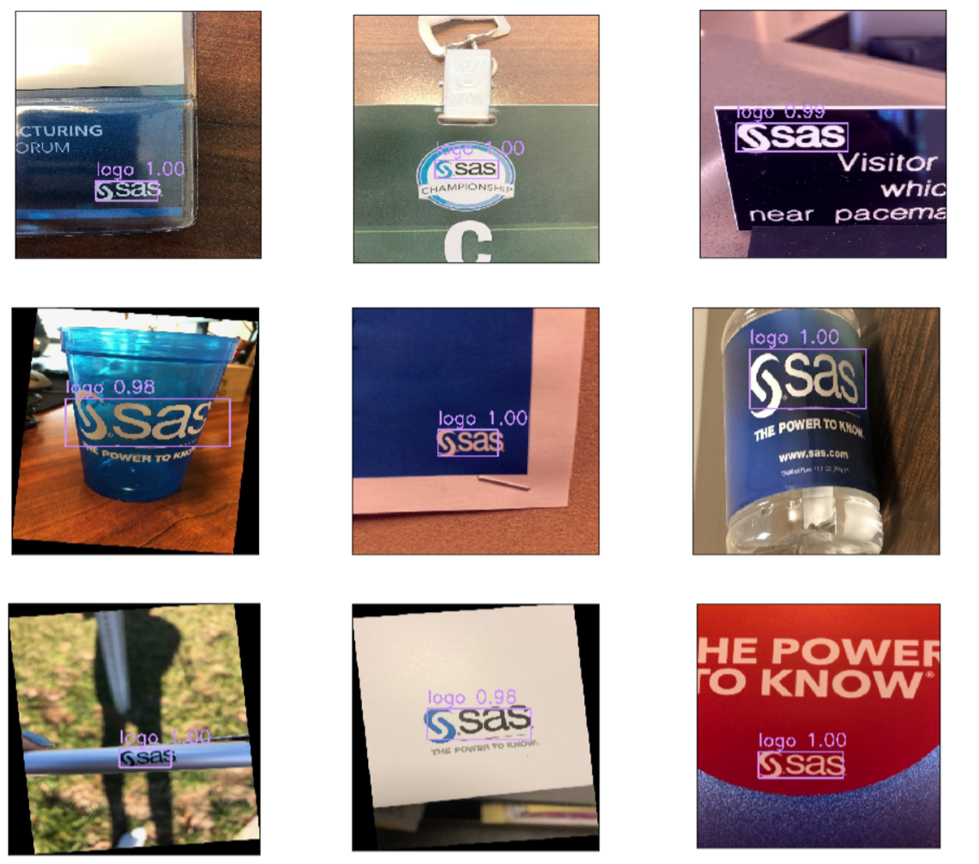
Using SAS Viya in combination with open-source capabilities, we were able to develop an automated solution for logo detection that does not require any manual data labeling.
Using SAS Viya in combination with open-source capabilities, we were able to develop an automated solution for logo detection that does not require any manual data labeling.
こんにちは!SAS Institute Japanの堀内です。今回も自然言語処理について紹介いたします。 前回の投稿では、実際にSASを使って日本語の文章を扱う自然言語処理の例を解説しました。 最終回の本投稿ではその応用編として、自然言語処理の代表的なタスクとSASによる実装方法を紹介します。なお、ここでいうタスクとは「定式化され一般に共有された課題」といった意味になります。自然言語処理には複数のタスクがあり、タスクごとに、共通する部分はあるとはいえ、問題解決のアプローチ方法は基本的に大きく異なります。SASには各タスクごとに専用のアクションセット1が容易されています。 要約タスク その名の通り文章を要約するタスクです。SASではtextSummarizeアクションセットで対応可能です。 ここでは、NHKのニュース解説記事「気になる頭痛・めまい 天気が影響?対処法は?」(https://www.nhk.or.jp/kaisetsu-blog/700/471220.html) の本文を5センテンスで要約してみましょう。 import swat conn = swat.CAS('mycashost.com', 5570, 'username', 'password') conn.builtins.loadActionSet(actionSet='textSummarization') conn.textSummarization.textSummarize(addEllipses=False, corpusSummaries=dict(name='corpusSummaries', compress=False, replace=True), documentSummaries=dict(name='documentSummaries', compress=False, replace=True), id='Id', numberOfSentences=5, table={'name':CFG.in_cas_table_name}, text='text', useTerms=True, language='JAPANESE') conn.table.fetch(table={'name': 'corpusSummaries'}) numberOfSentencesで要約文のセンテンス数を指定しています。結果は以下の通りです。 'まず体調の変化や天気、気温・湿度・気圧などの日記をつけ、本当に天気が影響しているのか、どういうときに不調になるのかパターンを把握すると役立ちます。 気温・湿度以外にも、気圧が、体調の悪化や、ときに病気の引き金になることもあります。 私たちの体は、いつも耳の奥にある内耳にあると言われている気圧センサーで、気圧の変化を調整しています。 ただ、天気の体への影響を研究している愛知医科大学佐藤客員教授にお話ししを伺ったところ、「台風最接近の前、つまり、気圧が大きく低下する前に、頭が痛いなど体調が悪くなる人は多い」ということです。 内耳が敏感な人は、わずかな気圧の変化で過剰に反応し、脳にその情報を伝えるので、脳がストレスを感じ、体のバランスを整える自律神経が乱れ、血管が収縮したり、筋肉が緊張するなどして、その結果、頭痛・めまいなどの体に様々な不調につながっているのです。' 重要なセンテンスが抽出されていることが分かります。 テキスト分類タスク 文章をいくつかのカテゴリに分類するタスクです。その内、文章の印象がポジティブなのかネガティブなのか分類するものをセンチメント分析と呼びます。