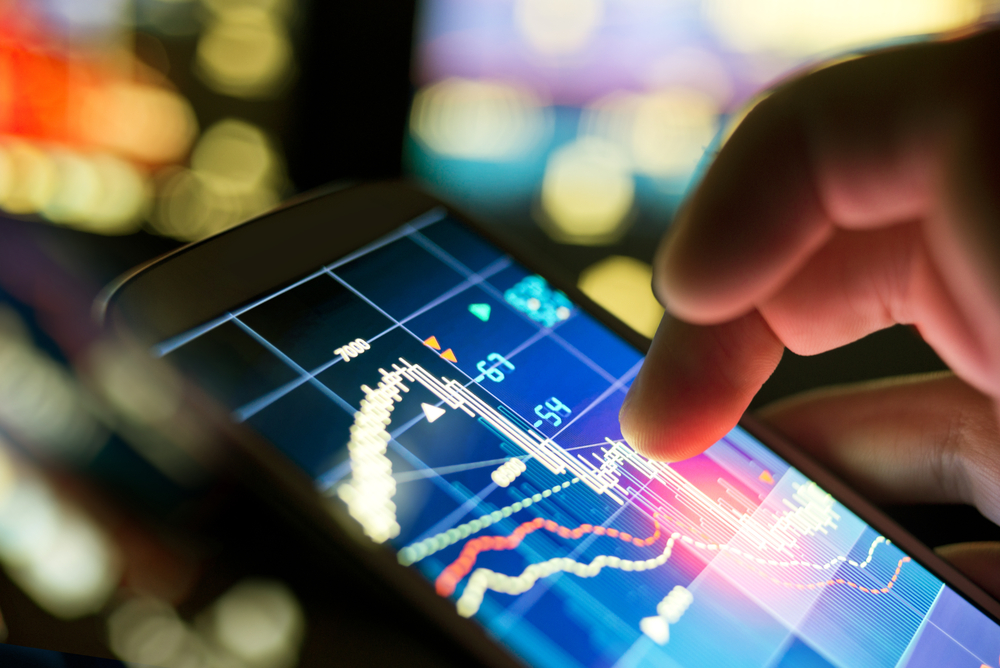
Muchas veces nos encontramos en nuestro día a día sin saber: ¿Cuál es conjunto óptimo de clientes objetivo de una campaña de marketing y la comunicación óptima a utilizar para cada cliente? ¿Cómo maximizar ingresos esperados y sus beneficios? ¿Cómo realizar una optimización y la evaluación equilibrada de cada tarea
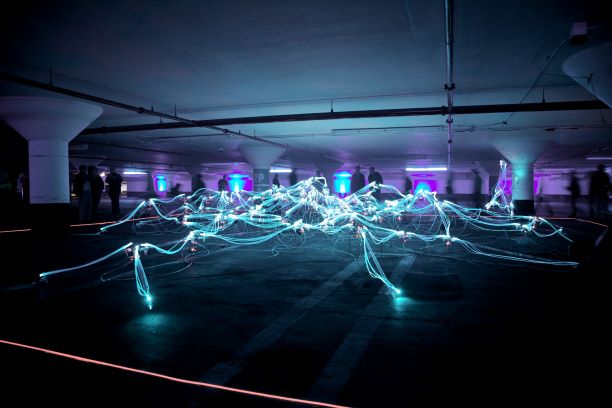
La visualización de redes se ha convertido en un básico para la detección e investigación de fraude, pero hay mucho más que obtener de la compleja estructura de gráficos que la respalda. En este sentido, y para comprender cómo podemos utilizarlas de manera efectiva para mejorar las tasas de detección,
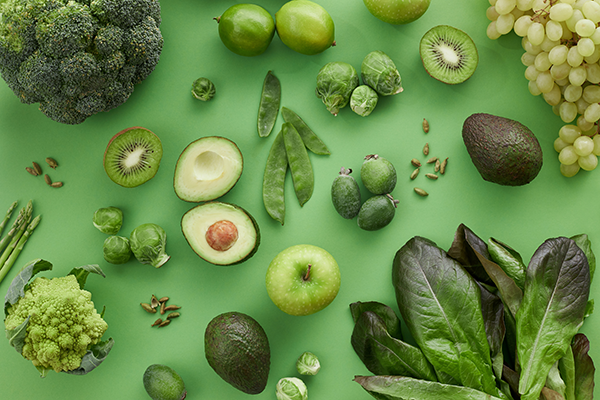
Each color of the rainbow – red, orange, yellow, green, blue and purple – provides a unique set of nutrients and health benefits that allow our bodies to function more optimally. As we continue to work our way through the rainbow in this occasional series, let’s go for green. Remember, our
