Blend, cleanse and prepare data for analytics, reporting or data modernization efforts
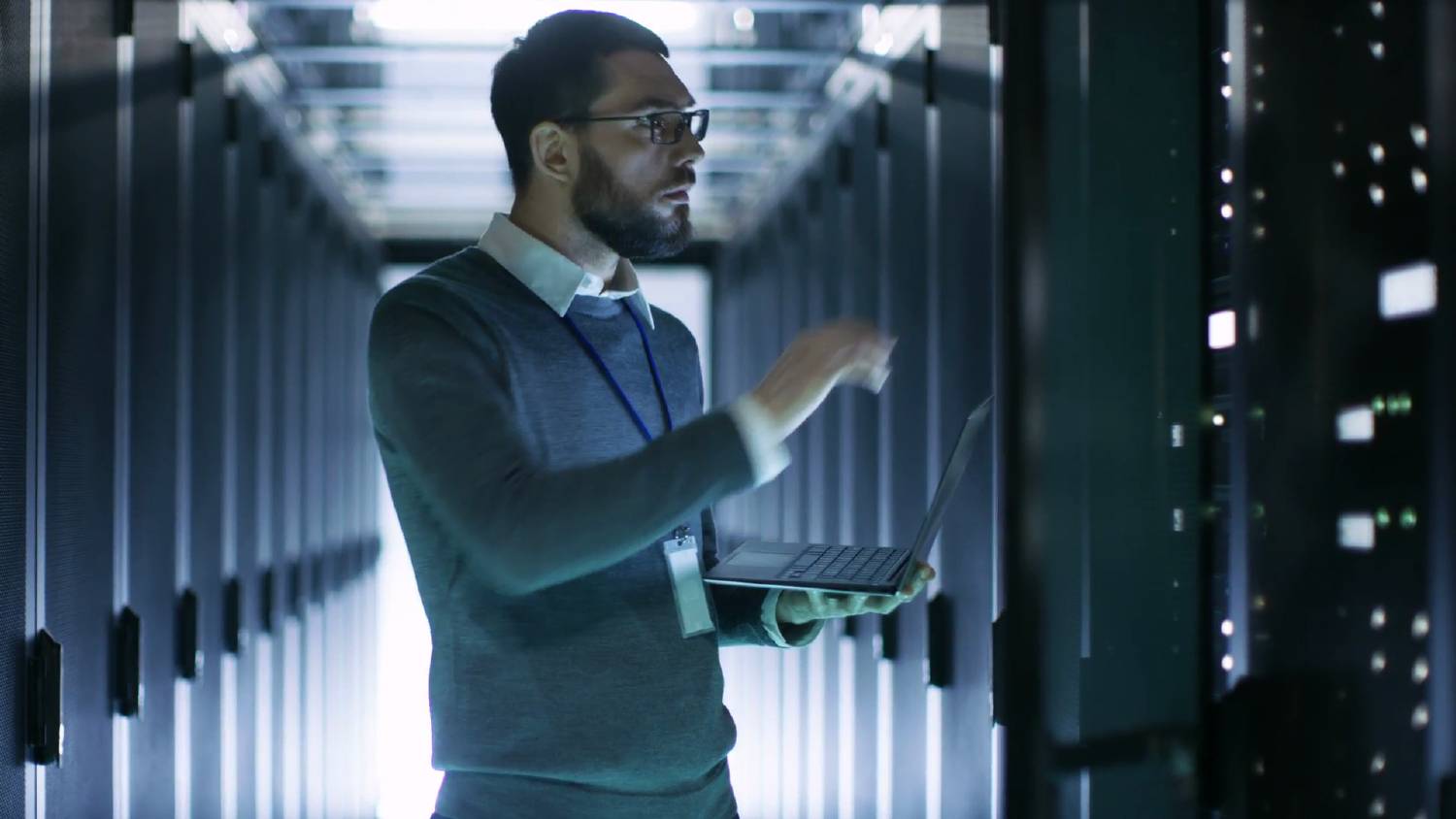
Guest blogger Khari Villela shares tips to help you skip common pitfalls of building a data lake.
