Government Data Connection
Using data to serve citizens, save money & improve quality of life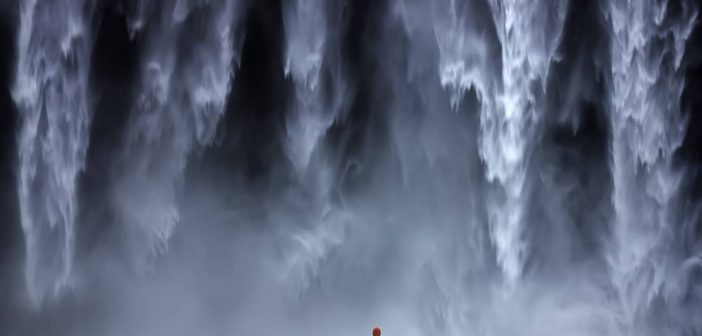
Government employees charged with monitoring environmental compliance face a downpour of information, wading through countless reports and stacks of paperwork to accomplish their mission. To help these dedicated public servants increase productivity, agencies should consider a broader set of tools to control pollution, enforce regulations and improve compliance. Although foundational
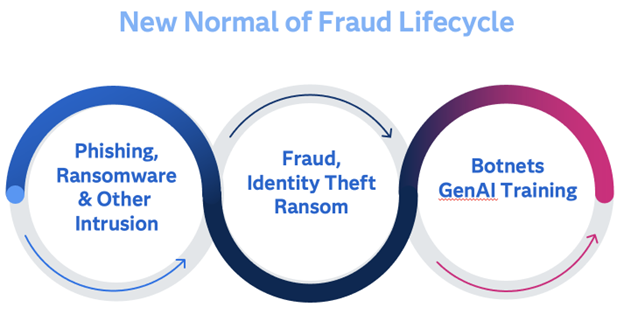
When we think of something “new”, we tend to picture something clean, shiny, or efficient. The new normal of fraud only checks one of those boxes, and unfortunately, that’s “efficient.” Today, scams and cyber attacks are persistent, and part of a larger ecosystem of phishing and hacking attacks by both
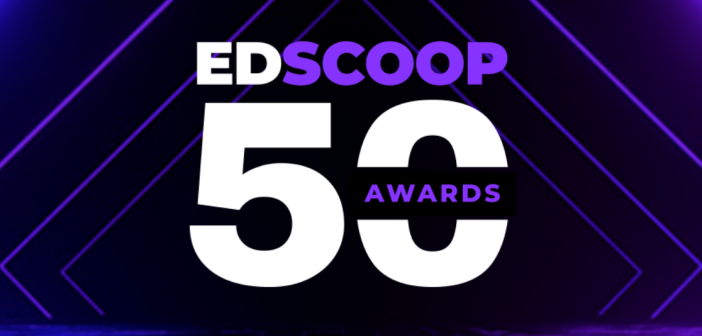
Higher Education has been slow to adopt analytics in comparison to the commercial sector, but those institutions that have embraced a culture of analytics have seen significant and tangible results. Higher Education analytics can help in nearly every corner of academia including enrollment and retention, student success, academic research and
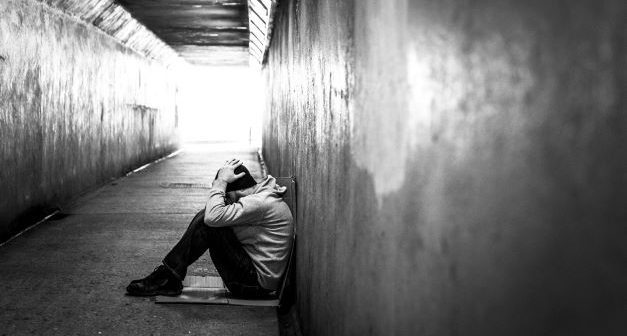
September honors Recovery Month, emphasizing hope for recovery in behavioral health, especially from substance use disorders (SUD). A key motto of Recovery Month is that Recovery Happens, helping people know that even at rock bottom, things can improve. We all need that hope at various points in our lives. Often,
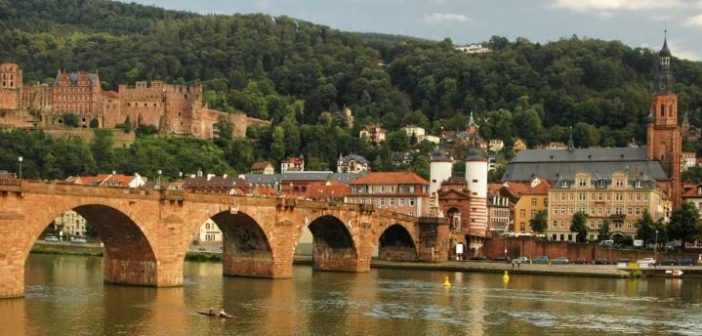
The city of Heidelberg, Germany is known for its romantic cityscape, which attracts tourists from all over the world. It also has the youngest population in Germany, thanks largely to the many students at one of Europe's oldest and largest universities. Perhaps less well known is that Heidelberg is twinned
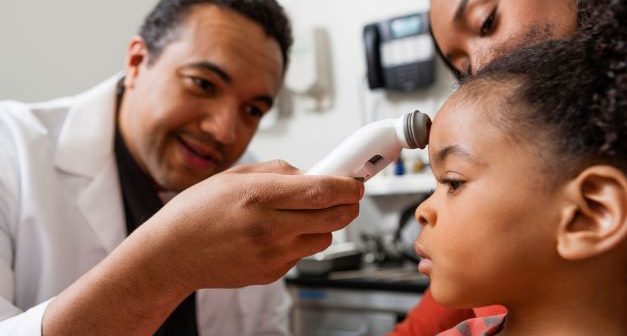
When Los Angeles County invested in Whole Person Care (WPC) it could not have known just how important the system’s flexibility would be. Anyone who has had an interface with health care delivery, policy, oversight and management know things change quickly. As data becomes a priority, expectations of the use