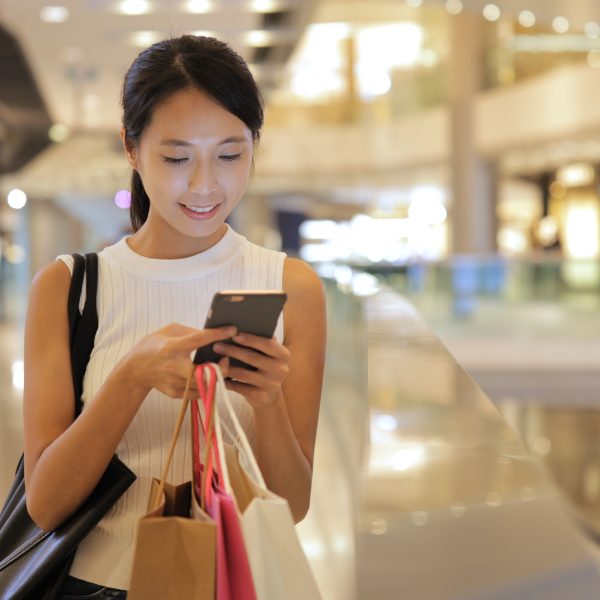
Retailers face unprecedented challenges with supply chain volatility, inflation, oil price fluctuations, labor shortages and geopolitical activities, making it difficult to plan across the organization. With retail evolving, coupled with persistent supply chain issues, this adds complexity to anticipating and planning for shifts in consumer demand. The emergence of an