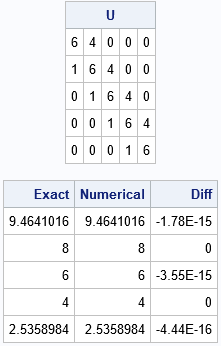
While writing an article about Toeplitz matrices, I saw an interesting fact about the eigenvalues of tridiagonal Toeplitz matrices on Nick Higham's blog. Recall that a Toeplitz matrix is a banded matrix that is constant along each diagonal. A tridiagonal Toeplitz matrix is zero except for the main diagonal, the
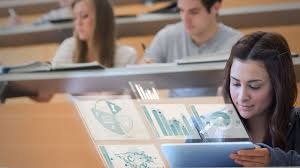
Del 17 al 28 de julio de 2023 SAS entrenará de forma gratuita a estudiantes de Latinoamérica y recién egresados para desarrollar las habilidades demandadas en el mercado laboral. El entrenamiento total tiene un valor aproximado de 7,500 dólares. Al finalizar los estudiantes se podrán certificar de forma gratuita. La
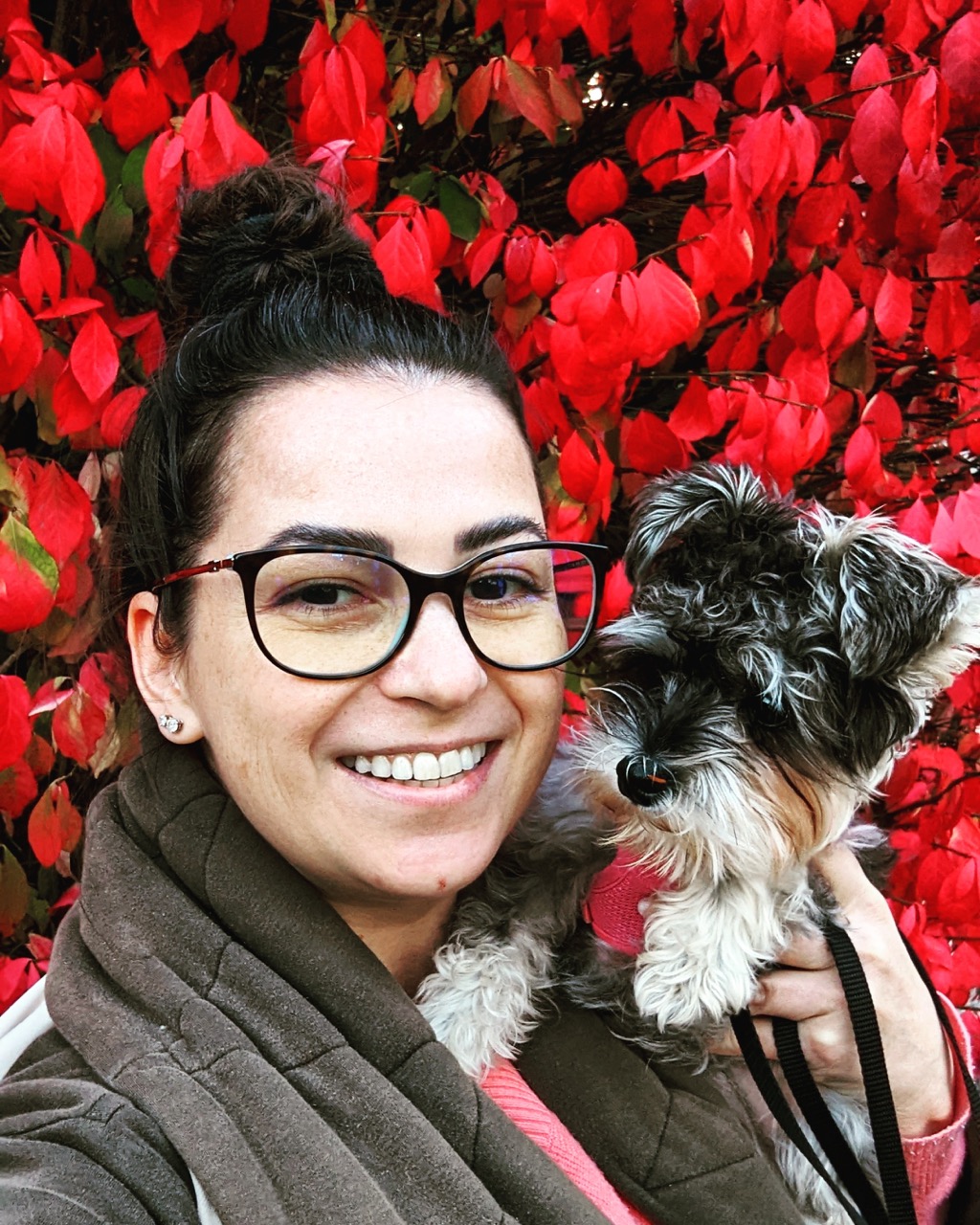
At SAS, where you start doesn’t have to be where you end; and there’s ample opportunity for internal career mobility. Milena Vukasovic has experienced