ここでは日本語の有価証券報告書の文章をポジティブかネガティブか判定してみます。使用するデータセットは以下になります。 https://github.com/chakki-works/chABSA-dataset (なお、こちらのデータセットには文章ごとにポジティブかネガティブかを示す教師ラベルは元々付与されておりませんが、文章内の特定のフレーズごとに付与されているスコアを合算することで教師ラベルを合成しております。その結果、ポジティブ文章は1670文章、ネガティブ文章は1143文章、合計2813文章になりました。教師ラベルの合成方法詳細はこちらのブログをご覧ください。) pandasデータフレームにデータを格納した状態を確認してみましょう。 df = pd.read_csv(CFG.local_input_file_path) display(df)
Welcome to the continuation of my series Getting Started with Python Integration to SAS Viya. In previous posts, I discussed how to connect to the CAS server, how to execute CAS actions, and how to filter CAS tables. Now it's time to focus on how to summarize columns. Load and explore data Let's first load
こんにちは!SAS Institute Japanの堀内です。今回も自然言語処理について紹介いたします。 第1回目の投稿では、最近の自然言語処理の応用例とSAS社が携わった自然言語処理関連の実案件の概要を紹介しました。 第2回目の本投稿では実際にSASを使って日本語の文章を扱う自然言語処理の例を解説していきます。 テキストデータって何? 自然言語処理を語る前に、自然言語処理が処理対象とするデータのことを知る必要があります。自然言語処理で扱われるデータはテキストデータと呼ばれています。ここからはテキストデータがどういうものか探っていきます。 テキストとは以下のようなものです。 「自然言語処理で扱われるデータはテキストデータと呼ばれています。本投稿ではテキストデータがどういうものか探っていきます。」 何の変哲もない日本語の文章です。日本語以外の言語で書かれた文章ももちろんテキストと呼ばれます。 ではテキストデータとは何でしょう?データと言うからには何らかの構造を持っていると考えます。例えば行と列が与えられたテーブルデータがわかりやすい例です。 テキストデータと呼ぶとき、テキストに何らかの構造を与えられたものを想起すると良いかと思います。上で挙げたサンプルのテキストをテキストデータに変換してみましょう。 ["自然言語処理で扱われるデータはテキストデータと呼ばれています。", "本投稿ではテキストデータがどういうものか探っていきます。"] これは句読点でテキストを区切り、リストに格納した例です。やりかたは他にもあります、 [["自然言語処理", "で", "扱われる", "データ", "は", "テキストデータ", "と", "呼ばれて", "います", "。"], ["本投稿", "では", "テキストデータ", "が", "どういうもの", "か", "探って", "いきます", "。"]] これは先ほどの例で2つのテキストに区切ったうえで、それぞれのテキストを更に単語ごとに区切って別々のリストに格納した例になります。これをテーブルデータのように整えると、 ID COL1 COL2 COL3 COL4 COL5 COL6 COL7 COL8 COL9 COL10 1 自然言語処理 で 扱われる
Let's create a Multi-stage Computer Vision model to detect objects on high-resolution imagery taken from an aerial view. The goal is to locate a dog and determine if he is wearing a scarf or not and what color the scarf is.
