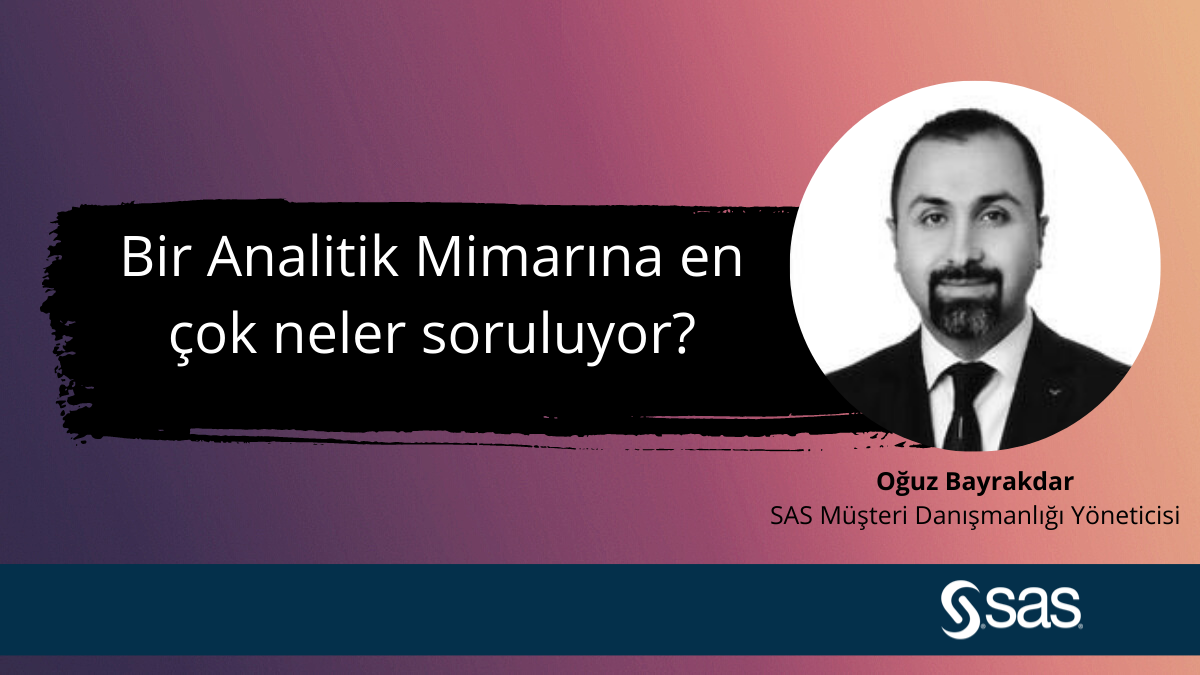
Teknik mimari çeşitli bir konudur- ancak bazı sorular tekrar tekrar gündeme gelir. Altyapıyı, açık kaynak politikasını, IoT veri yönetimini, paydaş katılımını kapsayan geniş yelpazenin tamamı, kurumsal mimarların oynadığı rolün giderek daha önemli bir hale geldiğine işaret ediyor. Bu yazımda son 12 ay boyunca en sık sorulan on soru ve müşterilerime