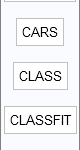
Sometimes I can't remember where I put things. If I lose my glasses or garden tools, I am out of luck. But when I can't remember where I put some data, I have SAS to help me find it. When I can remember the name of the data set, my
Sometimes I can't remember where I put things. If I lose my glasses or garden tools, I am out of luck. But when I can't remember where I put some data, I have SAS to help me find it. When I can remember the name of the data set, my
Every year near Halloween I write a trick-and-treat article in which I demonstrate a simple programming trick that is a real treat to use. This year's trick features two of my favorite functions, the CUSUM function and the LAG function. By using these function, you can compute the rows of
The FREQ procedure in SAS supports computing exact p-values for many statistical tests. For small and mid-sized problems, the procedure runs very quickly. However, even though PROC FREQ uses efficient methods to avoid unnecessary computations, the computational time required by exact tests might be prohibitively expensive for certain tables. If
Did you know that the FREQ procedure in SAS can compute exact p-values for more than 20 statistical tests and statistics that are associated with contingency table? Mamma mia! That's a veritable smorgasbord of options! Some of the tests are specifically for one-way tables or 2 x 2 tables, but many apply
How do you simulate a contingency table that has a specified row and column sum? Last week I showed how to simulate a random 2 x 2 contingency table when the marginal frequencies are specified. This article generalizes to random r x c frequency tables (also called cross-tabulations) that have the same marginal row
A recent question posted on a discussion forum discussed storing the strictly upper-triangular portion of a correlation matrix. Suppose that you have a correlation matrix like the following: proc iml; corr = {1.0 0.6 0.5 0.4, 0.6 1.0 0.3 0.2, 0.5 0.3 1.0 0.1, 0.4 0.2 0.1 1.0}; Every correlation
When modeling and simulating data, it is important to be able to articulate the real-life statistical process that generates the data. Suppose a friend says to you, "I want to simulate two random correlated variables, X and Y." Usually this means that he wants data generated from a multivariate distribution,
This article shows how to visualize a surface in SAS. You can use the SURFACEPLOTPARM statement in the Graph Template Language (GTL) to create a surface plot. But don't worry, you don't need to know anything about GTL: just copy the code in this article and replace the names of
Suppose that you are tabulating the eye colors of students in a small class (following Friendly, 1992). Depending upon the ethnic groups of these students, you might not observe any green-eyed students. How do you put a 0 into the table that summarizes the number of students who have each
You can use SAS to generate random integers between 1–10 or in the range 1–100. This article shows how to generate random integers as easily as Excel does. I was recently talking with some SAS customers and I was asked "Why can't SAS create an easy way to generate random
In a previous post I described how to simulate random samples from an urn that contains colored balls. The previous article described the case where the balls can be either of two colors. In that csae, all the distributions are univariate. In this article I examine the case where the
If not for probability theory, urns would appear only in funeral homes and anthologies of British poetry. But in probability and statistics, urns are ever present and contain colored balls. The removal and inspection of colored balls from an urn is a classic way to demonstrate probability, sampling, variation, and
You've had a long day. You've implemented a custom algorithm in the SAS/IML language. But before you go home, you want to generate some matrices and test your program. If you are like me, you prefer a short statement—one line would be best. However, you also want the flexibility to
Occasionally a SAS statistical programmer will ask me, "How can I construct a large correlation matrix?" Often they are simulating data with SAS or developing a matrix algorithm that involves a correlation matrix. Typically they want a correlation matrix that is too large to input by hand, such as a
Dear Rick, I have a data set with 1,001 numerical variables. One variable is the response, the others are explanatory variable. How can I read the 1,000 explanatory variables into an IML matrix without typing every name? That's a good question. You need to be able to perform two sub-tasks:
Last week I discussed ordinary least squares (OLS) regression models and showed how to illustrate the assumptions about the conditional distribution of the response variable. For a single continuous explanatory variable, the illustration is a scatter plot with a regression line and several normal probability distributions along the line. The
I've previously written about how to generate a sequence of evenly spaced points in an interval. Evenly spaced data is useful for scoring a regression model on an interval. In the previous articles the endpoints of the interval were hard-coded. However, it is common to want to evaluate a function
A friend who teaches courses about statistical regression asked me how to create a graph in SAS that illustrates an important concept: the conditional distribution of the response variable. The basic idea is to draw a scatter plot with a regression line, then overlay several probability distributions along the line,
Statistical programmers often have to use the results from one SAS procedure as the input to another SAS procedure. Because ODS enables you to you to create a SAS data set from any ODS table or graph, it is easy to obtain a data set that contains the value of
In my previous blog post, I showed how you can use SAS to program a "weaving" algorithm that takes an image, cuts it into strips, and weaves the strips together to create mathematical art. I used matrices and heat maps for the computations and visualization. At the end of the
An artist friend of mine recently created a beautiful abstract image and described the process on her blog. She says that "after painting my initial square, I cut it into strips and split them down the middle, then wove them together.... I had no idea when I started piecing these
The title of this blog post might seem strange, but I occasionally need to compute the number of digits in a number, usually because I am trying to stuff an integer value into a string. Each time, I have to derive the formula from scratch, so I am writing this
Ugh! Your favorite regression procedure just printed a warning to the SAS log. Something is wrong, and your attempt to fit a model to the data has not succeeded. A typical message is "WARNING: The validity of the model fit is questionable," perhaps followed by some additional diagnostic messages about
One of my presentations at SAS Global Forum 2015 was titled "Ten Tips for Simulating Data with SAS". The paper was published in the conference proceedings several months ago, but I recently recorded a short video that gives an overview of the 10 tips: If your browser does not support
I wanna be an airborne ranger, Live the life of guts and danger.* If you are an 80's movie buff, you might remember the scene in The Breakfast Club where Bender, the juvenile delinquent played by Judd Nelson, distracts the principal by running through the school singing this song. Recently,
Typically a correlation analysis reports the correlations between all pairs of variables, including the variables with themselves. The resulting correlation matrix is square, symmetric, and has 1s on the main diagonal. But suppose you are interested in only specific combinations of variables. Perhaps you want the pairwise correlations between one
When SAS 9.4m3 was released last month (including SAS/STAT and SAS/IML 14.1), I was happy to see that a HEATMAP statement had been added to the SGPLOT procedure. Although heat maps in the SAS/IML language have been available for several releases, you previously had to use the Graph Template Language
Last month I wrote about how to simulate a drunkard's walk in SAS for a drunkard who can move only left or right in one direction. A reader asked whether the problem could be generalized to two dimensions. Yes! This article shows how to simulate a 2-D drunkard's walk, also
When using SAS to format a number as a percentage, there is a little trick that you need to remember: the width of the formatted value must include room for the decimal point, the percent sign, and the possibility of two parentheses that indicate negative values. The field width must
Last week's post about odds ratio plots in SAS made me think about a similar plot that visualizes the parameter estimates for a regression analysis. The so-called regression coefficient plot is a scatter plot of the estimates for each effect in the model, with lines that indicate the width of