Blend, cleanse and prepare data for analytics, reporting or data modernization efforts
Guest blogger Khari Villela shares tips to help you skip common pitfalls of building a data lake.
Las organizaciones se enfrentan a la creciente demanda de analítica de alto rendimiento que produzcan resultados rápidos y confiables. La transformación digital y el análisis empresarial son una prioridad en la agenda de muchas organizaciones. Para los CIO en sus organizaciones, significa una oportunidad para habilitar el valor a escala
Jim Harris shares some simple tips about how to minimize data usage.
Foi em Dezembro de 1991 que a relação do SAS e a Fidelidade começou, na altura entre o representante do SAS em Portugal e a Bonança, mais tarde integrada no que é hoje a Fidelidade, sendo que em 1995 e já com o SAS Portugal, foram assinados dois licenciamentos, um
David Loshin suggests that reference data could be the foundation of future governance structures.
Desde el primero de mayo, la GDPR (Reglamento General de Protección de Datos de la Unión Europea) pasó a ser de cumplimiento obligatorio. Eso significa que todas las organizaciones que manejan datos de ciudadanos europeos (sin importar dónde estén ubicadas) deben cumplir con los más altos estándares de transparencia y
Jim Harris shares three more examples of how data quality improves AI in Part 2 of his series.
この記事はSAS Institute Japanが翻訳および編集したもので、もともとはJames Ochiai-Brownによって執筆されました。元記事はこちらです(英語)。 自己完結型のパッケージ内でソフトウェアを実行するというアイディアは、2013年のDockerの立ち上げと共に広まり始め、今ではアプリケーション開発とDevOpsのコミュニティにおけるホットなトピックとなっています。Red Hat社による最近の調査では、調査対象企業の57%が、いくつかのワークロードにコンテナを利用しており、次の2年間で採用数が2倍近くになると期待している、と回答しています。 SASはこのトレンドを認識しており、現在ではデプロイメント・オプションの一つとしてSAS for Containersを提供しています。これが仮想マシン上でSASを実行する手法の完全なリプレースになるとは思われませんが、そこには顕著なメリットがいくつか存在します。 1. アナリティクスへのセルフサービス型アクセス 組織の中には、「SASを利用したいが、それを手にできない分析担当者」を抱えているところもあります。また、SAS Platformを保有しているものの、そのオンボーディング・プロセスに承認手続きが設けられているビジネス部門も存在します。プラットフォームの運用管理者がファイルシステムやセキュリティモデルに変更を加えなければならない可能性があり、そのプロセスに時間がかかることもあります。 コンテナを利用すると、物事がよりセルフサービス型になります。IT部門はSAS用の標準的なコンテナイメージを準備し、それを社内のユーザー向けに提供します。分析担当者は用途に応じてその中から選択し、自分専用のインスタンスを起動するだけで、数分以内にSASでの作業を開始できます。Domino Data LabとBlueData は、こうした機能を提供するコンテナベースのデータサイエンス・プラットフォームの例です。 2. 様々なソフトウェア・ツールやバージョンに関するニーズへの対応が簡素化 SAS Platformの従来型の実装は、多数のユーザーによって共用されます。ユーザーは設定済みのソフトウェアを使用しなければなりませんが、それが最新バージョンであるとは限りません。コンテナを利用すると、IT部門はデータ分析担当者に対し、SASとオープンソースのソフトウェアを組み合わせた幅広い種類のコンテナイメージを提供することができます。