As organizations infuse trustworthy practices into the fabric of AI systems, remembering that trustworthiness should never be an afterthought is important.
Pursuing trustworthy AI is not a distant destination but an ongoing journey that raises questions at every turn. For that, we have meticulously built an ethical and reliable AI framework within this iterative process.
Our trustworthy AI approach begins by asking the right questions across five pivotal steps of the AI life cycle, each contributing to the goal of creating responsible and trustworthy AI. These steps – questioning, managing data, developing the models, deploying insights and decisioning – represent the stages where thoughtful consideration paves the way for an AI ecosystem that aligns with ethical and societal expectations.
In this blog post, we’ll dive into the first step: the questioning phase, where developers define the problem and chart the course of action. Let’s explore the trustworthy AI aspects that need to go into this step in detail.
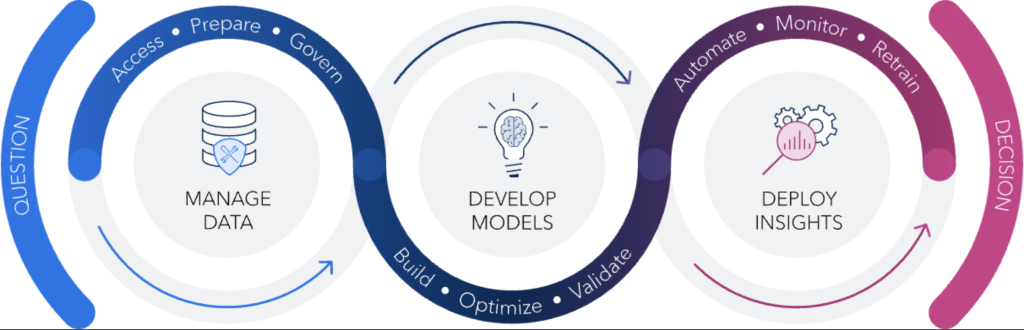
An introduction into the question phase of the AI life cycle
Let’s not get lost in technical jargon just yet. At its core, the question phase is simply about just asking the right questions. Who are we building this AI system for? What impact will it have on society? And perhaps most importantly, what could go wrong if we don’t get it right? We must answer these questions and more, including:
How do regulations impact the trajectory of AI development?
As AI adoption grows, so do the challenges. Governments worldwide are stepping up to propose and enact guidelines to balance competitiveness with trust, creating fair, ethical operations. Take the EU AI Act, for example, which was put in place to ensure better conditions for the development and use of AI. These regulations are like a GPS for AI developers, guiding them towards fair and ethical operation, safeguarding individuals and minimizing associated risks.
While designing the AI system, one should ask if the proposed AI system has been reviewed for compliance with applicable laws, regulations, standards and guidelines. However, it's not just about adhering to regulations during development; it's also about considering the life cycle of AI models. At this phase, we should contemplate how and when to decommission models and what to do with them. By integrating regulatory compliance considerations throughout the AI life cycle, organizations create a culture of responsible innovation that safeguards individuals and upholds ethical standards.
What measures can be taken to address ethical concerns?
Addressing ethical concerns in AI requires a comprehensive strategy focused on fairness, transparency and accountability. Without a clear understanding of how AI algorithms reach conclusions, there is a risk of perpetuating societal inequalities and eroding trust in their decisions.
Because of that, we need to make sure models are fair because this ensures equitable outcomes and safeguards against bias. Being transparent helps us get clear explanations of AI decision processes. This fosters trust and effectiveness.
Lastly, we need accountability mechanisms to map out defined responsibilities and consequences for unethical practices and reinforce ethical standards. Collaboration among stakeholders, including policymakers, data scientists and ethicists, is also crucial in addressing the concerns of AI use. This is especially true in sensitive areas like health care and law enforcement.
Who are the stakeholders, and what is their responsibility?
Identifying the diverse stakeholders involved in the AI system – ranging from business leaders, data engineers, ML engineers, data scientists, business analysts, model risk owners, domain experts, model owners and information technologists – is important.
But what are their roles and responsibilities when it comes to AI governance? Think of them as the driving force behind the scenes. They assess the big picture – weighing AI initiatives' potential benefits, risks and impacts. Technical teams and domain experts team up to ensure data quality, address potential biases and ensure compliance with regulations.
In short, it’s like a well-oiled machine, with everyone doing their part to ensure the AI system runs smoothly and ethically.
What is the role of feedback mechanisms in fortifying integrity and efficacy?
Feedback loops help AI systems to learn from experience, driving accuracy and fairness over time. These loops offer invaluable insights into AI decision making, ultimately leading to improved accuracy and fairness.
But it’s not just about making AI smarter – it’s also about building trust. These feedback loops shine a light on AI decision making processes, promoting transparency and accountability at every step. When users can report potential vulnerabilities, it shows organizations are serious about building long-lasting AI systems.
Considering these thoughtful integrations of feedback mechanisms in the question step strengthens AI capabilities and cultivates a culture of responsible innovation and continuous improvement.
Charting the future of AI development
Our journey through the AI lifecycle question phase underscores the interplay between regulatory compliance, ethical considerations, stakeholder engagement and feedback mechanisms. Also, adherence to governmental regulations ensures responsible innovation and fosters trust in AI technologies.
Addressing ethical concerns in AI decision making processes is important to uphold integrity and accountability. Identifying and engaging diverse stakeholders facilitates alignment with strategic objectives and ethical principles, driving the development of AI solutions that meet societal needs.
Furthermore, integrating feedback mechanisms creates continuous refinement and enhancement of AI systems, ensuring they remain responsive to evolving challenges and user needs.
Embracing these principles can help organizations confidently navigate the question phase, laying the groundwork for AI technologies' ethical, responsible, reliable and impactful deployment.