La integración del Big Data en los procesos y programas de gestión de datos existentes se ha convertido en algo así como una llamada de alerta para las organizaciones en su afán de convertirse en empresas del siglo XXI. Jim Harris, blogger y obsesivo-compulsivo del Data Quality, nos da estos
Tag: data management

In today’s brave new technological world, most of us live cocooned in thermo-regulated cars and buildings. Our food and drink is on tap, and we experience little inconvenience beyond death and taxes. It’s easy to forget that life as we know it would come to an abrupt halt without the

Sizing is a topic that solutions managers typically leave until the end after decisions about the application have been settled. But there are often many variables that can impact the final size requirement. We have seen across our customer base that sizing and the number of environments has been determined
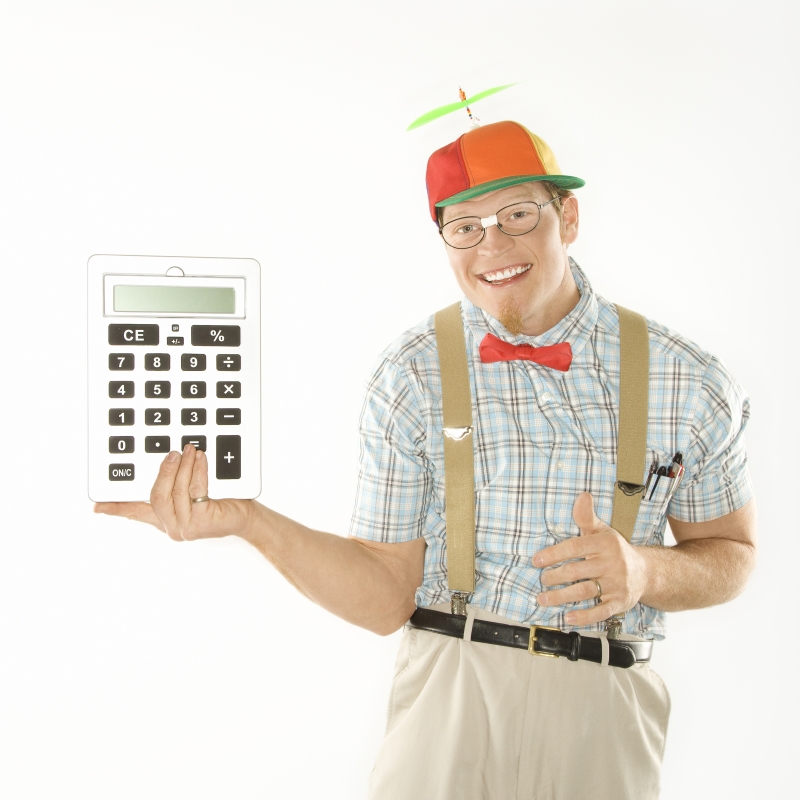
If I were to believe the feedback I get, statisticians are among the most difficult people to work with. What’s more, they’re the only group that should be allowed to work in data analytics. It sounds harsh, but this may explain why big data projects continually fail. Businesses need statisticians who are both
