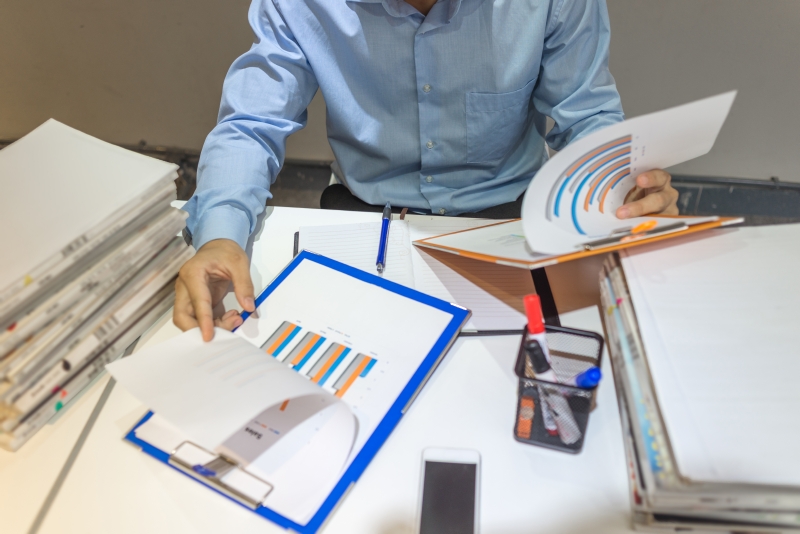
The rise of self-service analytics, and the idea of the ‘citizen data scientist’, has also brought a number of issues to the fore in organizations. In particular, two common areas of discussion are the twin pillars of data quality and data preparation. There is no doubt that good quality, well-prepared