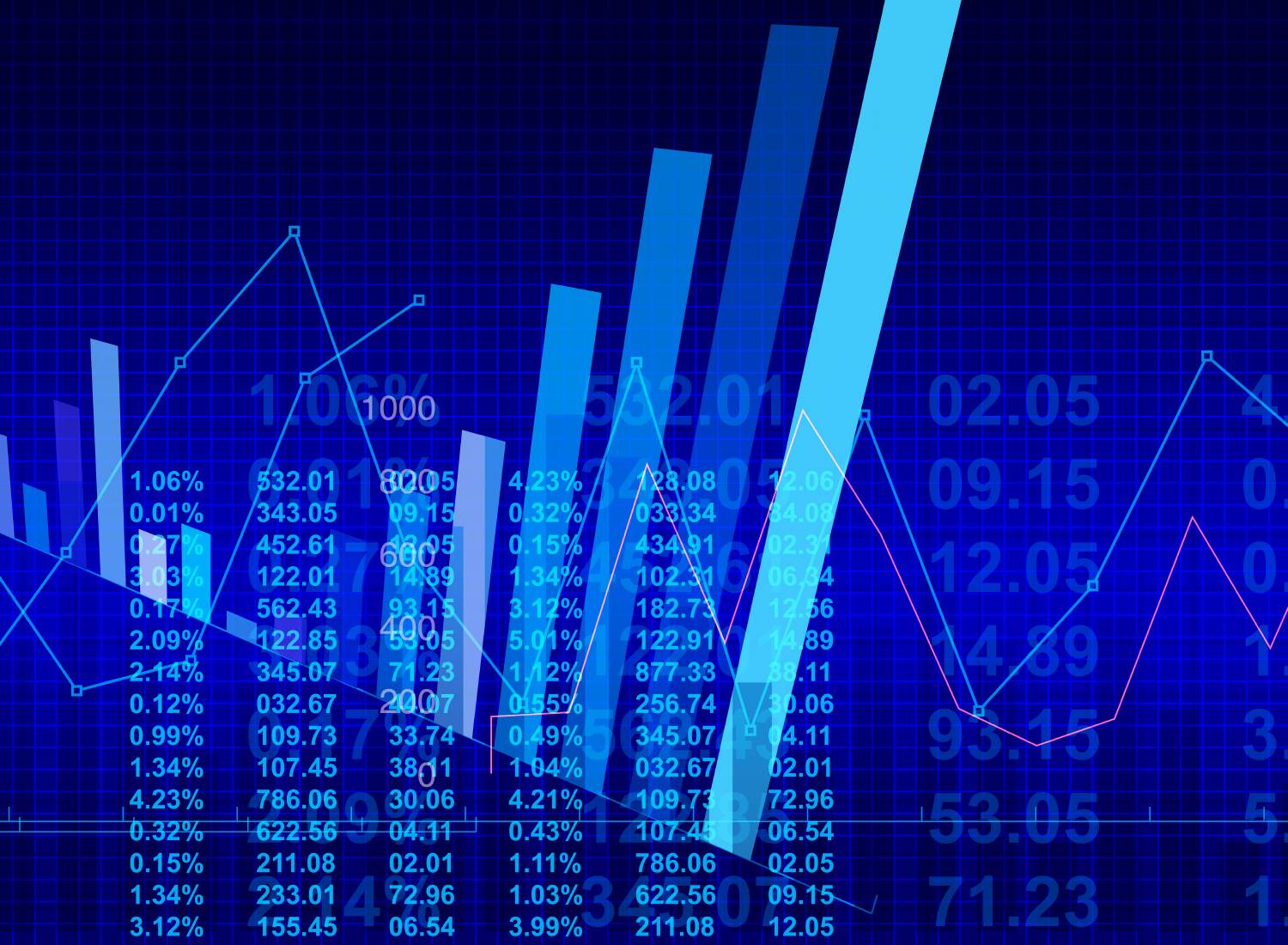
The global hype cycle of AI, driven in large part by ChatGPT, is dying down and real-world artificial intelligence (AI) adoption and application are taking hold. Early adopters are reaping rewards, and AI leaders are driving significant change in their business models. Banking as a sector was quick to grasp