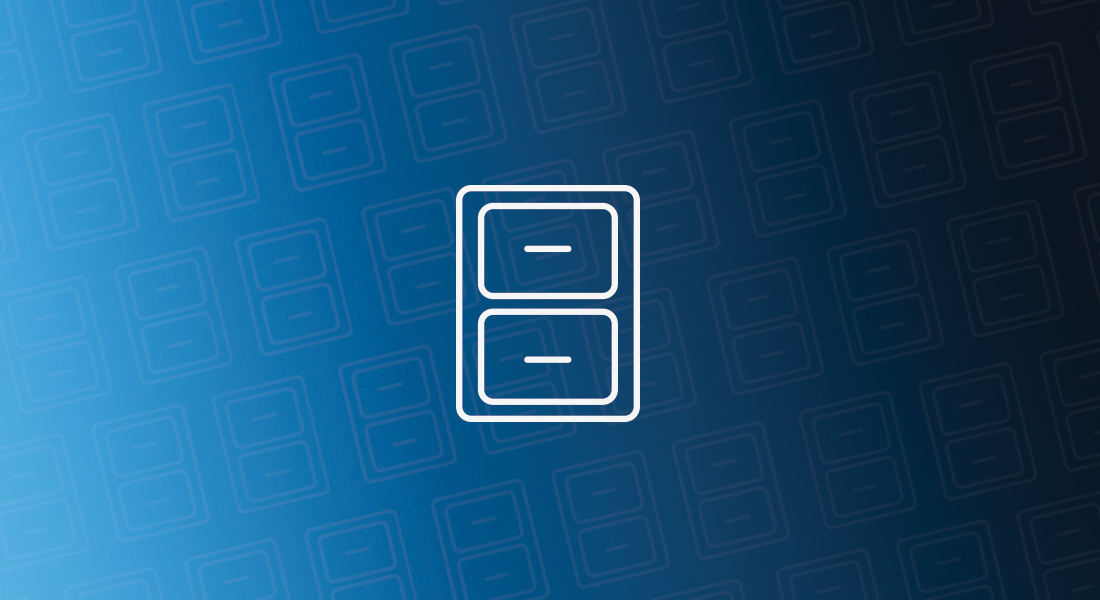
Healthcare IT News recently published an article on 18 health technologies poised for big growth, a list culled from a HIMSS database. The database is used to track an extensive list of technology products that have seen growth of 4-10 percent since 2010, but have not yet reached a 70
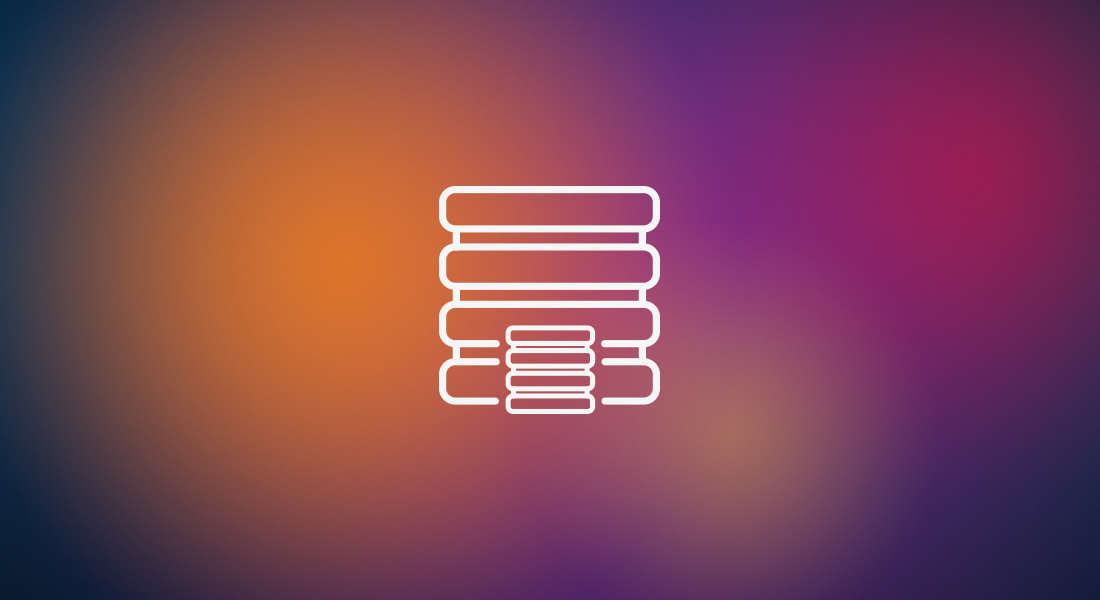
New York City is a pioneer in use of technology in many ways. For instance, the work of the Mayor’s Office of Data Analytics has been cited repeatedly as an example of smart city innovation. But the innovation doesn’t stop there. Two projects that used SAS data visualization and data
It’s been an amazing journey with Hadoop. As we discussed in an earlier blog, Hadoop is informing the basis of a comprehensive data enterprise platform that can power an ecosystem of analytic applications to uncover rich insights on large sets of data. With YARN (Yet Another Resource Negotiator) as its
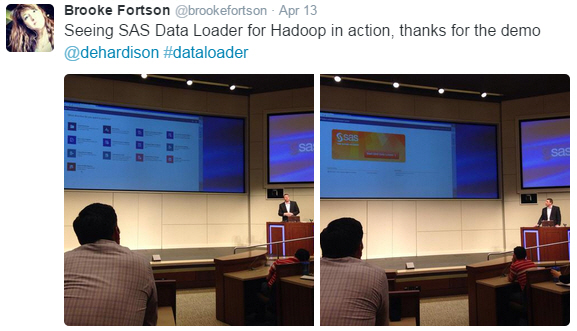
A week from today, we'll be in New York City for Strata + Hadoop World, where we’ll kick things off at the Opening Reception. Be sure to stop by booth 543 to meet the team IRL (in real life)! They are excited about the event and eager to talk with attendees.