例えば、SAS 9.4、SAS Studio、Jupyter Notebookを組み合わせたコンテナイメージも可能ですし、SAS Studio、Jupyter Notebook、R Studioのいずれからでもアクセスできる形でSAS Viyaの機械学習機能を提供するようなイメージも可能です。IT部門は、試用版ソフトウェアを提供することさえ可能です。開発者は、特定のプロジェクトに必要なソフトウェア・コンポーネントやAPI群を組み合わせて、独自のコンテナイメージを作成することもできるようになります。 3. ソフトウェア・アップデートの容易化 実際には、コンテナ内のSASソフトウェアがアップデートされることはありません。必要なのは、新しいバージョンで別のコンテナイメージを作成し、それを用いて別のコンテナを構築することだけです。つまり、ソフトウェアのアッグレード中にユーザーの作業を邪魔することは一切ありません。週末の作業も不要ですし、アップグレードがうまく進まないときに、どうやってシステムを元に戻せばよいかパニックになることもありません。新しいコンテナをテストし、準備が整った段階でそれをユーザー向けに展開すればよいのです。様々なバージョンのコンテナイメージを保持できるため、ユーザーは時間的な余裕をもって自分のコードを各バージョンでテストしたり、問題がないことを確認した上で新しいバージョンに移行したりできるようになります。 4. スケーラブルかつ柔軟で、隔離された計算処理環境 コンテナ・オーケストレーター(例:Kubernetes)は、多くのコンテナを起動することで、大きなコンピューティング・リソースを割り当てることができます。そのため、オンボードするユーザーが増えても、ジョブがスローダウンすることはありません。リソース消費が特に激しいプロセスを実行する場合でも、それが他のユーザーに影響することはありません。各コンテナは、それぞれのマシンのリソースの範囲内でのみ実行可能です。したがって、より多くのパワーが必要な場合は、コンテナを停止し、より大きなマシン上でそれを起動し直します。作業の完了後にコンテナを終了すると、そのマシンは他のユーザーのために解放されます。 5. アナリティクスをWebアプリに統合することが可能 今や、アナリティクスは分析担当者だけのものでありません。デジタル変革に取り組んでいる組織は、顧客がデジタルチャネルを通じて利用するWebアプリやモバイルアプリの背後にアナリティクスを組み込もうとしています。具体的には、画像処理、レコメンデーション、意思決定支援などを含むAIアプリケーションなどが考えられます。これらのWebアプリは従来の方式で実装されたSAS Platformと組み合わせて機能させることも可能ですが、その一方で、必要なSASソフトウェア、分析モデル、小型の実行エンジンとしてのサポーティング・コードだけで構成した実行エンジンを軽量なコンテナに実装すると複数の利点があります。こうすることで、開発者は、他のユーザーに影響を与えることなく、SASソフトウェアの設定やAPI群を変更する自由を手にします。これは、アプリケーションがPythonまたはJavaで実装される方法に似ています。 6. 自動モデル・チューニング モデルの中には、データが変化するたびに、あるいは新しいフィードバックを受け取るたびに、頻繁に更新する必要があるものもあります。コンテナを利用すると、そうしたモデルを再チューニングし、その結果をコンテナ内にパッケージし、実業務環境にデプロイするまでのプロセスを自動化することができます。 7. DevOpsやCI/CDによるデプロイメントの合理化/効率化 典型的なSASユーザーはDevOpsの世界には馴染みがないかもしれませんが、DevOpsは昨今の主流となりつつあるアプリケーション開発手法です。アナリティクスをWebアプリに統合したい場合、私たちはこのプロセスに沿って進める必要があり、それを最も簡単に行う方法が、コンテナを利用する手法です。SASコードとモデルをコンテナ内にカプセル化すると、アプリ開発者(=Dev)側では、デプロイのために運用チーム(=Ops)側に渡す前に、コンテナに接続しテストを実行できるようになります。「継続的インテグレーション(CI)」と呼ばれる手法では、アプリ(SASのパーツを含むアプリ)の全てのブランチ(分岐)における変更は、それらが一緒に正しく機能する状態を確保するために、定常的にマージされ、自動テストにかけられます。「継続的デリバリー(CD)」と呼ばれる手法は、本番の業務環境へのリリースまでのプロセスを自動化します。これにより、アナリティクス・プリケーションの開発とデプロイを数週間ではなく、数日または数時間で完了することが可能になります。 8. ほぼ全ての場所にデプロイすることが可能 コンテナはポータブル性に優れているため、オンプレミスのデータセンターから、パブリッククラウドや、ドローン/トラック/列車に搭載されたエッジデバイスに至るまで、あらゆる種類の場所でSASの実行エンジンを動かすことが可能です。 コンテナは、イマジネーション豊かなアナリティクス活用を実現可能にする大きなポテンシャルをもたらします。あなたがSAS Viyaのライセンスをお持ちの場合は、SASが運営するDockerイメージ・ライブラリへのアクセス権を有していますから、そこから事前準備済みのコンテナイメージの形でSAS
David Loshin reminds us that data protection compliance applies to different individuals in different contexts – and not just GDPR and CCPA.
