My recent work has focused heavily on migration, especially onto the SAS Viya platform and cloud more generally. Rather unexpectedly during this process, we have found that data observability is becoming increasingly important to customers. They start simply by looking at tracing files, but soon find that it has a whole range of other benefits. For many of them, it is now an essential way to manage and optimize workflows, troubleshoot problems and issues, monitor performance, and understand and allocate costs appropriately.
It turns out that this is part of a growing trend. Data quality is not new—but it is now becoming a multi-million dollar business.
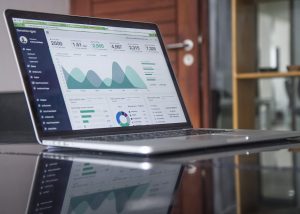
What is data observability?
In a very simplistic way, Data observability is the ability to be able to “observe” your data and your systems, and what is going on within them. The term “observability” was coined by engineer Rudolf E. Kálmán in 1960 in his paper “On the General Theory of Control System”1. It has since grown to mean many different things in different communities. In software engineering we can go deeper and think in Observability as the capability to understand how our applications work, in which states they transit from one to another, and narrowing the speech into our SAS analytical spectrum, predict new possible states they may fall, all of this by observing and interrogating with external tools. That’s one of the key aspects of all of this, not being intrusive within the systems.
It is, effectively, visibility of systems, workflows and data within an analytics environment. You may be wondering how this differs from having tracing models or monitoring of logins. The answer is that data observability systems such as SAS Enterprise Session Monitor provide all this—and then considerably more.
These systems give you the ability to oversee multiple users within the same environment and the jobs and workloads that they are generating. You can therefore manage these effectively. This means that you can keep the platform performance stable and predictable. In a cloud environment, you can also control costs by levelling out demand rather than having too many peaks and troughs. Ultimately, data observability systems can improve efficiency, at scale, and across multiple users. Going a step further with this into the enterprise, mixing Data observability with AIOPs will make the complete automation for operations for distributed applications and of course with the benefits of using all the capabilities regarding artificial intelligence already in place in our SAS platform.
Features of data observability systems
What should you look for in a data observability system? There are several features that are ideal, including:
- Real-time contextual identification of workload
You need to be able to see everything that is going on within the platform, in real time. This allows users and administrators to see if everything is behaving as expected, or if any action is required. Ideally, the workloads should be labelled appropriately, for example, with the job name, job flow hierarchy, and server context. This means that users can quickly identify any problems with their jobs, and administrators can rapidly act to prevent any major problems.
- Self-service administration and event investigation
Enabling users to identify problems quickly is no help if they cannot immediately act to address them. The best data observability systems provide secure and controlled ways for users to terminate workloads appropriately. Obviously, they must have the necessary permissions to do so, and a record of the termination and its justification should be saved onto an audit log. This increases the visibility of the overall process, and ensures that nobody can terminate another user’s job maliciously. Users also need to be able to view event logs in real time, and investigate incidents where necessary, and where they have the required permissions. This feature improves efficiency and enables users to act as their own helpdesk.
- Reliable and accurate performance analysis
Both users and administrators may be interested in analyzing the performance of the analytics platform. Administrators are most likely to need a top-down view of workflows, enabling them to identify hotspots and individuals or jobs that may be causing problems. Users are more likely to want to see the detail about their own jobs, including performance and resource use. They, too, want to be able to identify problems, and optimize performance where necessary.
- Scheduled job flow analysis and optimization
It is helpful to have a platform that enables scheduled job monitoring and analysis. It should automatically identify warnings or errors, and provide real-time alerts to enable rapid action. Analysis and monitoring functions should also support root-cause analysis and anomaly identification.
- Allocation of costs and resource use
Finally, the best data observability platforms and systems will allow users and administrators to allocate costs and resources to particular projects and/or users. This may also be useful for historical performance comparisons. Ideally, any interface for users will be simple enough that they can use ‘drag and drop’ to generate rules for cost allocations. This can then be used to provide regular reports to departments or teams.
See what you need?
Key to the rise in data observability is the importance of being able to see what is going on—and therefore rapidly prevent or address problems. Finding the right data observability platform can make all the difference.
1 Rudolf E. Kálmán, “On the General Theory of Control Systems”, IFAC Proceedings Volumes 1, no. 1 (August 1960): 491–502.