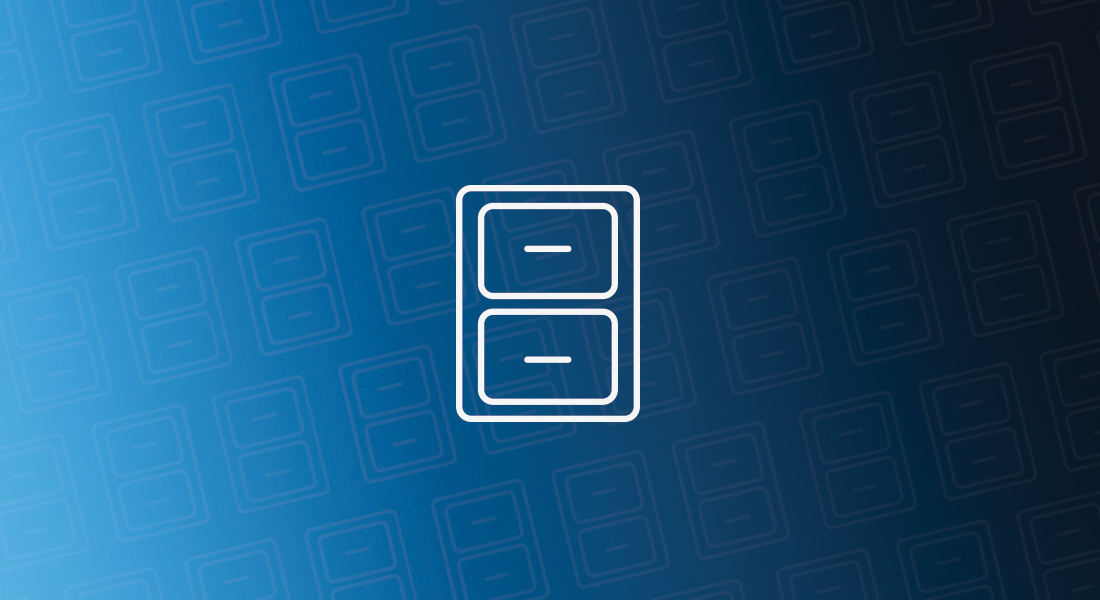
It’s rather appropriate that the rock band Europe recorded the hit “The Final Countdown”, because today, September 22nd, represents 100 days until the much anticipated (and delayed) European insurance legislation Solvency II will come into effect on January 1st 2016. Designed to introduce a harmonized, EU-wide insurance regulation, Solvency II
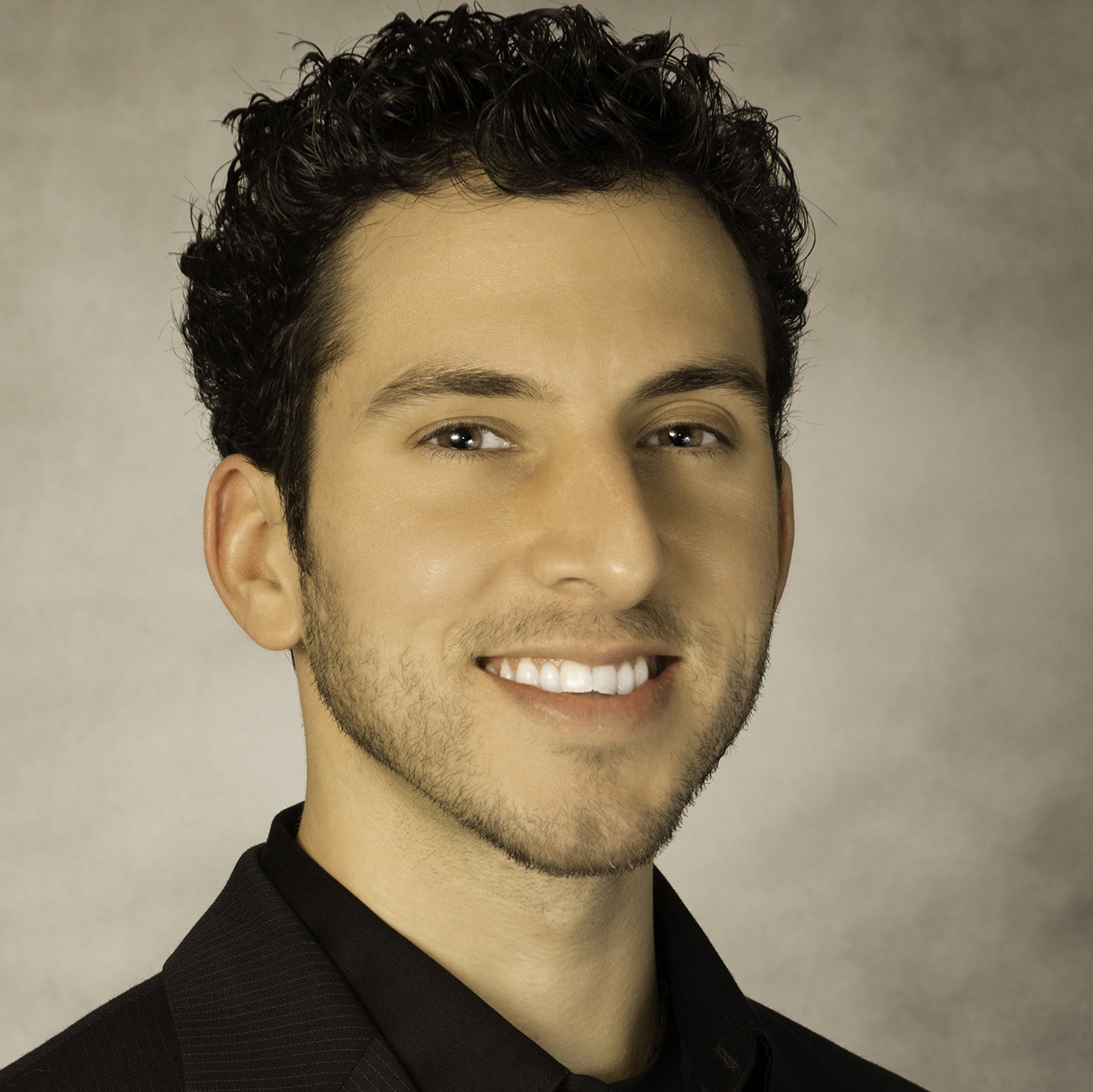
It’s me again!! We're at the halfway point of meeting our Strata + Hadoop World dream team. So far, you’ve met machine learning guru Patrick Hall; data management expert Clark Bradley; and advanced analytics specialist Rachel Hawley. Next up … Dan Zaratsian! I met Dan a few years back while preparing for Analytics 2013
Data integration, on any project, can be very complex – and it requires a tremendous amount of detail. The person I would pick for my data integration team would have the following skills and characteristics: Has an enterprise perspective of data integration, data quality and extraction, transformation and load (ETL): Understands
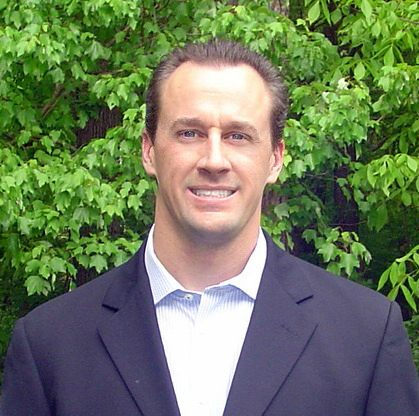
