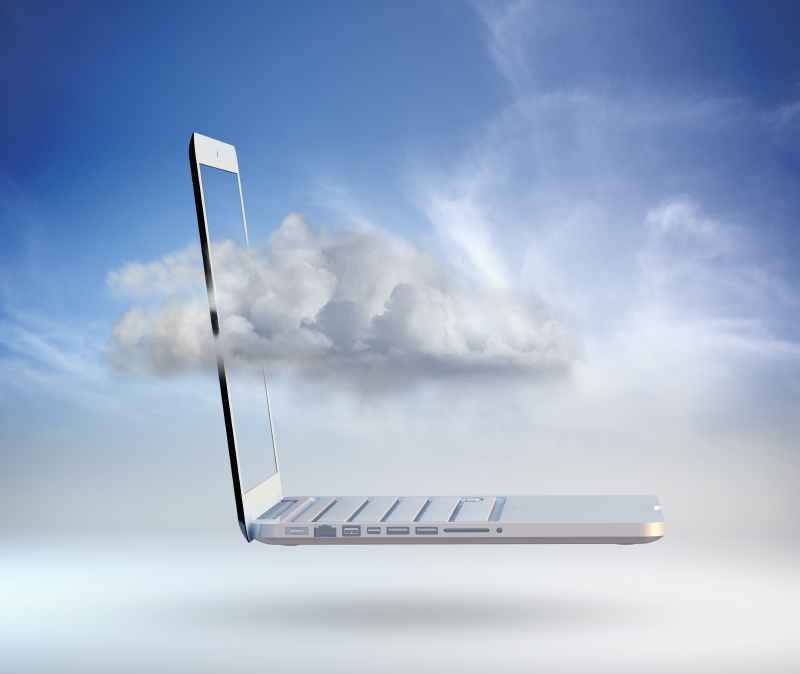
Na tym etapie nasza organizacja posiada określone dla programu Data Governance cele biznesowe oraz zarządza i współdzieli definicje pojęć biznesowych, którymi się posługuje. Ten logiczny obszar zarządzania danymi i informacją uzupełniony został o pomost do metadanych technicznych - w poprzednim kroku uzyskaliśmy jedno miejsce łączące informacje o technicznym przepływie danych w organizacji