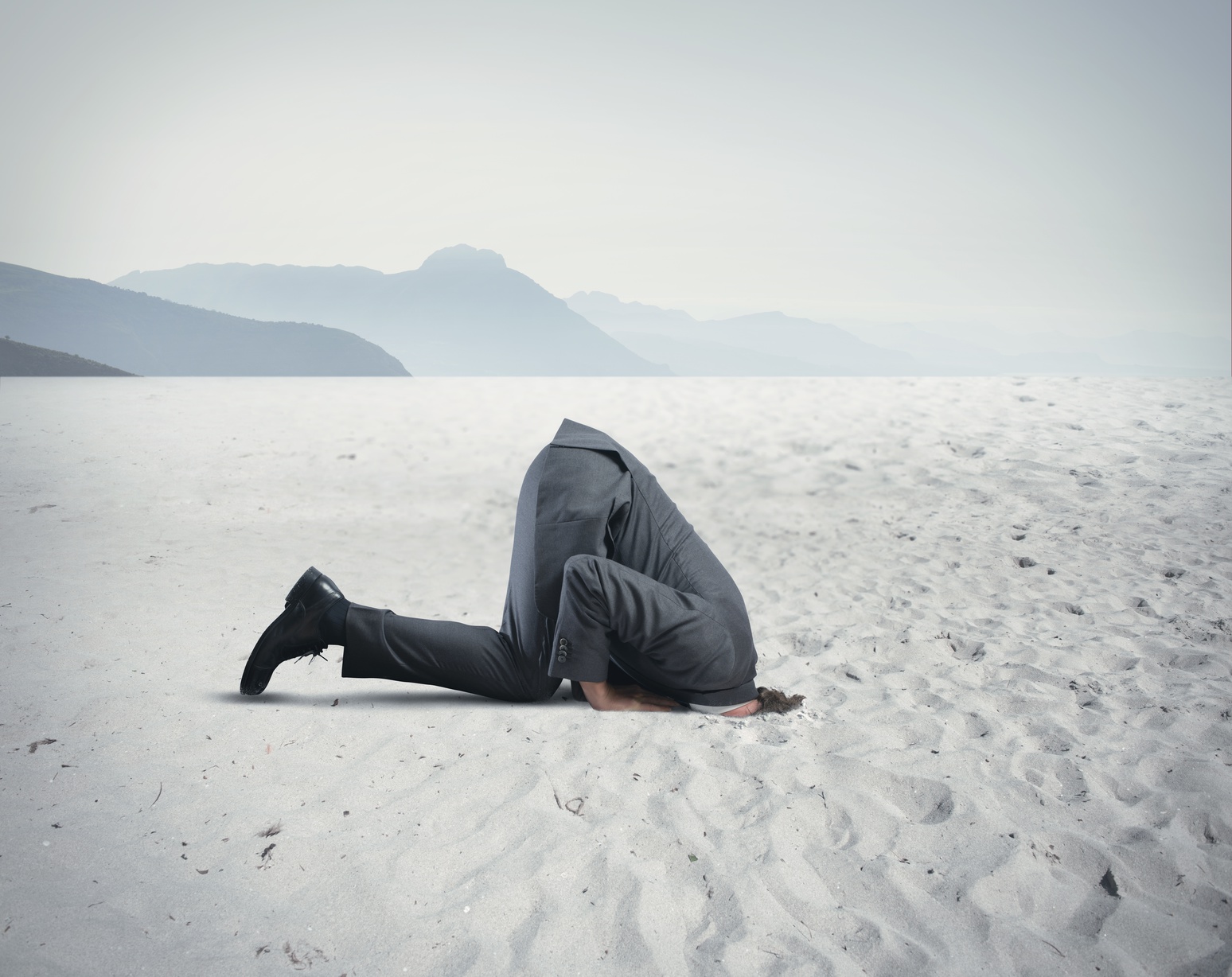
Datenaufbereitung, Datenintegration, Datenqualität, Datensicherheit – all das hört sich nach Pflichtprogramm für die IT an und ist längst nicht so sexy wie Hype-Themen à la Data Science, Internet of Things oder Artificial Intelligence. Dass Datenmanagement im Businesskontext aber einen mindestens ebenso großen Stellenwert hat – sei es für die Optimierung