Meet Clark Bradley: SAS technical architect by day and comedian by night. When he’s not demoing SAS Data Loader for Hadoop, he’s blogging about it on The Data Roundtable. Clark and a core SAS team of thought leaders, developers and executives will be in New York City on September 29 at Strata
A few of our clients are exploring the use of a data lake as both a landing pad and a repository for collection of enterprise data sets. However, after probing a little bit about what they expected to do with this data lake, I found that the simple use of
Many people who plan data governance initiatives ignore the need for a business case. "We've already had approval for the project; why do we need a business case when we've got the budget signed off?" The perception is that because they have a strong commitment, there is no need to get
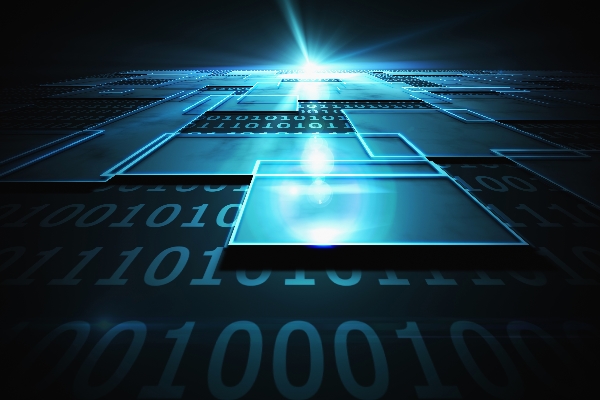
In the oil and gas industry, analytics are used to improve both upstream and downstream operations, from optimizing exploration and forecasting production to reducing commodity trading risk and understanding customer's energy needs. If you plan to derive value from the digital oil field, big data, and analytics, one of the first things