Welcome to the continuation of my series Getting Started with Python Integration to SAS Viya. In previous posts, I discussed how to connect to the CAS server, how to execute CAS actions, and how to filter CAS tables. Now it's time to focus on creating calculated columns on a CAS table. Load and explore
Welcome to the seventh installment in my series Getting Started with Python Integration to SAS Viya. In previous posts, I discussed how to connect to the CAS server, how to execute CAS actions, and how to work with the results. Now it's time to learn how to filter CAS tables. Load and explore data
The Proc Python procedure, Python code editor & Python code step facilitate low-code analytics calling Python and SAS from a common interface. Data scientists also appreciate the connection to Python & R through the Model Studio Open-source Code node. Older methods of interaction include the swat and sas_kernel packages running on Python clients.
Welcome to the sixth installment in my series Getting Started with Python Integration to SAS Viya. In previous posts, I discussed how to connect to the CAS server, how to execute CAS actions, and how to work with the results. Now it's time to generate simple descriptive statistics of a CAS table. Let's begin
[Editor's note: this post was co-authored by Marinela Profi and Wilbram Hazejager] Data science teams are multidisciplinary, each with different skills and technologies of choice. Some of them use SAS, others may have analytical assets already built in Python or R. Let's just say each team is unique. As part
This post, written by Radhikha Myeni and Jagruti Kanjia, will demonstrate how easy it is to build and deploy a machine learning pipeline by using SAS and Python. The Model Studio platform provides a quick and collaborative way to build complex pipelines by dragging and dropping nodes from a web-based
Welcome to the fifth installment in my series Getting Started with Python Integration to SAS Viya. In previous posts, I discussed how to connect to the CAS server, how to execute CAS actions, how to work with the results, and how your data is organized on the CAS server. In
Welcome to the fourth installment in my series Getting Started with Python Integration to SAS Viya. In previous posts, I discussed how to connect to the CAS server, how to execute CAS actions, and how to work with the results. Now it's time to understand how your data is organized
In this article, we summarize our SAS research paper on the application of reinforcement learning to monitor traffic control signals which was recently accepted to the 34th Conference on Neural Information Processing Systems (NeurIPS 2020), Vancouver, Canada. This annual conference is hosted by the Neural Information Processing Systems Foundation, a non-profit corporation that promotes the exchange of ideas in neural information processing systems across multiple disciplines.
A year ago we could not imagine stadiums being empty during the most exciting sports events, but it is a common sight now. The entertainment sector is one of the hardest hit sectors because of the COVID-19 pandemic [1]. Social distancing requirements made it impossible to have viewers in stadiums
The model management process, which is part of ModelOps, consists of registration, deployment, monitoring and retraining. This post is part of a series examining the model management process, orchestrated through the Model Manager (MM) APIs. The focus of part one is on model registration, specifically on using the APIs from
An embedding model is a way to reduce the dimensionality of input data, such as images. Consider this to be a type of data preparation applied to image analysis. When an embedding model is used, input images are converted into low-dimensional vectors that can be more easily used by other computer vision tasks. The key to good embedding is to train the model so that similar images are converted to similar vectors.
Are you looking for a specific CAS action to use in your project? Maybe you need to create a linear or logistic regression and can't seem to find the CAS action? In this post in the Getting Started with Python Integration to SAS® Viya® series, we are going to look
In the second post of the Getting Started with Python Integration to SAS® Viya® series we will learn about Working with CAS Actions and CASResults Objects. CAS actions are commands sent to the CAS server to run a task, and CASResults objects contain information returned from the CAS server. This
CAS Actions and Action Sets - a brief intro - A quick introduction about the distributed CAS server in SAS® Viya®. Index of articles on Getting Started with Python Integration to SAS Viya. Making a Connection - An introduction to SAS Viya and the massively parallel processing CAS engine, and
Learning never stops. When SAS had to change this year’s SAS Global Forum (SASGF) to a virtual event, everyone was disappointed. I am, however, super excited about all of the papers and stream of video releases over the last month (and I encourage you to register for the upcoming live
We will use prescriptive analytics and optimization to select a stock portfolio that maximizes returns while taking risk tolerance into account.
With increasing interest in Continuous Integration/Continuous Delivery (CI/CD), many SAS Users want to know what can be done for Visual Analytics reports. In this article, I will explain how to use Python and SAS Viya REST APIs to extract a report from a SAS Viya environment and import it into another environment.
