Find out how analytics, from data mining to cognitive computing, is changing the way we do business
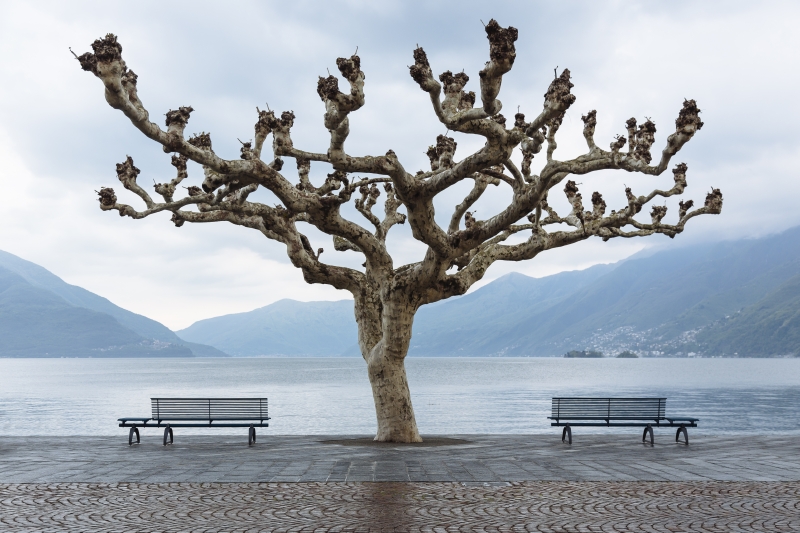
“It doesn’t stop being magic just because you know how it works.” Terry Pratchett, The Discworld Series Welcome to the third, and final, installment of Data Science in the Wild. In Part 1 we were lost in the woods thinking about how to start a data science project. In Part