Operationalizing data governance means putting processes and tools in place for defining, enforcing and reporting on compliance with data quality and validation standards. There is a life cycle associated with a data policy, which is typically motivated by an externally mandated business policy or expectation, such as regulatory compliance.
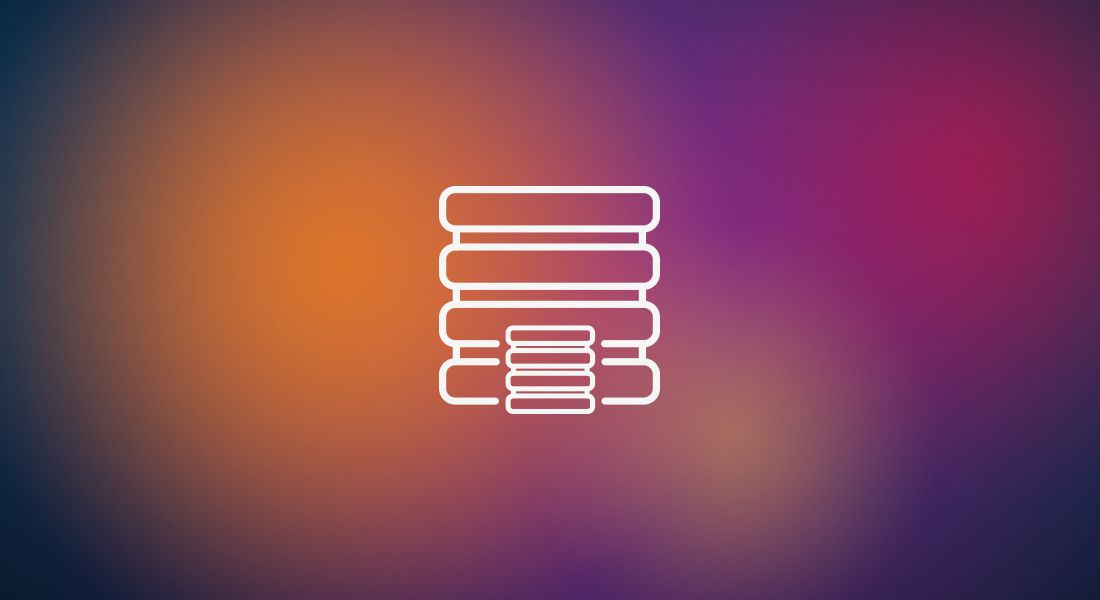
What does the future of analytics look like in your organizations enterprise architecture? Does it include thinking about a two speed approach to analytics which includes both: An agile rapidly changing analytics platform for innovation (a lab) seperated from operations and broad enterprise audience usage A slowly moving systematic enterprise analytics platform (a factory)
If your organization is large enough, it probably has multiple data-related initiatives going on at any given time. Perhaps a new data warehouse is planned, an ERP upgrade is imminent or a data quality project is underway. Whatever the initiative, it may raise questions around data governance – closely followed by discussions about the
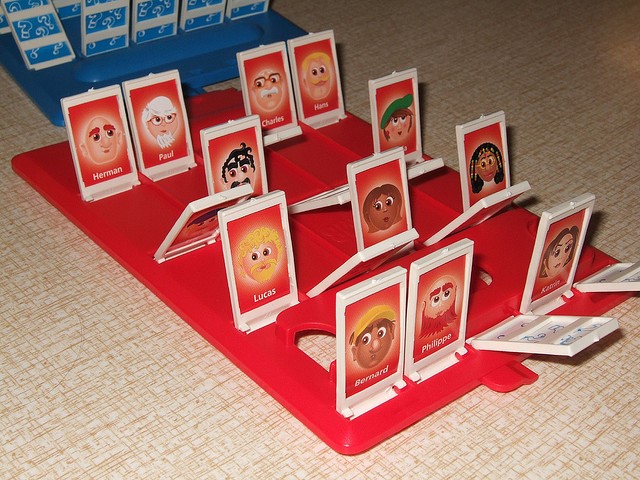
