Get the latest machine learning algorithms and techniques
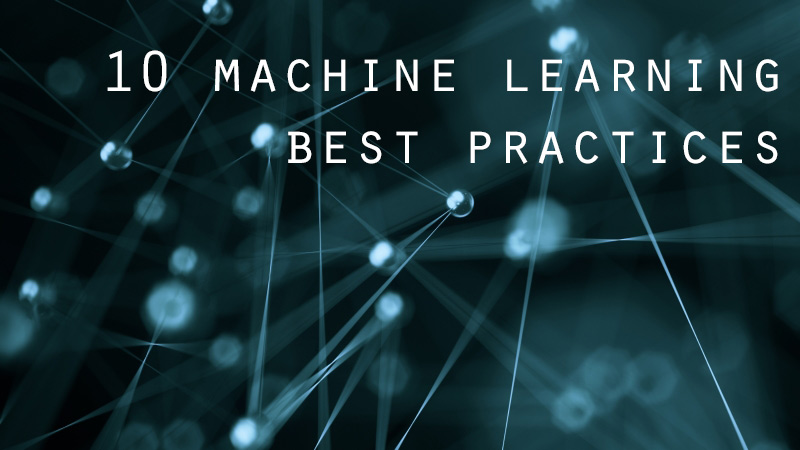
I started my training in machine learning at the University of Tennessee in the late 1980s. Of course, we didn’t call it machine learning then, and we didn’t call ourselves data scientists yet either. We used terms like statistics, analytics, data mining and data modeling. Regardless of what you call