The DO Loop
Statistical programming in SAS with an emphasis on SAS/IML programs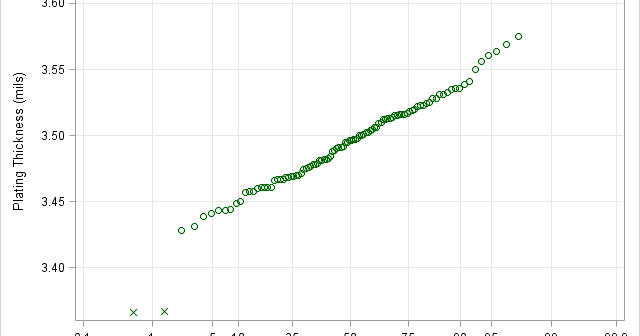
Many data analysts use a quantile-quantile plot (Q-Q plot) to graphically assess whether data can be modeled by a probability distribution such as the normal, lognormal, or gamma distribution. You can use the QQPLOT statement in PROC UNIVARIATE to create a Q-Q plot for about a dozen common distributions. However,
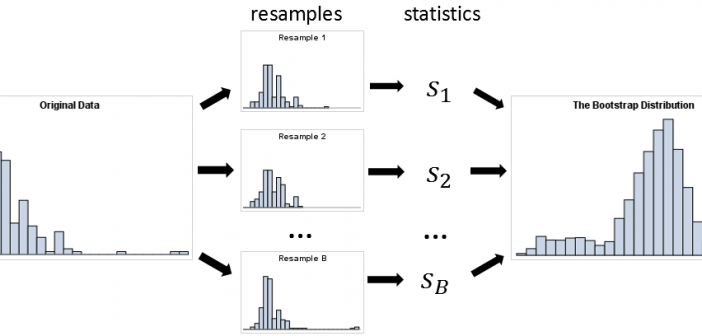
This article describes best practices and techniques that every data analyst should know before bootstrapping in SAS. The bootstrap method is a powerful statistical technique, but it can be a challenge to implement it efficiently. An inefficient bootstrap program can take hours to run, whereas a well-written program can give
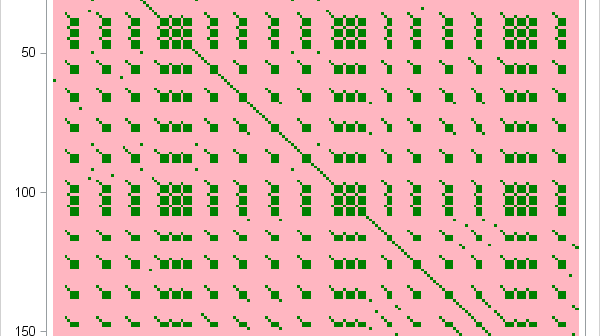
The best way to spread Christmas cheer is singing loud for all to hear! -Buddy in Elf In the Christmas movie Elf (2003), Jovie (played by Zooey Deschanel) must "spread Christmas cheer" to help Santa. She chooses to sing "Santa Claus is coming to town," and soon all of New
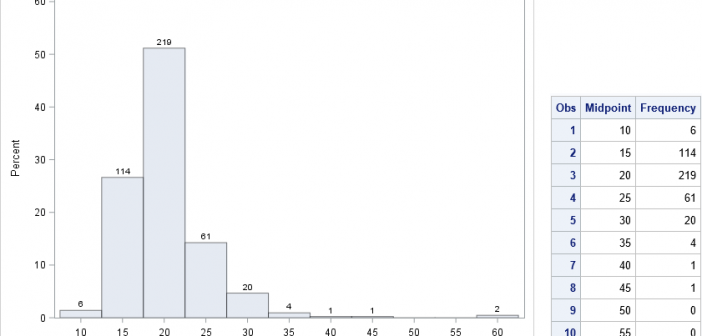
Recently a SAS programmer wanted to obtain a table of counts that was based on a histogram. I showed him how you can use the OUTHIST= option on the HISTOGRAM statement in PROC UNIVARIATE to obtain that information. For example, the following call to PROC UNIVARIATE creates a histogram for
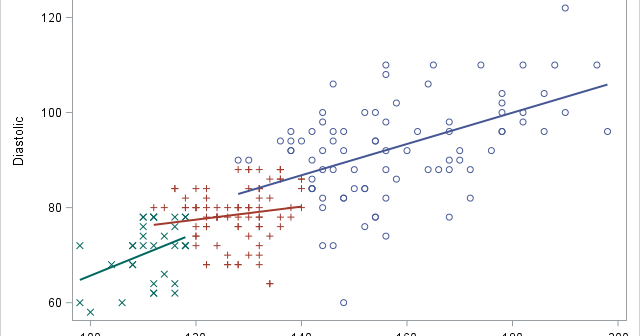
When a graph includes several markers or line styles, it is often useful to create a legend that explains the relationship between the data and the symbols, color, and line styles in the graph. The SGPLOT procedure does a good job of automatically creating and placing a legend for most
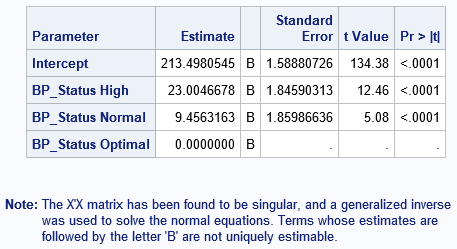
I remember the first time I used PROC GLM in SAS to include a classification effect in a regression model. I thought I had done something wrong because the parameter estimates table was followed by a scary-looking note: Note: The X'X matrix has been found to be singular, and a