I’ve spent some time over the past couple of months learning more about anonymization. This began with an interest in the technical methods used to protect sensitive personally-identifiable information in a SAS data warehouse and analytics platform we delivered for a customer. But I learned that anonymization has two rather different meanings; one in the
In recent years, we practitioners in the data management world have been pretty quick to conflate “data governance” with “data quality” and “metadata.” Many tools marketed under "data governance" have emerged – yet when you inspect their capabilities, you see that in many ways these tools largely encompass data validation and data standardization. Unfortunately, we

Big data. Streaming data. Complex data. We’ve all heard the reasons why organizations feel like they’re facing an insurmountable data challenge. Now, it’s time to do something about it. For the past few years, SAS has helped some of the world’s leading companies make sense of an avalanche of data.
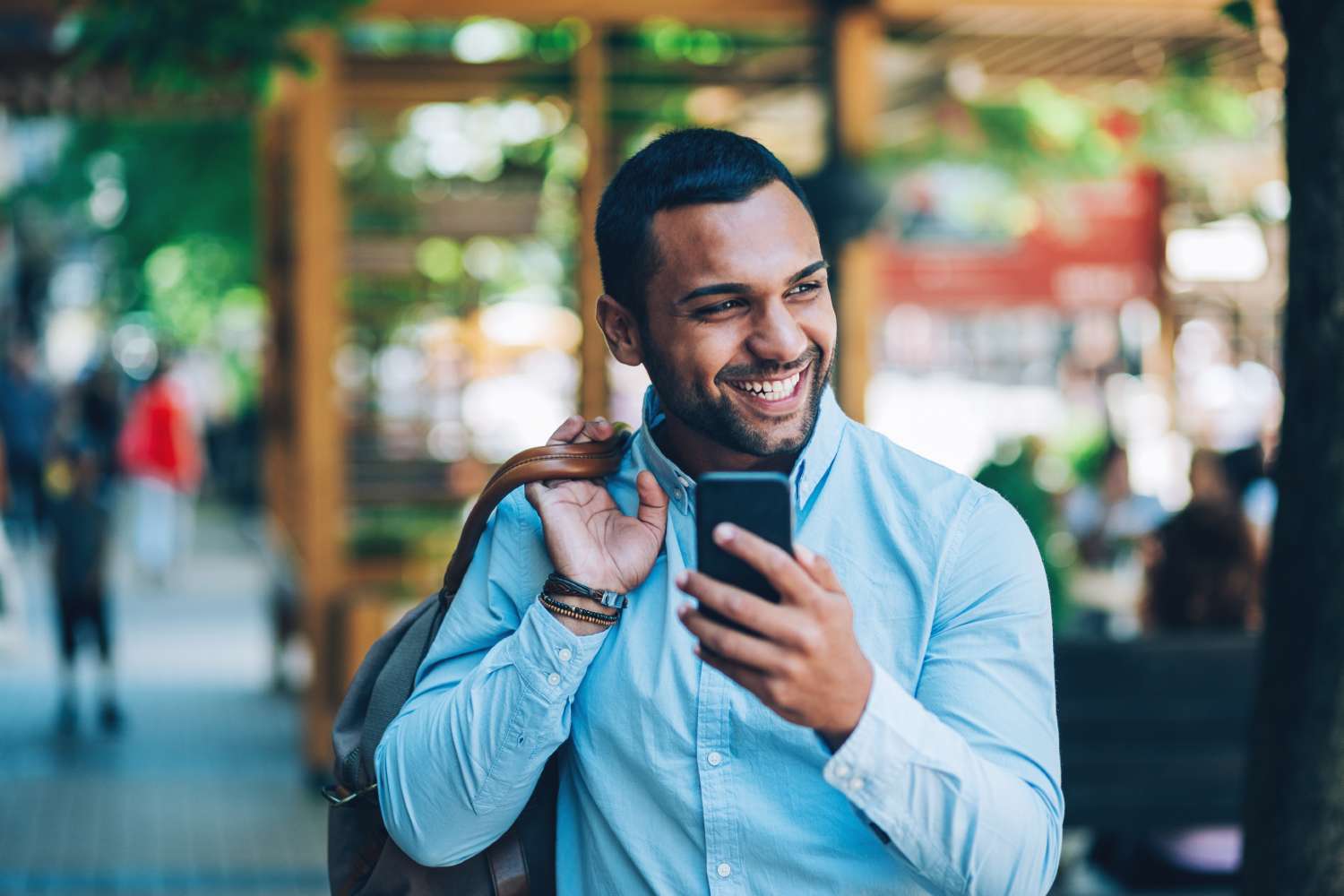
Jim Harris says event stream processing determines if big data is eventful and relevant enough to process and store.
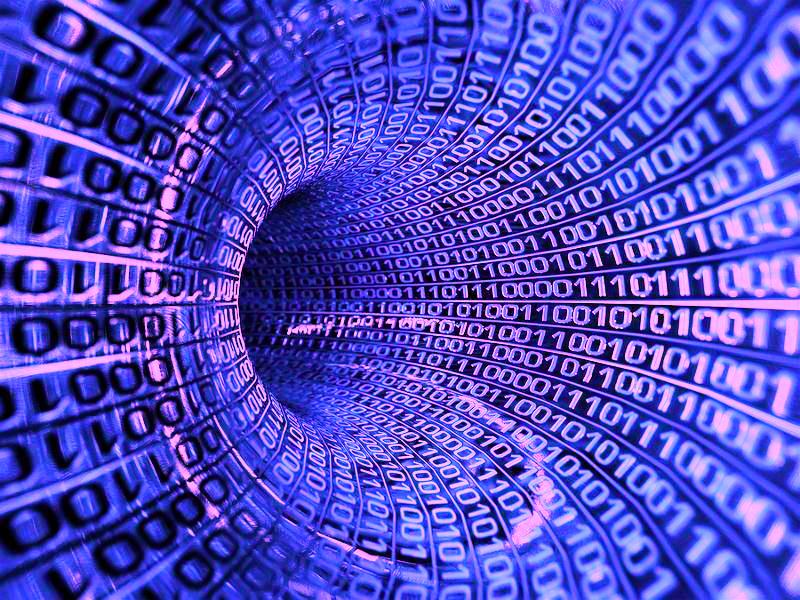