this firsthand, and recently moved into a new role at SAS as a Sr. Associate Implementation Project Manager. Meet Milena Milena’s SAS story began at our
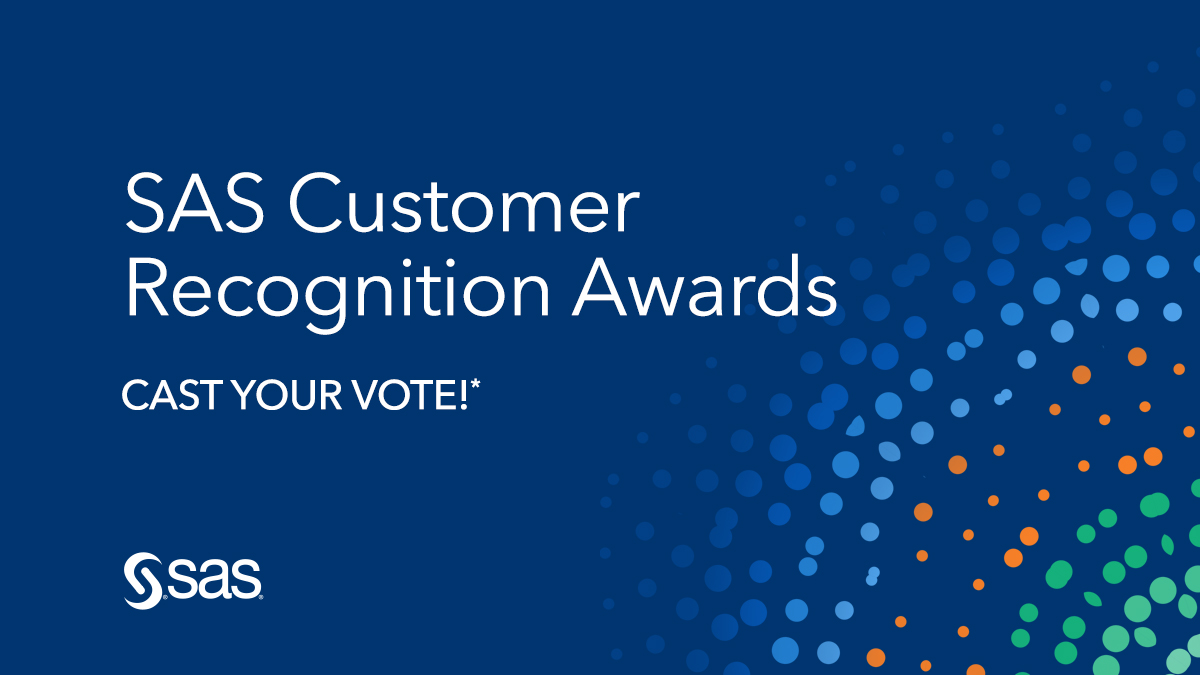
Entries are in. Now it’s your turn to cast your vote for the best in each category.
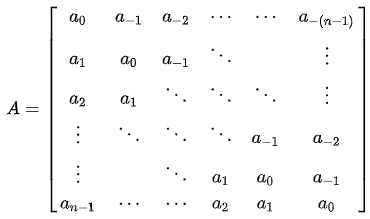
A Toeplitz matrix is a banded matrix. You can construct it by specifying the parameters that are constant along each diagonal, including sub- and super-diagonals. For a square N x N matrix, there is one main diagonal, N-1 sub-diagonals, and N-1 super-diagonals, for a total of 2N-1 parameters. In statistics and applied
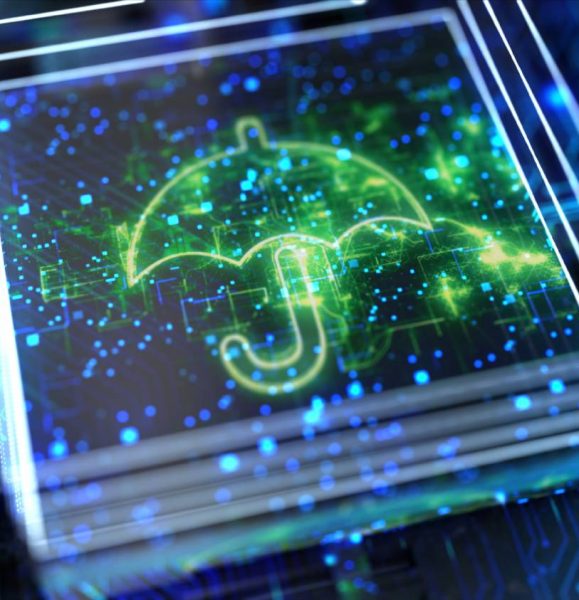
What if, 12 months ago, someone bet you that a technology was coming in the next year that would cause great excitement and great fear in all businesses, industries and geographies? Would you have taken that bet? Of course, now we know that the technology is generative AI. Popularized by