Phil Simon weighs in on using data to make the most of AI.
Phil Simon says that the downsides of even a few discrepancies can be enormous.
Además de mejorar la eficiencia de las organizaciones, con el aprovechamiento del Big Data, las instituciones pueden gestionar información que los ayude a responder a las necesidades de los ciudadanos. Su uso en el sector del gobierno, por ejemplo, contribuye a mejorar la comprensión de los fenómenos sociales y apoyar
What's the impact of using data governance and analytics for the business side of education? It's an interesting question, and during a video interview, Dale Pietrzak, Ed.D.,Former Director of Institutional Effectiveness and Accreditation (IEA) at the University of Idaho shared details on the results they're realizing from using SAS for
Jim Harris shares examples of how and why AI applications are dependent on high-quality data.
The idea of running software in a self-contained package took off with the launch of Docker in 2013 and has become a hot topic in the application development and DevOps community. In a recent survey by Red Hat, 57 percent of companies questioned said they use containers for some workloads
Data scientists spend a lot of their time using data. Data quality is essential for applying machine learning models to solve business questions and training AI models. However, analytics and data science do not just make demands on data quality. They can also contribute a lot to improving the quality
The broad CCPA definition of personal information could open a can of worms for compliance and governance.
Además de mejorar la eficiencia de las organizaciones, con el aprovechamiento del Big Data, las instituciones pueden gestionar información que los ayude a responder a las necesidades de los ciudadanos. Su uso en el sector del gobierno, por ejemplo, contribuye a mejorar la comprensión de los fenómenos sociales y apoyar
SAS Decision Manager enables you to build and test decisions to use in batch processes, real-time web applications or with SAS Event Stream Processing. In this blog, I explain how to use Rulesets in an Event Stream Process project. If you are streaming data using SAS Event Stream Processing and
I am obsessed with jigsaw puzzles. Specifically, 1000-piece mystery puzzles, entertaining not just for their pictorial humor, but also for the challenge. Unlike traditional puzzles, you don't know what you are putting together because the completed puzzle isn't pictured on the box. Mystery puzzles are constructed so that you must
Jim Harris says curating AI’s curriculum is the responsibility of data stewards.
Learn why Jason Simon from UNT calls data governance critical.
There's a chasm in today's business world between "can" and "should." Let's hope that gap closes soon.
Learn about the role data classification plays when governing a diversity of data policies.
불과 몇 년 전 까지만 해도 ‘데이터 사이언티스트’라는 단어는 많은 사람들에게 꽤나 생소한 단어였을 것입니다. 하지만 4차 산업혁명이 이미 산업 전반에 깊숙이 자리한 오늘날, 빅데이터 분석이 핵심 역량으로 부상하며 데이터 과학자(data scientist)의 인지도 역시 굉장히 높아졌습니다. 단순히 인지도뿐만이 아닙니다. 세계 최대 취업 정보 사이트 글래스도어(Glassdoor)가 발표한 2019년 ‘미국 최고 직업
Expect to lose time if you don't include a data steward in your project until you're reviewing the data model.
Recently, I worked on a cybersecurity project that entailed processing a staggering number of raw text files about web traffic. Millions of rows had to be read and parsed to extract variable values. The problem was complicated by the varying records composition. Each external raw file was a collection of
Todd Wright says questions from the C-suite morph as the complex data and analytics landscape evolves.
Brush up on the latest news around data privacy. Todd Wright shares his top 10 picks.
Jim Harris discusses a key role of the data engineer – protecting sensitive personal data.