The metaphors we choose to describe our data are important, for they can either open up the potential for understanding and insight, or they can limit our ability to effectively extract all the value our data may hold. Consisting as it does of nothing but electric potentials, or variations in
I believe most people become overwhelmed when considering the data that can be created during event processing. Number one, it is A LOT of data – and number two, the data needs real-time analysis. For the past few years, most of us have been analyzing data after we collected it,
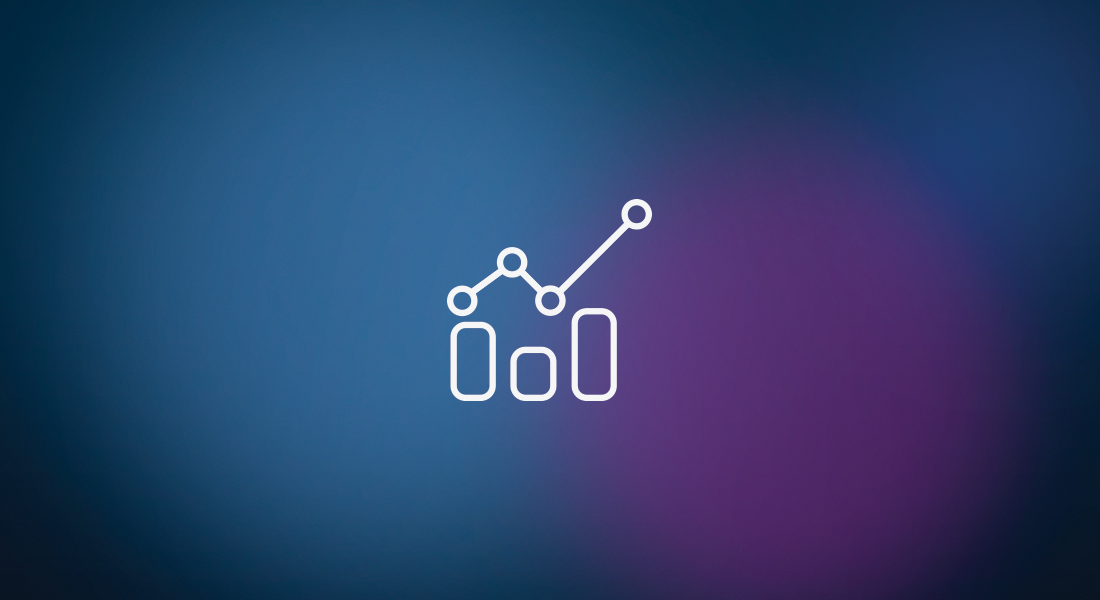
You've probably heard many times about the fantastic untapped potential of combining online and offline customer data. But relax, I’m going to cut out the fluff and address this matter in a way that makes the idea plausible and its objectives achievable. The reality is that while much has been
In my last two posts, I introduced some opportunities that arise from integrating event stream processing (ESP) within the nodes of a distributed network. We considered one type of deployment that includes the emergent Internet of Things (IoT) model in which there are numerous end nodes that monitor a set of sensors,