Let's talk about using DLPy to model employee retention through a survival analysis model. Survival analysis is used to model time-to-event. Examples of time-to-event include the time until an employee leaves a company, the time until a disease goes into remission, or the time until a mechanical part fails. The
Welcome to the first post for the Getting Started with Python Integration to SAS Viya series! With the popularity of the Python programming language for data analysis and SAS Viya's ability to integrate with Python, I thought, why not create tutorials for users integrating the two? To begin the series
Whether you like it or not, Microsoft Excel is still a big hit in the data analysis world. From small to big customers, we still see fit for daily routines such as filtering, generating plots, calculating items on ad-hoc analysis or even running statistical models. Whenever I talk to customers,
SAS Enterprise Guide(EG)で簡単にPythonなどオープンソース・コードを実行できる方法を紹介します。 1.オープンソースコード実行用タスク(OST)の概要 2.事前設定 3.EGでの使用方法 4.AMOでの使用方法 1.オープンソースコード実行用タスク(OST)の概要 SAS Enterprise Guide(EG)は直感的なユーザインタフェース上で、マウスの簡単操作で、透過的にデータにアクセスし、様々な分析用タスクを活用し、データの準備から加工・変換、集計・分析、レポート作成に至る一連の作業をグラフィカルなフロー図として描き、実行することができるツールです。 一方、多くの組織ではPython, Rなど様々なオープンソースのスキルを持つデータサイエンティストが混在することが多く、こうしたオープンソース(OS)とのコラボレーションも必要になってきています。従来、EGとPythonなどOSと連携する際には、データでのやりとりが必要でした。例えば、EGで作成したデータをエクスポートし、OSユーザに渡して処理してもらうか、その逆か、になります。 都度このようにデータをやりとりするには手間と時間がかかりますし、データやPythonコードなどの管理も課題となります。こうした課題を解決する手段の一つとして、カスタムタスクを活用することができます。 カスタムタスク:EGには、数多くのタスク(データ準備用タスク、分析用タスク、など)が実装されています。このタスクは顧客ニーズに応じてカスタムで作成し、活用することができるようになっています。SASのサポートサイトからカスタムタスクを作成するためのテンプレートをダウンロードし、使用することができるようになっています。カスタムタスクの作成方法の詳細に関しては、次回のブログでご紹介します。 オープンソースコード実行用タスク(OST):OSTはOpenSourceTaskの略で、EGカスタムタスクのテンプレートに基づいて開発されています。OSTを使用することで、EG上で簡単にPythonコードを実行することができ、GUIベースの簡単マウス操作でPythonコードを再利用し、EG上で、標準実装のタスクとOSTを活用し、連携してアナリティクス作業を完結することができます。これによって、様々異なる分析スキル(GUIユーザー、SAS言語ユーザー、OS言語ユーザー)を連携し、有効活用することが可能となります。また、この分析処理は自動化することも可能です。 次にPythonコード実行用のOSTの使用方法を紹介します。EGのバージョンは8.2を使用したものです。 2.事前設定 ・Python環境の確認 まずSAS9サーバ側にPython環境がインストールされていることを確認してください。Pythonの環境がない場合は、PythonまたAnacondaなどPythonのruntimeをインストールする必要があります。 ・SAS9サーバーの設定 SAS9のSMC(SAS Management Console)を起動し、以下の画面を開きます。 「Environment Management」>「Server Manager」> 「SASApp」>「SASApp Logical Workspace Server」>「SASApp - Workspace」を右クリックし、「Properties」をクリックし 以下の設定でXCMDの実行を許可します。 「Options」タブ >「Advanced Options」>「Launch Properties」で「Allow XCMD」をチェックし、「OK」をクリックします。 設定を有効するにはシステムのObject Spawnerのサービスを再起動する必要があります。 ・OSTパッケージの取り込み OSTパッケージをダウンロードし、展開したCustomフォルダをEG(またAMO)のインストール先(以下画面ショットのロケーション)にコピーします。そのほか、臨時ファイル保存と作業用フォルダC:/Customを作成し、準備作業が完了です。 これで、OSTが使用できる状態になります。 3.EG上の使用方法 まず EGを起動し、処理対象データを選択します。今回はSASHELPライブラリ内にある「CLASS」を選びます。データを選択した状態で、タスクリストのSAS
SAS' Kris Stobbe shows how you can predict survival rates of Titanic passengers with a combination of both Python and CAS using SWAT, then see how the models performed.
This blog is a part of a series on the Data Science Pilot Action Set. In this blog we review all nine actions in Python. Have you noticed the button bar in the upper right-hand corner of the SAS Visual Data Mining and Machine Learning Programming Guide? This button bar
Muchas organizaciones equilibran las soluciones de código abierto con software comercial para cumplir con los requisitos de análisis estadístico, tanto dentro de sus organizaciones como externamente con los organismos reguladores. Si bien las herramientas analíticas de código abierto ofrecen una comunidad en línea sólida y una amplia gama de algoritmos,