In my previous post, I discussed the similarities, differences and overlap between event stream processing (ESP) and real-time processing (RTP). In this post, I want to highlight three things that need to get real. In other words, three things that should be enhanced with real-time capabilities, whether it’s ESP, RTP or
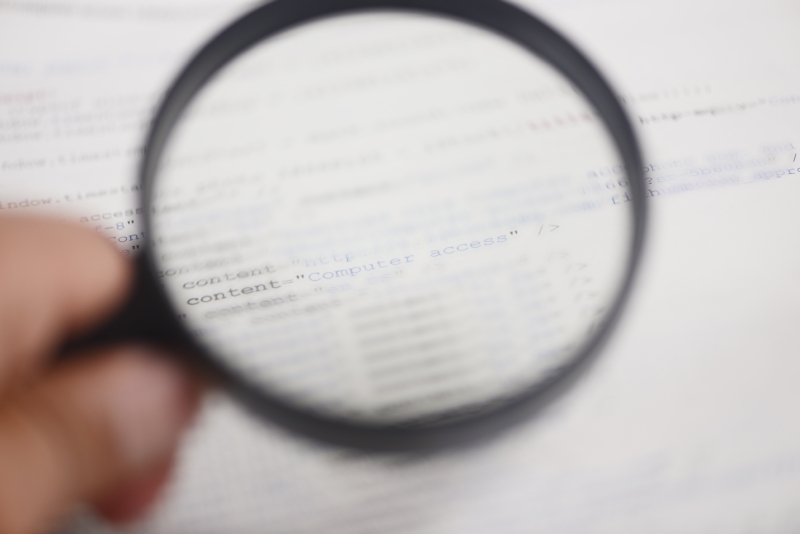
You might have lots of data on lots of customers, but imagine if you could suddenly add in a huge dollop of new, highly informative data that you weren’t able to access before. You could then use analytics to extract some really important insights about these customers, allowing you to
In my last post, we examined the growing importance of event stream processing to predictive and prescriptive analytics. In the example we discussed, we looked at how all the event streams from point-of-sale systems from multiple retail locations are absorbed at a centralized point for analysis. Yet the beneficiaries of those
(Otherwise known as Truncate – Load – Analyze – Repeat!) After you’ve prepared data for analysis and then analyzed it, how do you complete this process again? And again? And again? Most analytical applications are created to truncate the prior data, load new data for analysis, analyze it and repeat